Understanding Genomic Data: Key Insights and Implications
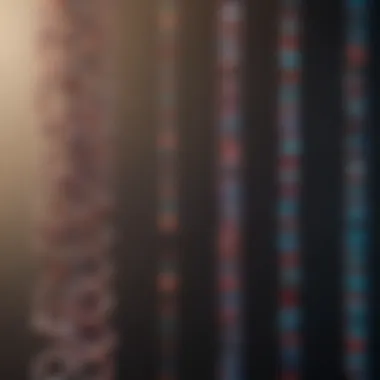
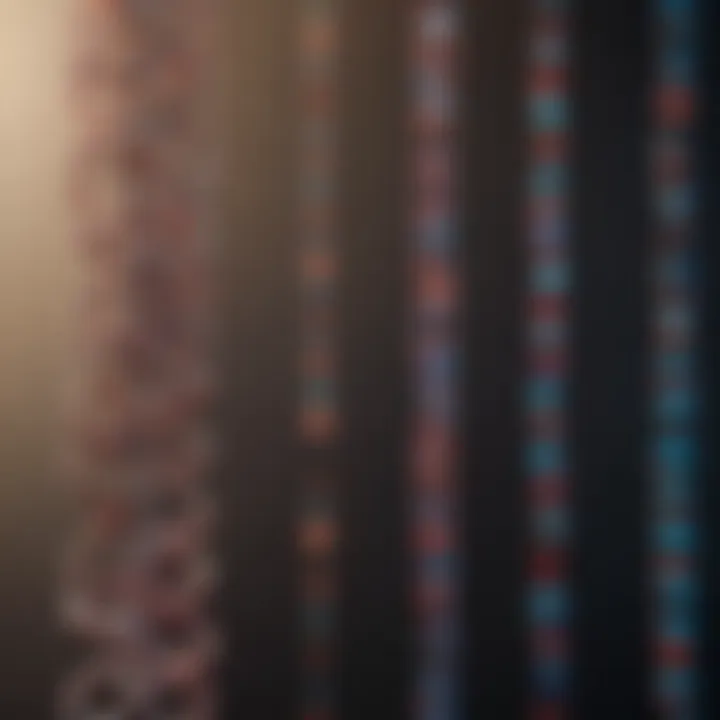
Intro
Genomic data has emerged as a pivotal element in the landscape of biological research and medical practice. This multifaceted field encompasses a wealth of information, including DNA sequences, RNA expressions, and inherent variations among organisms. Understanding genomic data is not merely an academic pursuit; it has profound implications in health, disease prevention, and personalized medicine. This article endeavors to provide a detailed exploration of genomic data, examining its significance, methodologies employed in research, and the challenges it presents.
Research Overview
Summary of Key Findings
The investigation into genomic data reveals several critical insights. Firstly, advancements in sequencing technologies have made it possible to decode genomic information at an unprecedented scale and speed. The implications for health are vast, allowing for more accurate diagnoses, targeted therapies, and even the potential for preventive measures based on an individual’s genetic profile. Understanding the genetic factors contributing to diseases has opened new avenues for research in areas like cancer genomics and rare genetic disorders.
Research Objectives and Hypotheses
This article aims to clarify how genomic data is utilized across various fields. The primary objectives include:
- Examining the integration of genomic data in clinical practice.
- Analyzing the methodologies that researchers use to collect and interpret this data.
- Understanding the ethical considerations associated with genomic research, particularly concerning privacy and consent.
The hypotheses guiding this exploration includes the premise that genomic data significantly contributes to advancements in healthcare outcomes and informs public health strategies.
Methodology
Study Design and Approach
The approach taken in this exploration combines both qualitative and quantitative analysis of existing literature. By reviewing peer-reviewed articles, case studies, and reports from reputable sources, a comprehensive view of how genomic data integrates into different fields emerges. This method allows for a balanced perspective, addressing the nuances and complexities inherent in genomic research.
Data Collection Techniques
Collecting genomic data often involves several sophisticated techniques. These include, but are not limited to:
- Whole Genome Sequencing (WGS): This technique reveals the entirety of an organism's genetic material.
- Gene Expression Profiling: Used to study the activity levels of various genes at once, providing insights into cellular responses.
- Single Nucleotide Polymorphism (SNP) Analysis: This identifies variations at the single nucleotide level, crucial for understanding genetic diversity and predisposition to diseases.
Genomic data can be intricate and requires careful consideration when interpreting results to draw meaningful conclusions.
"The integration of genomic data into healthcare promises not only to enhance diagnostics but also to tailor treatment strategies to individual patients."
By focusing on these methodological aspects, the article will highlight the capabilities and limitations that genomic data presents in both research and clinical settings.
Preamble to Genomic Data
Genomic data represents a core facet of modern biological research, playing a pivotal role in various fields such as medicine, agriculture, and evolutionary biology. Understanding this data is essential for comprehending the biological mechanisms that underlie health and diseases. As we move deeper into the realm of genomics, it is crucial to appreciate how genomic data influences scientific inquiry and practical applications. This section introduces key concepts and sets the stage for a detailed exploration of genomic data's significance.
Definition of Genomic Data
Genomic data refers to the comprehensive information encoded within the genetic material of organisms. This includes both DNA and RNA, which carry the instructions necessary for life processes. The data encompasses sequences of nucleotides, variations in those sequences, structural arrangements, and overall genomic architecture. Essentially, genomic data is a blueprint that helps explain biological traits, disease phenotypes, and evolutionary changes.
Importance of Genomic Data in Research
The value of genomic data in research cannot be overstated. Here are several key reasons emphasizing its importance:
- Disease Understanding: Genomic data aids in identifying genetic factors associated with diseases. This information is critical for developing targeted therapies and enhancing prevention strategies.
- Personalized Medicine: With genomic profiling, healthcare can move towards individualized treatments based on a patient’s unique genetic makeup. This shift improves treatment efficacy and reduces adverse effects.
- Biological Insights: Researchers can examine the genomic variations that contribute to traits in species, broadening our understanding of fundamental biology.
- Agricultural Improvement: Genomic data supports the development of genetically modified organisms that can withstand environmental stresses or provide higher yields. This is increasingly important in the context of global food security.
"The future of genomics is not just about understanding the genetic code; it's about applying that knowledge to improve health outcomes and quality of life."
In summary, genomic data is indispensable in driving advancements across various fields. The following sections will delve into types of genomic data, methods for data collection, and its applications. Each aspect will highlight how genomic data shapes our understanding of life itself.
Types of Genomic Data
Understanding the types of genomic data is pivotal for comprehending how genetic information is analyzed and utilized in various research areas. Each category of genomic data provides unique insights into biological processes, disease mechanisms, and potential therapeutic targets. Knowing these types enhances research effectiveness and informs decisions in clinical settings, facilitating advancements in healthcare and scientific research.
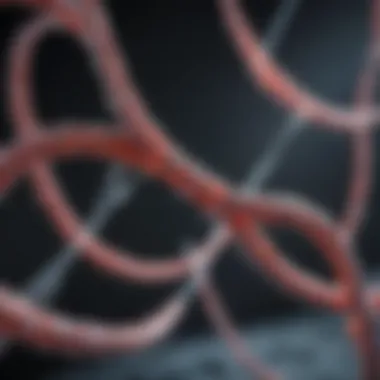
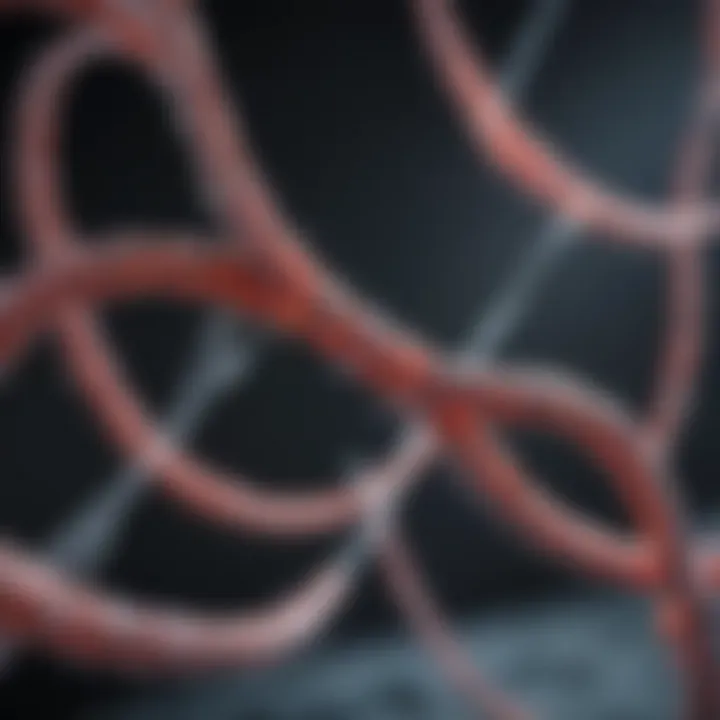
DNA Sequence Data
DNA sequence data is fundamental in genomics. It refers to the precise sequence of nucleotides within a DNA molecule. This data helps researchers identify the genetic variations that can influence traits and disease susceptibility. For instance, variations in the BRCA1 gene relate to breast cancer risk.
The analysis of DNA sequence data primarily relies on sequencing technologies like Next-Generation Sequencing (NGS). NGS has revolutionized genomic studies by enabling the analysis of entire genomes quickly and cost-effectively. Important techniques involved include whole-genome sequencing, targeted sequencing, and exome sequencing. Each of these techniques have distinct applications, from basic research to clinical diagnostics.
Some significant benefits and considerations include:
- Identification of Genetic Variants: Helps in linking specific genes to diseases.
- Evolutionary Studies: Provides insights into evolutionary biology and relationships between species.
- Clinical Applications: Enables the development of tailored treatments based on individual genetic make-up.
RNA Sequence Data
RNA sequence data pertains to the sequences of ribonucleic acid (RNA) molecules, which play crucial roles in gene expression and regulation. This data helps researchers understand how genes are expressed in different cells and under various conditions. It can reveal how environmental factors influence gene activity, which is essential for studies on development and disease.
The primary method for obtaining RNA sequence data is RNA-Seq. This technique allows for the quantification of RNA, enabling the determination of gene expression levels. Researchers can analyze the transcriptome comprehensively, identifying differentially expressed genes in conditions like cancer versus healthy tissues.
Key considerations with RNA sequence data include:
- Dynamic Expression: RNA levels can change rapidly, reflecting biological responses.
- Alternative Splicing: Understanding the variations of RNA can lead to insight into protein diversity.
- Non-coding RNAs: Many RNA molecules do not code for proteins but play roles in regulation, which are crucial for a full understanding of cellular function.
Epigenomic Data
Epigenomic data focuses on the chemical modifications to DNA and histone proteins that regulate gene expression without altering the underlying DNA sequence. Epigenetics plays a critical role in development, cellular differentiation, and responses to environmental factors. Changes to the epigenome can lead to significant implications in health and disease, such as cancer and neurological disorders.
Methods for studying the epigenome include ChIP-Seq for histone modifications and bisulfite sequencing for DNA methylation analysis. These techniques help reveal the layers of regulation that control which genes are active and when.
Considerations when working with epigenomic data include:
- Reversibility: Epigenetic changes can be reversible, making them potential targets for therapeutic interventions.
- Environmental Influences: Factors such as diet, stress, and exposure to toxins can modify epigenetic marks.
- Complex Interactions: Epigenomic data often requires integration with other omics data, presenting challenges in analysis and interpretation.
Understanding the various types of genomic data is essential. Each type provides unique insights that enhance our understanding of genetics, disease, and potential therapies.
By dissecting DNA sequence data, RNA sequence data, and epigenomic data, researchers can forge pathways toward innovative treatments and deepen our grasp of genetic science. The implications of their findings influence not only scientific understanding but also practical applications in clinical settings, further showcasing the importance of comprehensive genomic exploration.
Methods for Collecting Genomic Data
Collecting genomic data is a vital aspect in the field of genetics. These methods provide the necessary foundation for understanding the genetic blueprint of organisms. The accuracy and effectiveness of genomic studies depend heavily on the techniques employed for data collection. Each method offers specific advantages and limitations.
Next-Generation Sequencing
Next-Generation Sequencing (NGS) represents a major leap in genomic data collection. This technology allows for the rapid sequencing of large stretches of DNA or RNA. One of the significant benefits of NGS is its ability to generate millions of sequences in a single run. This capability is not only time-efficient but also cost-effective compared to traditional sequencing methods.
NGS is particularly useful in various research fields. For instance, it enables whole-genome sequencing, exome sequencing, and targeted sequencing. The data obtained can be utilized in medical research, population genetics, and evolutionary biology. Despite its advantages, NGS does come with considerations, such as the need for extensive data analysis and the potential for errors in sequencing.
Microarray Technology
Microarray technology is another essential tool for collecting genomic data. This method allows researchers to analyze the expression levels of thousands of genes simultaneously. By using a small glass slide or a silicon chip, microarrays can detect a wide variety of genetic information. They are particularly valuable in understanding gene expression patterns in different conditions, such as diseases or treatments.
One of the main advantages of microarrays is their ability to quickly obtain information about gene expression across many samples. However, microarrays require prior knowledge of gene sequences, which can limit their use in certain contexts. Researchers need to ensure they are meticulously designed to avoid cross-hybridization, which can lead to misleading results.
PCR and Sanger Sequencing
The Polymerase Chain Reaction (PCR) and Sanger Sequencing are foundational techniques in genomics. PCR is a method used to amplify a specific DNA segment, making it easier to study. This method is widely used due to its simplicity and efficiency. It allows researchers to produce millions of copies of a particular DNA sequence from a small initial sample.
Sanger Sequencing, in contrast, is a more traditional method used for determining the sequence of DNA. It is often employed to confirm sequences obtained from NGS or to sequence smaller sections of DNA. Although it is more labor-intensive and costly than NGS, it provides high accuracy and reliability. Both methods play a critical role in clinical diagnostics and research, providing vital insights into genetic variations.
Important Note: The choice of method for collecting genomic data significantly affects data quality and research insight.
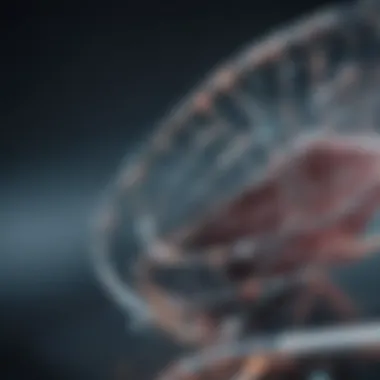
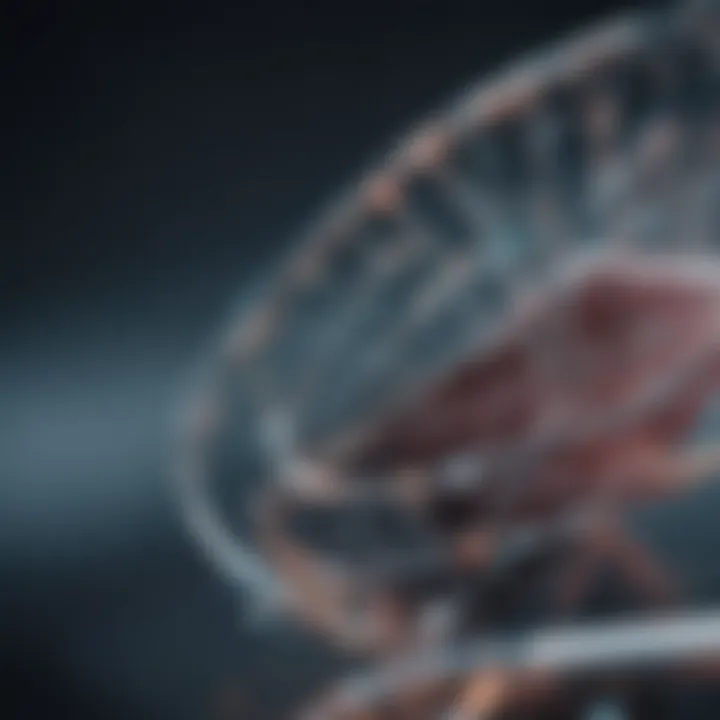
In summary, understanding these methods for collecting genomic data is essential for students, researchers, educators, and professionals in genetics. Each method comes with its own strengths and weaknesses. By selecting the appropriate technique, researchers can enhance the reliability and depth of their genomic studies.
Applications of Genomic Data
The field of genomic data carries significant implications across various sectors. Its applications extend from healthcare to agriculture, fundamentally altering how we approach disease management, treatment options, and food production. Understanding these applications is essential for appreciating the transformative potential of genomic understanding.
Clinical Diagnostics
Clinical diagnostics benefit immensely from genomic data. This information allows for the identification of genetic disorders at earlier stages. For instance, sequencing an individual's genome can reveal mutations linked to specific diseases. Accurate diagnosis leads to timely interventions, improving overall patient outcomes. Moreover, genomic data aids in stratifying patients based on their genetic predisposition to diseases.
"Genomic data empowers clinicians to make informed decisions about patient care, enhancing diagnosis and treatment efficacy."
As a result, tests like whole-genome sequencing or targeted therapies based on a patient’s genetic profile can be implemented. This level of specificity is invaluable for conditions such as cancer, where targeted therapies are often more effective than standard treatments.
Personalized Medicine
Another pivotal application of genomic data is in personalized medicine. This approach tailors healthcare to the unique genetic profile of each individual, recognizing that one-size-fits-all solutions may not suffice. With genomic insights, healthcare providers can select the most effective medication for a patient based on their genetic makeup. For example, the response to drugs like warfarin or clopidogrel can vary drastically among individuals due to genetic differences.
The incorporation of genomic data into drug development also streamlines the discovery process. Researchers can identify biomarkers to target specific genetic variations, which significantly enhances the success rate of clinical trials. Personalized medicine promises a future where treatments are not only more effective but also safer for the patient.
Agricultural Biotechnology
In the domain of agricultural biotechnology, genomic data plays a crucial role. It enables the development of genetically modified organisms (GMOs), which are designed to exhibit desirable traits such as enhanced resistance to diseases or improved nutritional content. This technology addresses global challenges like food security, as it allows for higher yields and resilience against environmental stressors.
Additionally, genomic tools facilitate the breeding of crops that are more sustainable and environmentally friendly. By analyzing the genetic traits of plants, scientists can accelerate breeding programs that incorporate desirable characteristics more quickly than traditional methods. Thus, genomic data not only supports agricultural advancement but also contributes to sustainable farming practices.
Overall, the applications of genomic data are vast and impactful. It transforms diagnostics, personalizes medical treatments, and enhances agricultural productivity. As the integration of genomic data in these fields continues to evolve, it holds immense promise for improving health outcomes and addressing challenges in food production.
Challenges in Genomic Data Usage
The analysis and application of genomic data present various challenges which must be carefully navigated to fully harness its potential. First and foremost, these challenges can hinder the effective use of genomic information across diverse fields such as healthcare, agriculture, and research. Understanding these challenges is essential for stakeholders, including scientists, policymakers, and patients. This section will explore three primary challenges: data privacy and security, interpretation of complex data, and integration across disciplines.
Data Privacy and Security
Data privacy and security is a significant concern in genomic research. As genomic data contains a wealth of personal information, its misuse can lead to privacy violations. This issue raises questions regarding who owns the data and how it should be managed. Individuals may provide their genomic information for research purposes, but they often expect that their privacy will be safeguarded.
The adoption of comprehensive protocols and legislation, such as the General Data Protection Regulation (GDPR) in Europe, has become critical. These regulations aim to protect the privacy of personal data, including genomic data, while facilitating research. Researchers must implement robust encryption methods and secure data storage practices to minimize the risk of data breaches. Collaborations across institutions, focused on developing best practices, are essential to ensure compliance.
"Privacy concerns must be at the forefront of genomic data usage to maintain public trust in research."
Interpretation of Complex Data
Another challenge facing researchers is the interpretation of complex genomic data. Genomic studies often produce vast amounts of data that can be overwhelming to analyze. Advanced computational tools and robust statistical methodologies are necessary to derive meaningful insights from this information. However, understanding the relationships between genetic variants and phenotypic outcomes remains a daunting task.
Bioinformatics plays a crucial role in deciphering this data. Tools such as genomic variant annotation and gene expression analysis help researchers navigate complexities. However, there remains a need for further advancements in these fields, as the sheer volume of data can lead to errors and misinterpretations. Continuous education and training for researchers will help mitigate these issues and support more reliable analyses.
Integration Across Disciplines
The integration of genomic data with other scientific disciplines presents its own set of challenges. Genomics does not exist in isolation; it overlaps with fields like proteomics, metabolomics, and clinical practices. Achieving collaborative efforts among these disciplines can be hindered by differences in methodologies, terminologies, and data formats.
Fostering interdisciplinary collaboration is essential to overcome these barriers. Platforms that facilitate shared resources and data exchange can help researchers from various fields work together effectively. This integration can lead to more holistic understandings of biological systems, ultimately advancing our knowledge in areas such as disease prevention and treatment.
Ethical Considerations in Genomic Data
The rise of genomic data generation has brought forth an array of ethical questions and considerations. As researchers and clinicians gather vast amounts of genetic information, it becomes necessary to interrogate the implications of this practice. Ethical considerations not only ensure the responsible use of genomic data but also protect the rights and identities of individuals involved. In this section, we will address key elements pertaining to consent, ownership, and the technological implications that genomic advancements introduce.
Consent and Ownership
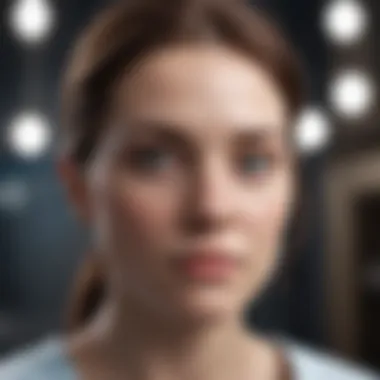
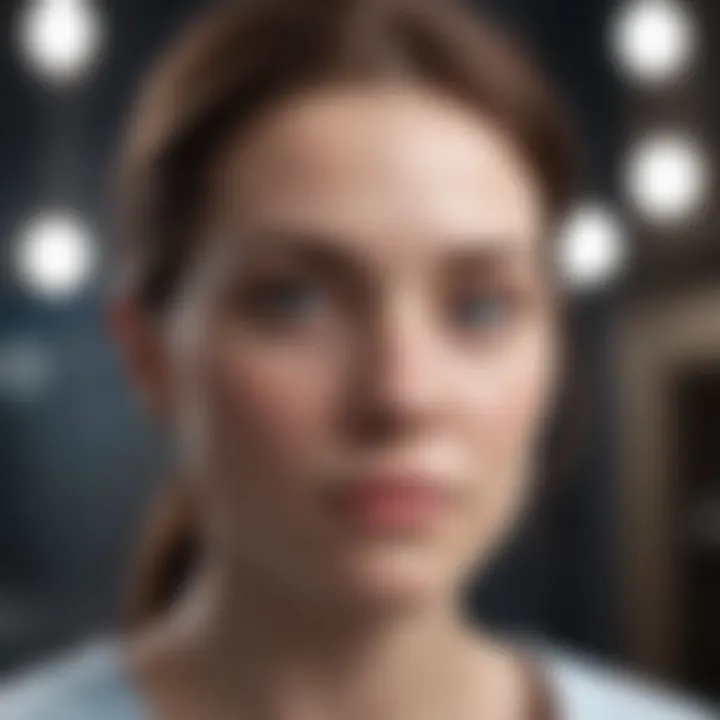
Consent is a cornerstone in the ethical landscape of genomic data research. It signifies an individual's agreement to participate in research and share personal genetic information. Informed consent should be more than just a formality; it must encompass a comprehensive understanding of what is being asked of participants.
- Key Aspects of Consent:
- Transparency: Participants should be fully aware of how their data will be used, stored, and shared. This transparency fosters trust between researchers and participants.
- Voluntariness: Consent must be given freely, without coercion. Participants should have the autonomy to decline or withdraw at any moment without facing repercussions.
Ownership of genomic data introduces further ethical challenges. Questions arise about who has the rights to data produced from a person's genetic material—should it belong to the individual, the researcher, or the institution? This debate includes:
- The need for policies addressing data ownership rights.
- Potential benefit-sharing models, ensuring that individuals whose data has been used for research might gain from any resulting advancements.
"As genomic data becomes more integrated into healthcare, understanding consent and ownership is crucial for ethical compliance."
Implications of CRISPR Technology
The advent of CRISPR technology has transformed the field of genetics, offering unprecedented opportunities for editing genetic material. However, this also raises ethical dilemmas. Although CRISPR has the potential to eliminate genetic diseases, it could also lead to unintended consequences such as off-target mutations or ethical implications regarding designer genes.
Several considerations emerge regarding CRISPR interventions:
- Health Equity: Access to CRISPR treatments may not be equally available across different demographic groups, raising concerns about fairness and access in health care.
- Gene Editing in Embryos: Editing embryos invokes sensitive questions about our responsibilities toward future generations and the potential for unintended hereditary effects.
- Regulation: Establishing guidelines to govern CRISPR research and applications is essential to ensure ethical practices and alignment with societal values.
Future Directions in Genomic Data Research
The landscape of genomic data research is evolving rapidly due to technological advancements and interdisciplinary collaborations. Understanding the future directions of this field is essential as it holds the potential to transform various aspects of healthcare, agriculture, and environmental science. This section will delve into the significance of future directions in genomic data research and how they can reshape our approach to understanding biological systems.
Advancements in Computational Biology
Computational biology is at the forefront of genomic data analysis. With the ever-increasing volume of genomic data produced by sequencing technologies, computational methods are critical for interpreting this data efficiently.
Recent advancements include:
- Machine Learning and AI: Machine learning algorithms provide tools to identify patterns in large-scale genomic datasets. Applications include predicting disease susceptibility and treatment responses.
- Data Integration: New computational frameworks are being developed to integrate data from diverse sources, such as genomics, proteomics, and metabolomics. This holistic approach enhances our understanding of biological processes.
- Cloud Computing: The rise of cloud platforms allows researchers to store and process large genomic datasets without the need for extensive local infrastructure. This facilitates collaboration across global research teams.
These advancements are crucial since they make analysis faster, more efficient and allow for novel insights that were previously unattainable.
Integration of Genomic and Phenomic Data
Phenomic data refers to the observable characteristics resulting from the interaction of an organism's genotype with its environment. The integration of genomic and phenomic data is a powerful direction in research, leading to a more comprehensive understanding of biological functions. This integration can be beneficial in several areas:
- Personalized Medicine: By correlating genomic information with phenotypic traits, healthcare professionals can tailor treatments to individuals, enhancing efficacy and reducing side effects.
- Agricultural Improvements: Incorporating phenomic data in crop development can lead to the creation of new varieties that better withstand environmental stresses. Genomic insights can be paired with phenotypic traits to select for advantageous features more effectively.
- Disease Research: Understanding the link between genotype and phenotype can aid in deciphering the molecular mechanisms underlying complex diseases. This will allow researchers to identify potential therapeutic targets more accurately.
The convergence of genomic and phenomic data may transform our capacity to decipher biological complexities and drive innovative solutions across multiple disciplines.
In summary, the future directions in genomic data research, particularly through advancements in computational biology and the integration of phenomic data, highlight the transformative potential of this evolving field. The implications will not only enhance our knowledge of genetic functions but also provide tangible benefits in personalized healthcare and agricultural practices.
Ending
The conclusion of this article encapsulates the significance of genomic data in various sectors, particularly in healthcare, agriculture, and research. It provides a synthesis of the prior sections and emphasizes how genomic data serves as a foundational element in advancing scientific understanding and technological innovations.
Through the sections covered, we have explored how genomic data is collected, analyzed, and applied. This data facilitates precision medicine, enabling tailored treatment based on individual genetic profiles. Moreover, the integration of genomic data in agricultural biotechnology enhances crop yields and disease resistance.
Summary of Key Points
- Definition and Importance: Genomic data is essential for understanding the genetic basis of diseases. It plays a crucial role in research by providing insights into hereditary conditions and potential therapies.
- Types of Data: Key types include DNA sequence data, RNA sequence data, and epigenomic data. Each type serves unique purposes in the study of genetics.
- Collection Methods: Techniques like Next-Generation Sequencing and microarray technology are pivotal in generating vast amounts of genomic data.
- Applications: This data has transformative applications in clinical diagnostics, personalized medicine, and agricultural enhancements.
- Challenges and Ethical Considerations: Issues around data privacy, interpretation complexities, and ethical implications will shape the future of genomic research.
- Future Directions: Innovations in computational biology and the integration of genomic with phenomic data promise to enhance our understanding of biological systems.
The Importance of Genomic Data in the Modern World
In the contemporary landscape, the relevance of genomic data cannot be overstated. As we transition into a more personalized approach in medicine, understanding the genomic underpinnings of diseases will become increasingly significant. These insights are crucial not only for developing new treatments but also for improving public health strategies. Furthermore, as genomic data continues to evolve, its potential applications expand, presenting new opportunities for research and innovation.
Genomic data drives advancements in various fields, from diagnostics to gene therapy. For instance, it aids researchers in identifying biomarkers that predict disease susceptibility and progression. Moreover, the ethical aspects surrounding genomic data will demand careful consideration from both scientists and policymakers.
The future of genomic data research holds vast potential; however, it requires responsible stewardship. As we advance, a commitment to ethical practices and data security will remain paramount. Thus, understanding genomic data is not just about gathering information—it is about harnessing this knowledge to better our health and our world.
"The future of genomic data will redefine our approach to health, making it more personalized and efficient."
As we summarize the points discussed, it becomes evident that genomic data occupies a central role in shaping the future of science and medicine.