Sentiment Analysis in Trading: Emotions and Markets
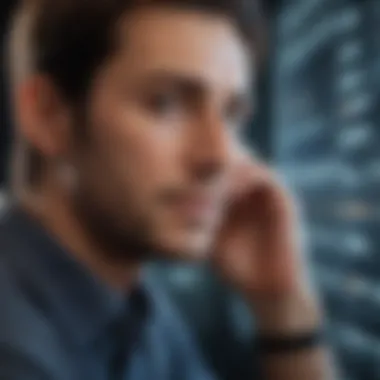
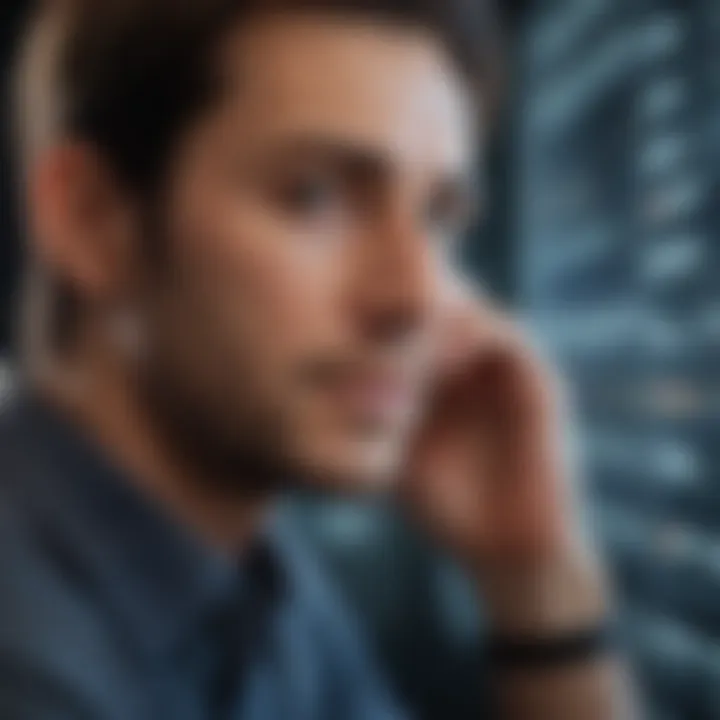
Intro
Sentiment analysis has emerged as a critical tool in the field of trading, intertwining the emotional states of various market participants with trading decisions and market dynamics. The influence of emotions on market behavior cannot be understated, as psychological factors often lead to irrational decisions that impact prices and trends. This article aims to dissect the methodologies that underpin sentiment analysis, evaluating how emotional indicators shape trading strategies.
Understanding sentiment analysis involves recognizing its foundational role in bridging the gap between human psychology and market fluctuations. Traders increasingly utilize this approach to gauge market sentiment through advanced data analytics, leveraging various sources of information to make informed trading decisions. Emotional indicators, when interpreted correctly, can provide significant insights into potential market movements.
This examination not only highlights how sentiment analysis affects trading but also evaluates the effectiveness of existing metrics and analytical methods. Through careful analysis, we will explore technological advancements that facilitate data processing, allowing traders to access real-time insights that were previously unattainable.
Research Overview
Summary of Key Findings
The exploration of sentiment analysis in trading reveals several key findings. First, emotional indicators derived from social media, news articles, and other platforms can serve as precursors for market trends. A rise in positive sentiment, for example, often correlates with bullish market behavior, while negative sentiment tends to lead to bearish trends.
Additionally, algorithms are now capable of analyzing vast amounts of unstructured data, enhancing the trading process. By assessing the public sentiment surrounding specific assets, traders can fine-tune their strategies to align with the collective mood of the market.
Research Objectives and Hypotheses
The primary objective of this research is to assess how sentiment analysis can predict market trends effectively. The hypotheses formulated include:
- Emotional indicators can be quantified to predict market movements.
- Social media sentiment is a reliable predictor of asset performance.
- Advanced algorithms improve the accuracy of sentiment analysis in trading.
Methodology
Study Design and Approach
A mixed-method approach will underpin this research. Combining qualitative data from trader interviews with quantitative data derived from market analysis enables a comprehensive evaluation of sentiment analysisβs impact on trading. The study will investigate both established methodologies and emerging practices in the field.
Data Collection Techniques
Key data collection techniques will include:
- Social Media Analysis: Scrutinizing platforms like Reddit and Facebook to gauge public sentiment about specific stocks.
- News Sentiment Analysis: Evaluating news articles and press releases for emotionally charged language regarding financial events.
- Market Data Correlation: Monitoring trading volumes and price fluctuations alongside sentiment indicators to draw correlations and insights.
The intersection of emotions and market dynamics forms a pivotal aspect of modern trading strategies, impacting decisions made by professional and amateur traders alike.
By diving into sentiment analysis, this article will enrich both novice and experienced tradersβ understanding, providing the necessary tools to navigate the complex landscape of emotional influences in trading.
Foreword to Sentiment Analysis in Trading
Sentiment analysis in trading represents a crucial intersection of emotional indicators and market behaviors. Understanding the underlying sentiments that drive trading decisions is fundamental for both novice and experienced investors. The rise of digital communication has made it possible to analyze sentiments from various data sources, enhancing market predictions and investment strategies. In this context, traders can leverage emotional data to gain insights that are not always apparent through traditional financial metrics.
By examining market sentiment, traders can identify trends and shifts in investor behavior. This approach enables them to make informed decisions based on how emotions can shape market dynamics. Furthermore, sentiment analysis can help mitigate risks by providing an additional layer of information, which can be particularly valuable in volatile markets. As such, grasping the nuances of sentiment analysis becomes imperative for those looking to navigate the complexities of trading.
Definition and Scope
Sentiment analysis refers to the computational method of analyzing textual data to determine the emotional tone behind it. In trading, this often involves studying social media posts, news articles, and financial reports to assess the prevailing mood of investors and market sentiment. The scope of sentiment analysis ranges from simple keyword tracking to sophisticated algorithms that utilize natural language processing for in-depth sentiment extraction. It aims to translate the qualitative emotions into quantifiable metrics that traders can use in their decision-making processes.
One important aspect to note is that sentiment analysis does not replace traditional analysis methods but complements them. Traders should consider both quantitative data and qualitative sentiment to form a well-rounded perspective on market movements. This dual approach allows for a more informed strategy that acknowledges the psychological elements at play in trading decisions.
Historical Context
Sentiment analysis is not a new concept, although its application in trading is relatively recent. The initial idea of gauging market sentiment can be traced back to the works of early economists and financial theorists, who recognized that emotions influence investor behavior. However, it was not until the digital age that sentiment analysis became a more systematic endeavor.
With the advent of social media platforms like Twitter and Facebook, the amount of data available for sentiment analysis grew exponentially. The growth of online forums and communities also contributed to this trend, as traders began sharing thoughts and opinions freely. Academics and financial analysts started developing methodologies for interpreting this data more systematically.
In recent years, the advancement of machine learning and natural language processing has revolutionized sentiment analysis in trading. Algorithms can now process large volumes of text quickly, enabling traders to react to market changes in real-time. This evolution reflects a broader trend in finance where technology and psychology converge, highlighting the importance of understanding emotions in market dynamics.
Understanding Market Sentiment
Understanding market sentiment is an essential component of trading and investment strategies. Market sentiment refers to the overall attitude of investors toward a particular security or financial market. It is influenced by emotions, cultural context, and psychological factors, making it a complex yet crucial aspect of trading behavior.
Recognizing market sentiment allows traders to gauge how collective emotions can impact stock prices and market trends. For instance, when market participants are overly optimistic, it can lead to inflated stock prices, whereas pessimism often drives prices down. This cyclical nature of sentiment can be leveraged to make more informed decisions. Here are some key benefits of understanding market sentiment:
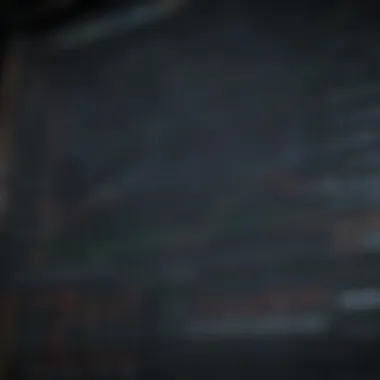
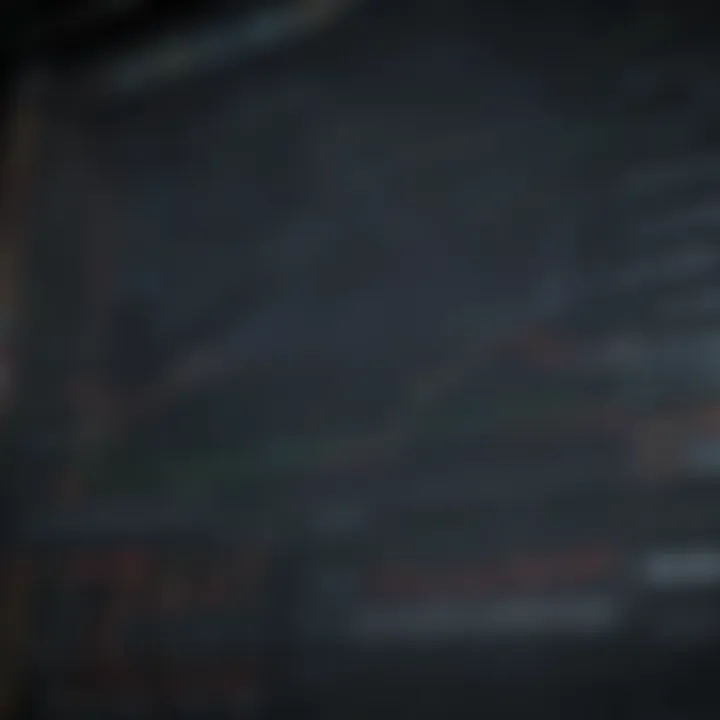
- Informed Decision-Making: By interpreting the sentiment surrounding an asset, traders can identify potential entry and exit points.
- Risk Management: Awareness of market mood can help anticipate potential downturns or overreactions in volatile markets.
- Behavioral Insights: Understanding the emotional drivers behind trades can provide insights into market trends and noise, aiding long-term strategies.
To effectively navigate market sentiment, one must consider various factors affecting it. Values, beliefs, and collective emotions all play a part. Therefore, traders must remain observant of external influences and market narratives to better interpret sentiment.
Psychological Factors in Trading
Psychological factors are critical when analyzing market sentiment. Investors are not always rational; emotions often drive their decisions, from enthusiasm to fear. Understanding these emotional responses can offer deeper insights into market behavior. Key psychological factors include:
- Fear and Greed: These two emotions frequently dominate trading behavior. Greed can lead to irrational exuberance, while fear can trigger panic selling.
- Herd Behavior: Market participants often follow the crowd, leading to rapid price movements based on group mentally rather than individual analysis.
Addressing psychological factors allows traders to develop strategies that mitigate emotional bias. Recognizing one's own emotional responses during trading can prevent unnecessary losses.
Role of News and Media
The role of news and media in shaping market sentiment is profound. Financial news outlets, social media, and market reports influence how traders perceive the value of assets. Events reported by the media can sway public opinion leading to immediate market reactions. For example, a companyβs quarterly earnings report can lead to significant stock price movements based on the sentiment conveyed in the report.
Moreover, the speed at which news spreads can amplify sentiment shifts. Social media platforms like Twitter and Reddit facilitate rapid dissemination of information, affecting trader psychology in real-time. The implications of this can be staggering, particularly in a volatile market where immediate reactions can lead to heightened volatility.
In summary, understanding market sentiment involves recognizing the complex interplay between psychological factors and media influences. By analyzing these elements, traders improve their ability to predict market movements and respond accordingly. Investing time in comprehending these dynamics is essential for anyone serious about mastering the complexities of trading.
Data Sources for Sentiment Analysis
In sentiment analysis, data sources act as the backbone that informs traders about market sentiment. Understanding where to gather relevant data is critical, as the quality and variety of sources can significantly affect trading decisions. The use of diverse data sources allows for a richer and more accurate representation of market emotions, which in turn enhances trading strategies. As sentiment often drives market movements, harnessing insights from various platforms can provide a competitive edge.
Social Media and Its Impact
Social media has rapidly become one of the most influential data sources in sentiment analysis. Platforms like Twitter and Facebook serve as real-time gauges of public opinion about stocks, commodities, and economic events. Traders analyze posts, comments, and interactions to determine the mood surrounding certain assets. For instance, a surge in positive sentiment on Twitter about a technology company may correlate with stock price increases. This immediate feedback loop is invaluable for traders seeking to capitalize on market movements.
However, one must be cautious about misinformation and hype. The spontaneous nature of social media means that sentiment can shift dramatically, often without fundamental backing. Therefore, while social media sentiment can be a powerful indicator, it requires validation from other sources.
Financial News Articles
Financial news articles provide structured and often in-depth analysis of market conditions. These articles typically discuss corporate earnings, macroeconomic indicators, or geopolitical events that may influence markets. Sentiment can be inferred from the language and tone of these articles. Positive outlooks can indicate bullish markets, while negative assessments might suggest bearish trends.
Traders frequently rely on aggregators to compile news from various outlets like Bloomberg and Reuters, thus enabling them to monitor the sentiment landscape efficiently.
Incorporating sentiment from financial news into trading strategies can provide a more comprehensive view of market conditions. However, it is essential to discern between subjective opinions and factual reporting. This critical evaluation enhances the reliability of sentiment metrics derived from these articles.
Market Research Reports
Market research reports present another vital source of sentiment analysis data. These reports, usually produced by financial institutions and market analysts, offer insights into trends and predictions based on extensive research. They often incorporate quantitative data and qualitative assessments, making them reliable for understanding market sentiment.
Sentiment derived from market research can help traders inform their strategies about potential future movements. They can highlight underlying strengths or weaknesses in sectors or specific assets based on current sentiment trends. Like other data sources, however, it is advisable to analyze multiple reports to ensure a well-rounded perspective.
In summary, the integration of various data sources enriches sentiment analysis, allowing traders to make informed decisions based on robust insights rather than isolated indicators. Leveraging social media, financial news articles, and market research reports collectively offers a comprehensive understanding of market dynamics that sentiment alone cannot achieve.
Techniques in Sentiment Analysis
Sentiment analysis plays a crucial role in the trading landscape. Understanding the emotions that drive market movements gives traders an edge in predicting trends and making informed decisions. This section will cover two major techniques essential for sentiment analysis: Natural Language Processing and Machine Learning Approaches. Each of these methodologies holds a significant position in how sentiment data is interpreted and applied in trading strategies.
Natural Language Processing
Natural Language Processing, or NLP, is a field of artificial intelligence that focuses on the interaction between machines and human language. Its importance in sentiment analysis cannot be overstated, as it allows traders to extract useful information from vast amounts of unstructured text data. With the continuous growth of social media and financial news, the ability to process and analyze text in an efficient manner is highly beneficial.
One of the key benefits of using NLP in sentiment analysis is its capability to identify and quantify emotions expressed in language. By applying techniques such as tokenization, stemming, and sentiment classification, traders can gauge the market sentiment towards particular stocks or assets. This enables them to respond to market conditions with precision.
Moreover, NLP helps in refining sentiment scores, which can be advantageous for assessing market reactions. For example, the algorithms can differentiate between positive, negative, and neutral sentiments among a sea of tweets or articles, providing clearer insights. Understanding data quality is also vital; NLP provides frameworks to filter out noise and focus on relevant linguistic patterns.
"NLP tools can turn unorganized data into structured insights, which is essential for informed trading decisions."
Machine Learning Approaches
Machine Learning (ML) approaches have transformed sentiment analysis by enabling models to learn from data automatically. In the context of trading, ML algorithms can analyze historical data to predict future market behavior based on emotional sentiment. Deploying ML enhances the accuracy and reduces the time needed to process extensive datasets.
Traders can leverage various ML techniques such as supervised learning and unsupervised learning based on the nature of their sentiment analysis needs. For instance, supervised learning can use labeled data to train models that identify sentiment in new, unseen data. Conversely, unsupervised methods are useful when dealing with unlabelled datasets, allowing models to discover patterns without prior examples.
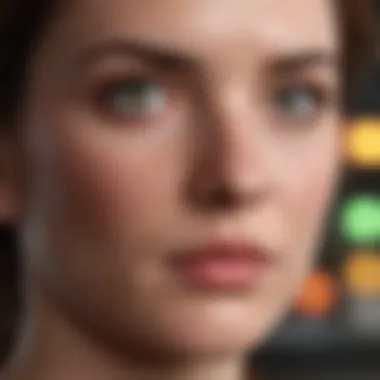
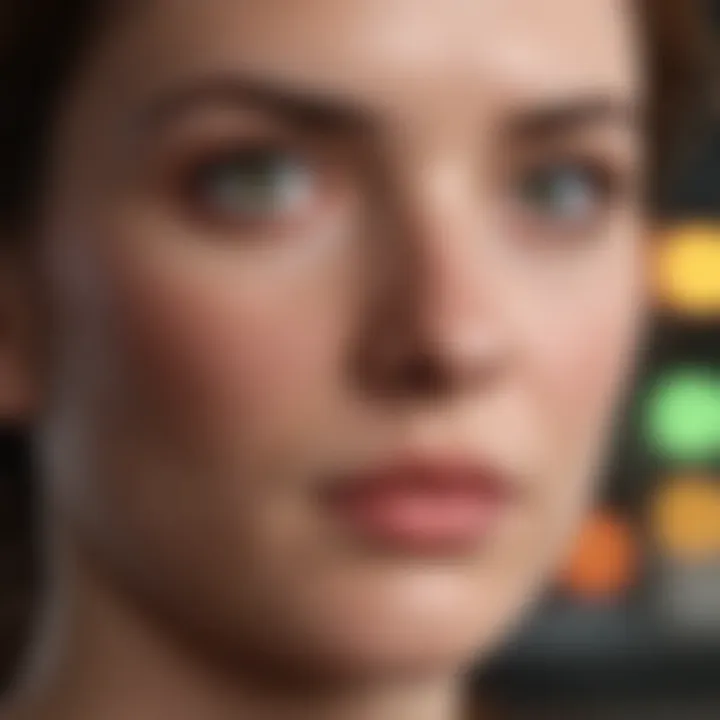
In practice, the implementation of these approaches can result in improved trading algorithms. For example, a machine-learning model could track sentiment across several platforms, assess its impact on specific stocks, and adjust trading strategies accordingly. Moreover, this adaptive learning model continues to improve as more data is fed into the system, leading to more robust predictions over time.
Additionally, combining ML with NLP creates a powerful synergy, where the strengths of both technologies enhance the analysis process. This collaboration allows traders to handle larger datasets and extract deeper insights into market dynamics, ultimately improving their decision-making processes.
By integrating both Natural Language Processing and Machine Learning, traders can better navigate the complexities of sentiment in the market. These techniques facilitate a deeper understanding of emotional influences and empower more precise trading strategies.
Measuring Sentiment: Metrics and Indicators
Understanding how to measure sentiment is crucial for traders utilizing this analytical approach. Metrics and indicators serve as the foundation for translating emotional data into actionable insights. They help in gauging the market's mood, allowing investors to make informed decisions based on current psychological trends.
When traders assess sentiment, they often turn to two primary metrics: sentiment scores and volatility indicators. Each of these provides unique perspectives on how emotions influence market behavior. Taking this approach enables traders to refine their strategies and to anticipate market movements more accurately.
Sentiment Scores
Sentiment scores are quantitative evaluations derived from various data sources, such as social media interactions, news headlines, and financial reports. These scores assess the positivity or negativity reflected in public sentiment regarding specific assets or markets.
Benefits of Sentiment Scores:
- Real-Time Analysis: They provide a snapshot of market sentiment at a specific time. This allows traders to gauge immediate reactions to events.
- Predictive Insights: Historical data shows that sentiment scores often correlate with price movements. A high positive score could indicate potential upward price action, while a low score may suggest declines.
- Risk Management: By understanding market sentiment, traders can manage risks more effectively, identifying overbought or oversold conditions.
"Sentiment scores are essential tools for navigating the complex emotions driving the market."
However, there are limitations to sentiment scores. They can be influenced by temporary events that may not have lasting effects. Moreover, false positives can arise from automated bots on social media, which can distort perceptions.
Volatility Indicators
Volatility indicators assess the degree of variation or fluctuation in a financial market. In the context of sentiment analysis, these indicators help traders understand how emotional swings can influence market volatility. High volatility often reflects significant uncertainty or emotional reactions from traders and investors.
Key Considerations with Volatility Indicators:
- Market Clarity: Volatility indicators provide insight into periods of uncertainty in the market. High volatility may suggest increased emotional trading, while low volatility could indicate stability.
- Trading Opportunities: Recognizing volatility can present trading opportunities, especially for short-term traders who aim to capitalize on rapid price movements.
- Psychological Assessment: By understanding volatility, traders can gauge broader market emotions. A market with heightened emotions may react disproportionately to news or events.
Though useful, volatility indicators also come with challenges. They may lag behind the actual market movements, and they can generate false signals that mislead traders.
In summary, measuring sentiment using metrics such as sentiment scores and volatility indicators provides traders with valuable tools for making decisions. These metrics can enhance trading strategies, helping traders navigate an emotionally driven market.
Application of Sentiment Analysis in Trading Strategies
Sentiment analysis plays a crucial role in trading strategies by providing insights into market mood and sentiment. Understanding the emotions behind market movements allows traders to make more informed decisions. The ability to capture the emotional landscape of traders offers distinct advantages, such as enhancing prediction capabilities and improving the overall effectiveness of trading strategies.
By leveraging sentiment analysis, traders can better navigate through market dynamics. This includes identifying potential trends and reversals that may not be evident through traditional analysis alone. Therefore, sentiment analysis can be seen as a necessary complement to multiple analytical techniques.
Algorithmic Trading
Algorithmic trading refers to the automated execution of trading strategies based on predefined criteria. The integration of sentiment analysis in algorithmic trading systems enhances their effectiveness. Algorithms can be programmed to assess sentiment from various data sources, such as social media feeds and financial news articles.
This approach allows for:
- Real-time analysis: Algorithms can process large volumes of data quickly, providing up-to-date sentiment readings.
- Enhanced decision-making: By factoring in market sentiment, algorithms can adjust their strategies according to prevailing emotions.
- Increased efficiency: High-frequency trading can benefit from sentiment signals, making rapid trades when favorable conditions present themselves.
Incorporating sentiment analysis transforms algorithmic trading into a more adaptive and responsive system. This results in potentially higher returns and reduced risks.
Long-Short Equity Strategies
Long-short equity strategies involve buying stocks expected to increase in value (long) while simultaneously selling short stocks expected to decline. Sentiment analysis adds a valuable layer of insight to these strategies. By analyzing sentiment data, traders can identify which stocks have positive sentiment and which are viewed unfavorably.
Considerations include:
- Positive sentiment for long positions: A strong bullish sentiment indicates a good opportunity to initiate long trades.
- Negative sentiment for short positions: Stocks with increased negative sentiment may present profitable shorting opportunities.
- Market timing: Incorporating sentiment data can enhance the timing of entering or exiting trades, providing a competitive edge.
The effectiveness of long-short strategies is significantly amplified when sentiment is deemed a key variable influencing the stock price, thus allowing for more precise positions.
Emotional Trading Strategies
Emotional trading strategies focus on human behavior and decision-making. These strategies use sentiment analysis to gauge the psychology of traders and the impact it has on market movements. Emotions such as fear and greed play pivotal roles in driving market trends and can be measured through sentiment indicators.
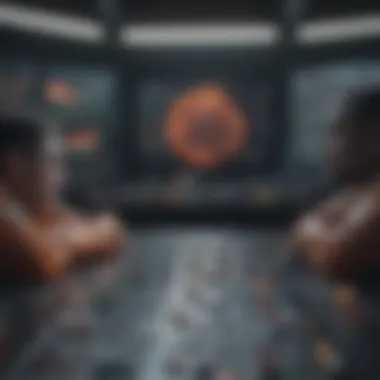
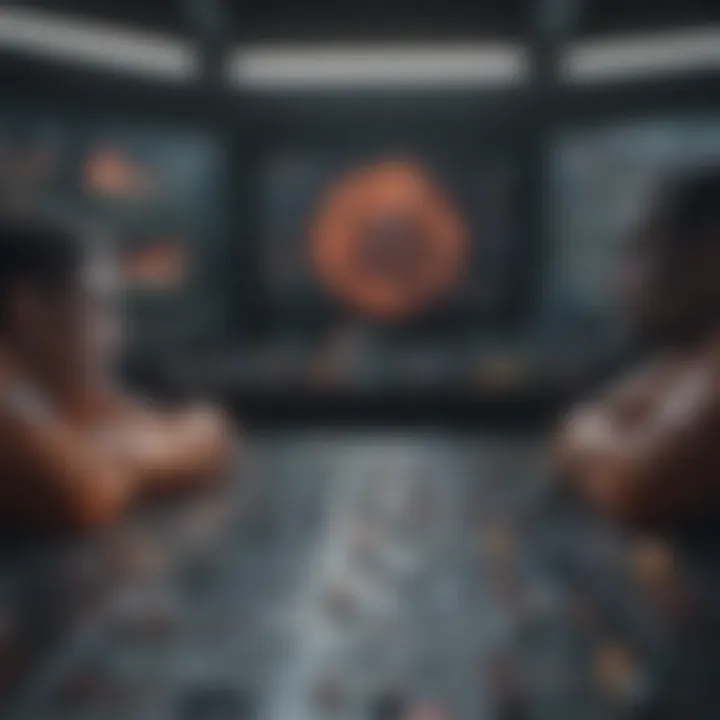
Key elements include:
- Behavioral patterns: Understanding common emotional responses can help traders anticipate market movements.
- Market sentiment indicators: Tools like the Fear & Greed Index provide traders insights into the prevailing emotional state of the market.
- Mitigation of biases: Sentiment analysis enables traders to recognize and counteract their emotional biases, leading to more rational trading decisions.
By understanding emotional dynamics, traders can create more sophisticated strategies that consider both sentiment and rational analysis, resulting in enhanced decision-making frameworks.
In summary, the application of sentiment analysis in trading strategies allows for a more comprehensive understanding of market behavior. Whether through algorithmic trading, long-short equity strategies, or emotional trading, sentiment analysis provides crucial insights that can significantly improve trading outcomes.
Limitations and Challenges
The integration of sentiment analysis into trading strategies, while promising, is not without its limitations and challenges. Acknowledging these hurdles is crucial for traders who seek to effectively utilize emotional indicators to inform their decisions. The nuances of market dynamics, combined with the inherent unpredictability of human behavior, necessitate a careful examination of potential pitfalls in sentiment analysis. By understanding these limitations, traders can better navigate the complexities of the financial landscape and enhance their strategies.
Data Reliability Issues
One of the foremost challenges in sentiment analysis is the reliability of data sources. As traders rely on diverse platforms, such as social media, news outlets, and financial reports, the quality and authenticity of information can vary significantly.
- Quality of Data
Not all social media platforms or news articles offer structured and credible information. For instance, Twitter posts may reflect impulsive reactions rather than informed opinions. This type of noise can skew sentiment analysis results, leading to misguided trading decisions. - Bias in Data
Another concern involves the inherent biases present in user-generated content. Sentiment can be influenced by emotions, misinformation, or market sentiment, making it difficult to ascertain the true market direction based on crowd behavior alone. - Volume versus Value
While a large volume of data may seem advantageous, it can lead to analytical overload. Finding valuable insights amidst an excess of information requires sophisticated tools and frameworks, which not all traders possess.
Adopting a critical approach to data evaluation is essential. Traders should cross-reference information from multiple sources and consider filters that account for biases or misleading data. This thorough scrutiny can assist in achieving a more accurate reading of market sentiment.
Market Volatility
Market volatility presents a further challenge for sentiment analysis in trading. High volatility can be a double-edged sword, amplifying both the potential for gains and the risks involved. This environment complicates how sentiment metrics should be interpreted.
- Rapid Changes
Markets can shift quickly due to external factors, such as geopolitical events or economic releases. In such conditions, sentiment analysis may not reflect the most current state of market psychology, leading to reactive instead of proactive strategies. - False Signals
During times of volatility, traders might encounter false signals from sentiment metrics. Emotional responses to sudden market movements can warp sentiment analysis results. Traders relying solely on these metrics might make rash decisions, ultimately experiencing losses. - Adaptability of Strategies
Effective trading strategies must adapt to changing market conditions. The same sentiment that influences market behavior in a steady environment might behave differently in a volatile scenario. Traders need to be aware of the limitations of sentiment analysis and integrate other market indicators to develop a more balanced strategy.
"Understanding market volatility is essential; it can lead to both opportunities and challenges in sentiment analysis."
Overall, both data reliability and market volatility present significant obstacles to effectively utilizing sentiment analysis in trading. A cautious, well-informed approach can help traders navigate these limitations, integrating sentiment analysis into a broader trading strategy with a clearer perspective on risks and opportunities.
Future Trends in Sentiment Analysis for Trading
The significance of sentiment analysis continues to expand as traders seek more reliable tools to interpret market behavior. In the trading environment, emotions can drive significant fluctuations in prices and trends. Understanding the future of sentiment analysis holds value for practitioners who aim to enhance their strategies and risk management approaches. An increased focus on technological advancements and their practical applications can result in better forecasting and more efficient trading decisions.
Artificial Intelligence and Improvement
Artificial intelligence (AI) is a game-changer in the realm of sentiment analysis. The advancement of machine learning algorithms enables more sophisticated data processing. AI has the capacity to analyze vast amounts of data from diverse sources in a fraction of the time humans can. This efficiency can lead to improved sentiment classification and thus, more accurate predictions of market trends.
Machine learning techniques, especially neural networks, allow for deeper insights into emotional cues from data, whether it comes from social media or financial news. These systems can learn from historical data, adjusting their models to enhance accuracy. Moreover, the integration of natural language processing (NLP) allows AI to understand nuances and context. This is crucial in sentiment analysis, as it can help differentiate between positive, negative, or neutral sentiments more effectively than traditional methods.
"The evolution of AI in sentiment analysis is not merely a trend; it represents a paradigm shift in how traders decipher market signals."
Integration with Other Analytical Tools
The future of sentiment analysis will also see an ecosystem where it is integrated with other analytical tools. Combining sentiment analysis with technical analysis could provide a more holistic view of market dynamics. Traders could leverage sentiment data alongside indicators like moving averages and relative strength indexes (RSI) to validate their strategies.
Additionally, the use of sentiment analysis in conjunction with fundamental analysis will consider both market psychology and economic conditions. This multifaceted approach can lead to more robust trading strategies. Traders can benefit from a clearer understanding of how emotional responses affect price movements in response to earnings announcements, geopolitical events, or product launches.
Culmination
The conclusion serves as a pivotal element in synthesizing key findings and insights discussed throughout the article. It reinforces the intricate relationship between sentiment analysis and trading strategies, highlighting its significance in todayβs financial landscape. Traders, both novice and experienced, can derive substantial benefits from understanding and implementing sentiment analysis into their decision-making processes.
In this article, we explored various methodologies, metrics, and indicators used in sentiment analysis. We uncovered how emotional indicators, whether derived from social media or financial news, can have profound effects on market dynamics. The application of advanced techniques such as natural language processing and machine learning positions sentiment analysis at the forefront of modern trading strategies.
While acknowledging the limitations and challenges of this field, it is clear that the future holds great promise. By continuously integrating sentiment analysis with other analytical tools, traders can refine their strategies and improve their market predictions. Therefore, the insights gained from this article should instigate thoughtful consideration about how sentiment analysis can be leveraged to navigate the complexities of financial trading.
Summary of Insights
Throughout our exploration, we identified key insights that define the landscape of sentiment analysis in trading:
- Emotional Indicators: Understanding market psychology and emotional responses can enhance decision-making.
- Data Sources: Social media, financial news, and market research reports are invaluable for gathering sentiment data.
- Analytical Techniques: The employment of natural language processing and machine learning enables more accurate sentiment interpretation.
- Strategy Application: Integrating sentiment analysis into algorithmic and emotional trading strategies can yield competitive advantages.
- Future Potential: The continuous evolution of AI and other technologies hints at promising developments in the effectiveness of sentiment analysis in trading.
These insights not only illuminate the current capabilities of sentiment analysis but also underscore its importance as a forward-looking tool for traders.
Final Thoughts
The interplay of emotions and market dynamics is increasingly becoming a cornerstone for successful trading strategies. Trading, which traditionally centered around technical and fundamental analysis, now welcomes the nuanced contributions of sentiment analysis. This evolution calls for traders to adapt and embrace the emotional undercurrents that shape market movements.
As we conclude, it is vital for traders to recognize sentiment analysis as more than a supplementary tool; it is often essential to comprehend market trends. Staying informed and utilizing data-driven sentiment metrics allows for better risk management and enhanced forecasting accuracy. In this way, sentiment analysis contributes meaningfully to the objective of achieving trading success, providing a comprehensive understanding of how emotional dynamics can impact financial markets.
By fostering an appreciation for sentiment analysis, traders may gain a strategic advantage in an ever-evolving market landscape.