Mastering Statistical Data Analysis Techniques
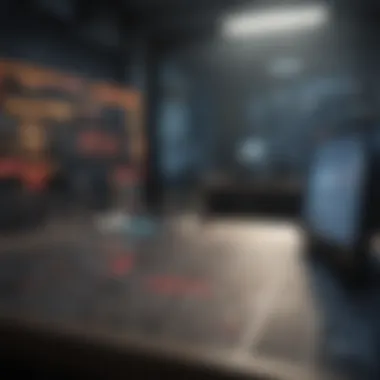
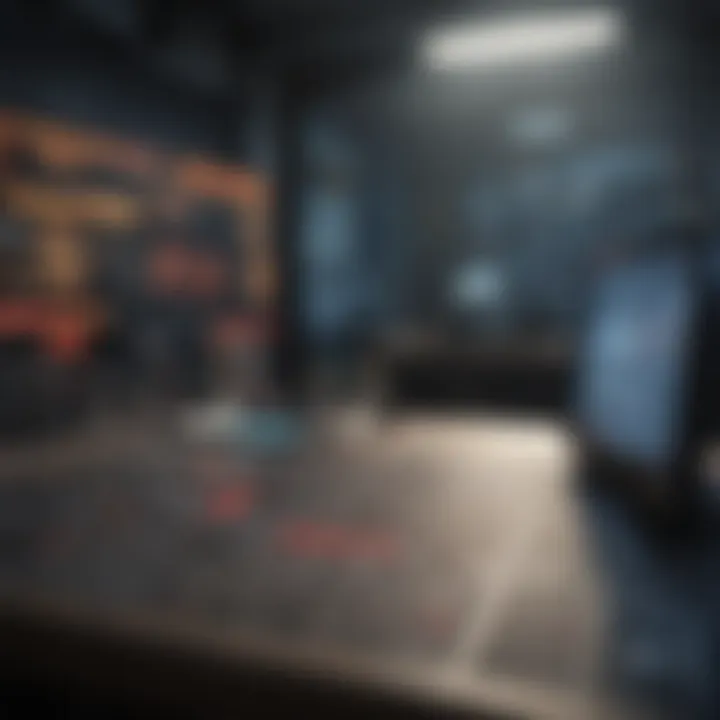
Intro
The art of data analysis in statistics is an essential skill for various professions and academic disciplines. It encompasses a wide range of practices and methodologies, aimed at extracting meaningful insights from raw data. Gaining a robust understanding of this subject not only aids in driving evidence-based decision-making but also enhances one's ability to interpret and communicate findings effectively. This article seeks to dismantle the complexities of statistical data analysis into manageable parts, drawing attention to the significance of each stage in the analytical process.
Research Overview
Summary of Key Findings
The landscape of statistical data analysis is rich and varied, with numerous techniques and methodologies at the disposal of the researcher. The key takeaways of this exploration include:
- Data Collection: Understanding how to gather data effectively is crucial. Methods like surveys, experiments, and secondary data sources all play a role.
- Analytical Techniques: Differentiating between descriptive and inferential statistics is fundamental. Descriptive statistics summarize existing data, while inferential statistics allow for predictions and generalizations beyond the study sample.
- Hypotheses Testing: Formulating and testing hypotheses is vital in guiding the analytical journey, providing a structured way to derive conclusions.
Research Objectives and Hypotheses
The primary aim of this article is to provide a systematic and detailed examination of statistical data analysis. Essential objectives include:
- Exploring different data collection methods and evaluating their appropriateness in diverse contexts.
- Investigating analytical techniques and their practical applications within various fields, from social sciences to natural sciences.
- Clarifying the role of hypotheses in data interpretation and fostering a critical understanding of data visualization tools.
The hypotheses underpinning this research assert that employing rigorous methods and techniques in data analysis leads to more reliable and insightful outcomes.
Methodology
Study Design and Approach
The study design emphasizes a comprehensive literature review combined with practical case studies. By correlating theoretical insights with real-world applications, this approach aims to bridge the gap between academic research and practical execution. Key steps in the design process include synthesizing existing literature and conducting analyses on actual datasets to illustrate the discussed concepts.
Data Collection Techniques
Data collection forms the backbone of any analytical process. Various techniques include:
- Surveys: Utilizing questionnaires to gather quantitative and qualitative data directly from respondents.
- Experiments: Conducting controlled experiments to observe effects and relationships within the data.
- Secondary Data: Leveraging existing datasets from credible sources for analysis, which can save time and resources.
Each of these methods comes with its strengths and limitations, which must be weighed carefully when embarking on any research endeavor.
"In statistics, data is only as valuable as the methods used to analyze it."
Understanding the nuances of these data collection methods will empower readers to choose the most suitable options for their unique research contexts. This foundational knowledge paves the way for a deeper engagement with advanced analytical techniques that come later in the statistical analysis process.
Foundations of Statistical Analysis
The concept of statistical analysis serves as the bedrock for making sense of data. In any statistical inquiry, understanding the fundamental aspects of data is crucial. Foundations of statistical analysis encompass various components like definitions, principles, and methods that guide practitioners in transforming raw data into actionable insights. These principles not only enhance comprehension but also improve decision-making, making this section essential to anyone engaging with data.
Understanding Statistics
Understanding statistics means grasping the ways data can be collected, analyzed, and interpreted. Statistics can be divided broadly into descriptive and inferential statistics. Descriptive statistics summarize and describe the characteristics of a data set. Examples include measures such as mean, median, and mode. On the other hand, inferential statistics deal with drawing conclusions from a sample and applying those conclusions to a broader population.
This understanding is fundamental to any researcher or professional using statistical methods. A strong grasp of statistics allows for proper data interpretation, ensuring that findings are not only insightful but also valid in their application. Therefore, individuals must have a working knowledge of key statistical terms and concepts. Some key aspects include:
- Basic terminology in statistics such as population, sample, variable, and bias.
- Different types of data, whether qualitative or quantitative.
- The importance of statistical reasoning in everyday life and professional environments.
Importance of Data Analysis
Data analysis is a critical endeavor as it unlocks the potential insights held within data. In today's data-driven world, organizations rely heavily on data analysis to inform strategies, improve efficiency, and drive decisions. For instance, in healthcare, data analysis helps to track disease outbreaks and patient outcomes, thus informing public health policies.
Several essential reasons underscore the importance of data analysis:
- Enhances decision-making: Organizations use data analysis to make decisions based on evidence rather than intuition.
- Identifies trends and patterns: Data analysis allows for the examination of past data to forecast future events.
- Supports risk management: By understanding data, potential risks can be identified and managed more effectively.
"The journey from raw data to meaningful insights is one of the most important processes in any analysis."
In summary, the fundamentals of statistical analysis form the backdrop against which deeper analytical techniques are applied. Grasping these foundational elements equips individuals with the necessary tools to navigate complex data landscapes effectively.
Data Collection Techniques
Data collection techniques are crucial for obtaining accurate and relevant data which is essential for analysis. The methods chosen can deeply influence the conclusions drawn from any statistical analysis. Proper data collection ensures that the results are reliable and valid, making it a foundational step in any research project or analytical study.
Quantitative vs Qualitative Data
Quantitative data is numerical and can be measured, analyzed statistically, and used to identify patterns or relationships. Examples include age, height, and income. The benefit of quantitative data is its ability to provide clear, objective information that can be analyzed using statistical methods. Conversely, qualitative data is descriptive and subjective, capturing information that can be observed but not measured. This includes opinions, experiences, and feelings. A benefit of qualitative data is its depth, allowing for richer insights into complex issues. We can note that both types of data are important in different contexts. They can even complement each other to offer a more rounded view of the subject matter.
Surveys and Experiments
Surveys are a popular method for collecting data, particularly when trying to gather opinions or attitudes across a larger population. They can be conducted in various ways, including online, face-to-face, or through phone calls. Experiments enable researchers to manipulate variables to observe outcomes in a controlled environment. This method can be useful in establishing cause-and-effect relationships. Both methods have their pros and cons; surveys might not yield in-depth data while experiments can be costly or impractical in some situations. Adequate design in these methods leads to better data quality, thereby enhancing the overall analysis.
Sampling Methods
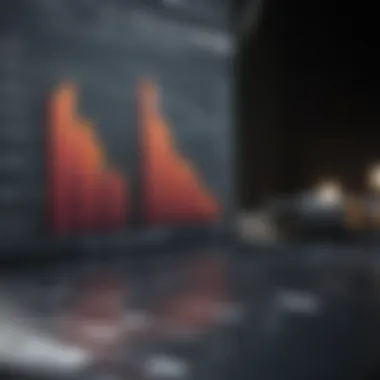
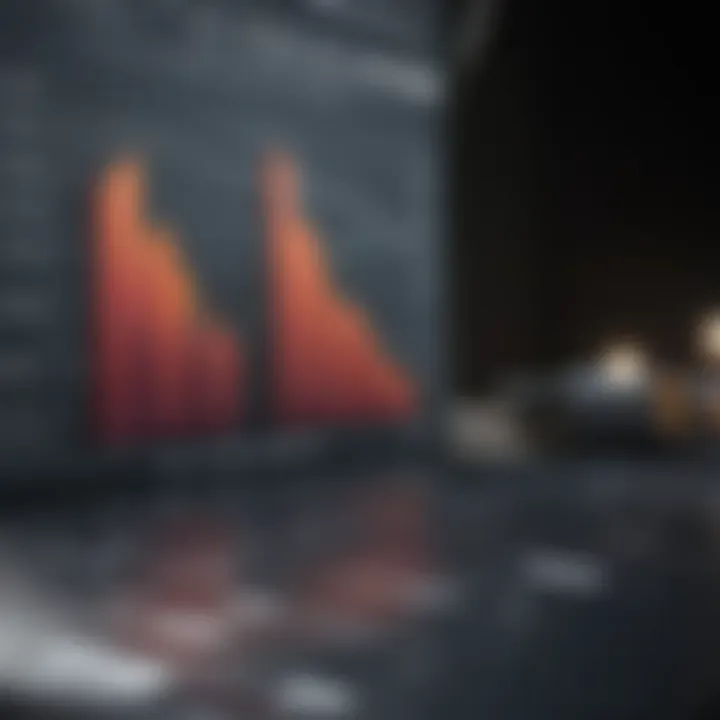
Sampling methods are vital to ensure that the data collected represents the population accurately without surveying everyone. There are several approaches to sampling, each with unique contributions.
Random Sampling
Random sampling is a technique where every individual has an equal chance of being chosen. This method's key characteristic includes the elimination of bias in selection, leading to results that can be generalized across the larger population. It is a beneficial choice because it allows for the broader application of findings. However, one unique feature of random sampling is its requirement for a comprehensive list of the population to avoid inaccuracies, which can sometimes be difficult to attain.
Stratified Sampling
Stratified sampling divides the population into subgroups and samples from each one. This method's key characteristic ensures that specific segments are represented, which is crucial in studies where these subgroups may display different behaviors or responses. It is ideal for enhancing the precision of results in diverse populations. One drawback can be the complexity in design, as researchers must identify key segments and recruit participants accordingly.
Systematic Sampling
Systematic sampling involves selecting every nth individual from a list. The key characteristic of this approach is its ease of use compared to random sampling. This method is beneficial due to its straightforward execution, which can save time in data collection. A unique feature can be that if the list from which sampling occurs has a hidden pattern, it may introduce bias into the sample selection. Therefore, careful consideration needs to be taken in its application.
Data Organization Techniques
Data organization techniques are crucial to the analysis of statistics. Properly organizing data can streamline the analytical process, making it easier to draw insights. It involves sorting through collected data and preparing it for analysis. This section discusses two main aspects of data organization: data cleaning and data structuring. Each of these aspects has significant contributions and considerations when working with data.
Data Cleaning
Data cleaning is essential for ensuring the integrity of the data before analysis begins. It involves identifying errors, inconsistencies, and inaccuracies within the dataset. One specific aspect of data cleaning is identifying outliers.
Identifying Outliers
Identifying outliers pertains to the process of detecting data points that deviate significantly from the typical patterns in a dataset. Outliers can result from errors in data collection or truly reflect unusual variations in the data. Identifying these outliers is beneficial for several reasons.
The key characteristic of identifying outliers is its ability to provide a clear view of data accuracy and make the dataset manageable. By isolating outliers, analysts can decide whether to investigate further or exclude these points from their analysis.
A prominent feature of this process is its impact on statistical conclusions. Outliers may influence the results, leading to misleading interpretations. This consideration is vital for maintaining the reliability of statistical findings. However, a disadvantage is that discarding outliers may result in the loss of valuable insights or significant variations in the data, impacting conclusions in unexpected ways.
Handling Missing Values
Handling missing values is another critical aspect of data cleaning. This process involves determining how to deal with gaps in the data where information is absent. Addressing missing values is essential to maintain the dataset's usability and reliability.
A key characteristic of handling missing values is its direct influence on data completeness. Missing information can lead to biased results if not addressed appropriately. Various strategies exist to manage these instances, ranging from exclusion to imputation.
One unique feature of handling missing values is the choice of approaches. For example, mean substitution might be popular, but it can reduce variability in the data set. Disadvantages of certain techniques include the risks of introducing biases or overfitting the data to suit analysis needs. Understanding these implications is crucial for effective data analysis.
Data Structuring
Data structuring is the systematic organization of data into formats that facilitate easy access and analysis. It plays a significant role in making datasets comprehensible to analysts and software. Here, we will explore three common data structures: tables, databases, and data frames.
Tables
Tables are one of the most basic and widely used structures for organizing data. They present data in rows and columns, where each column represents a variable, and each row corresponds to an observation. Tables are beneficial because they provide straightforward visibility into the dataset's attributes.
The unique feature of tables is their simplicity, which allows users to visualize and compare data easily. However, they can become cumbersome with large datasets. The distinct advantage is that they serve as a foundational format for storing data. Still, their limitations arise when dealing with complex datasets that require more sophisticated arrangements and querying capabilities.
Databases
Databases offer a more advanced way of organizing data. They store vast amounts of information while ensuring easy retrieval and management. Databases can support various data models, enhancing flexibility in how data is structured and accessed.
A key characteristic of databases is their capacity to handle relationships between multiple data tables. This aspect makes them a popular choice for large-scale data projects, where data interconnectivity is crucial. They allow for standardized data handling and may offer various advantages, like improved data integrity and security. However, the complexity of databases can be a disadvantage, especially for novice users who may struggle with managing and querying the data effectively.
Data Frames
Data frames are an especially popular choice within programming environments like R and Python. They provide a structured way to store data in rows and columns, much like tables but with added functionalities. Data frames enable users to apply various operations and analyses with ease, making them highly effective for analytical tasks.
The key characteristic of data frames is their dynamic nature, allowing operations on datasets without substantial overhead. This feature greatly benefits researchers and analysts who require efficient data manipulation. Yet, their reliance on specific programming environments may pose challenges for those not familiar with coding. With their advantages and limitations, data frames serve an important role in modern data analysis.
Descriptive Statistics
Descriptive statistics plays a fundamental role in summarizing and presenting data in a comprehensible form. It provides essential tools for data analysis by offering numeric summaries and visualizations that capture the main features of a dataset. This section explores two primary aspects: measures of central tendency and measures of dispersion. Together, they yield insights into the characteristics of the data set, aiding both researchers and decision-makers in their interpretations.
Measures of Central Tendency
Measures of central tendency are essential as they summarize essential information about a data set, providing a single value that represents the entire data collection. The three main measures include mean, median, and mode. Each measure offers a different perspective on the data.
Mean
The mean, often called the average, is calculated by adding all data points and dividing by the number of observations. Its contribution to data analysis is significant as it reflects the overall level of the dataset. The mean is a popular choice for many reasons: it considers every value in the dataset, making it comprehensive. However, it is sensitive to extreme values or outliers, which can skew the results. When dealing with datasets that might contain anomalies, this characteristic can present challenges, potentially leading to misinterpretations.
Median
The median represents the middle value of a dataset when arranged in ascending order. It serves as a vital measure of central tendency when analyzing skewed distributions. The median is less affected by outliers compared to the mean, making it a reliable indicator in circumstances where extreme values exist. This aspect of the median emphasizes its suitability for datasets that have non-normal distributions.
Mode
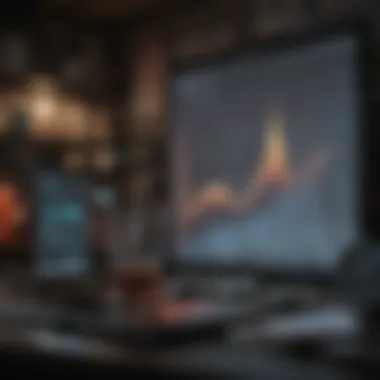
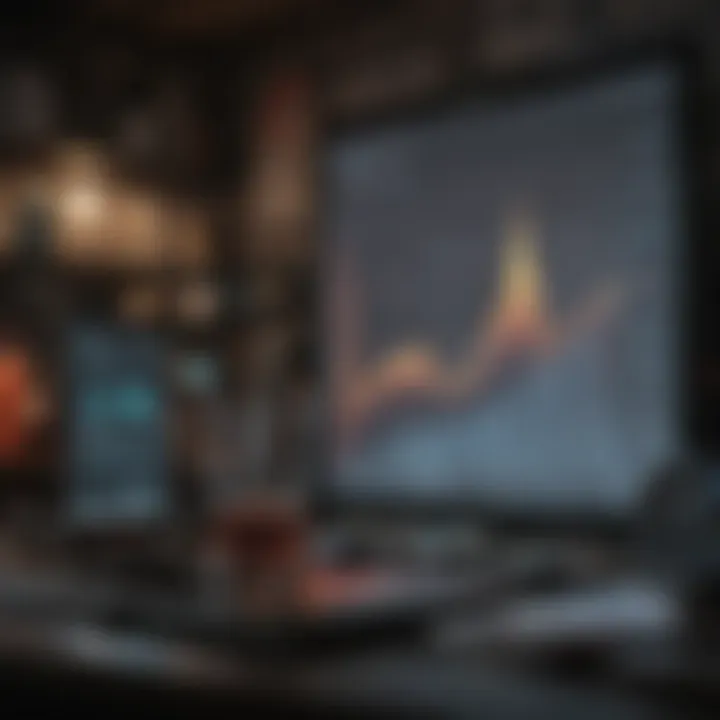
The mode indicates the most frequently occurring value in a dataset. This measure is beneficial in identifying trends within categorical data, where the mean or median might not apply. The mode can provide significant insights, especially in cases where understanding the most common occurrence is desirable, such as in marketing analysis or customer preferences. However, it is not always representative of the dataset as some datasets may not have a mode or may have multiple modes, adding complexity to its utility.
Measures of Dispersion
While measures of central tendency summarize the data, measures of dispersion describe the extent of variability within the dataset. These statistics help to understand the distribution of data points relative to the center.
Range
The range is the simplest measure of variability, calculated by subtracting the minimum value from the maximum value. It provides a basic overview of how spread out the values are, and it is particularly straightforward to compute. However, the range does not provide insights into the distribution of values within that span, which can result in a limited understanding of the dataset's characteristics.
Variance
Variance measures how far each data point in the set is from the mean and squares these deviations. It is a critical measure since it quantifies the overall variability in the dataset. Higher variance indicates that data points are widely spread out, while low variance suggests they are clustered close to the mean. Understanding variance is vital for statistical modeling and for assessing the reliability of the dataset.
Standard Deviation
Standard deviation is the square root of variance and provides a more interpretable value for data analysis. It indicates how much variability exists from the mean. A low standard deviation means that the values tend to be close to the mean, while a high standard deviation indicates a wider spread. This measure is crucial in comparing variability across different datasets, highlighting its importance in both descriptive and inferential statistics.
Understanding both descriptive statistics and measures of dispersion provides essential insight for further statistical analysis, making it easier to draw conclusions based on the collective information.
Inferential Statistics
Inferential statistics is a crucial element in the realm of statistical analysis. It allows us to make predictions and generalizations about a population based on a sample. This is important because often, it is impractical or impossible to collect data from every individual in a population. By utilizing inferential statistics, researchers can draw conclusions that extend beyond their immediate data, leading to broader insights and potential applications across different fields.
Hypothesis Testing
Null Hypothesis
The null hypothesis is a foundational concept in inferential statistics. It posits that no effect or difference exists between specified populations or variables. Essentially, it serves as a default position that indicates the absence of a relationship. One significant characteristic of the null hypothesis is its simplicity. Researchers often prefer it because it provides a clear basis for statistical testing.
Its main contribution to hypothesis testing lies in its role as a benchmark. By assuming no effect, researchers can examine if observed data significantly deviates from what the null hypothesis forecasts, thus establishing whether their findings warrant a different conclusion.
However, while the null hypothesis is beneficial for structuring tests, it also has limitations. One common issue is that it can lead to the acceptance of false negatives, where researchers fail to identify a significant relationship when one truly exists. This is labeled as a Type II error.
Alternative Hypothesis
In contrast, the alternative hypothesis provides a different scenario, suggesting that there is indeed an effect or difference present. This hypothesis is typically more specific than the null. A key characteristic of the alternative hypothesis is its ability to encompass several possible outcomes that oppose the prediction made by the null hypothesis. This makes it a popular choice for researchers aiming to explore the implications of their data.
The unique feature of the alternative hypothesis also lies in its flexibility. Researchers can adjust it according to the nature of their study, making it particularly advantageous when designing experiments. However, one disadvantage can be that it may lead to increased complexity in interpretation. For instance, confirming an alternative hypothesis can sometimes prove cumbersome if not carefully defined from the beginning.
Confidence Intervals
Confidence intervals represent an essential part of inferential statistics. These intervals give a range of values that are used to estimate a population parameter. The confidence interval conveys how confident we are about our estimation, typically expressed in percentages, like 95% or 99%. This statistic provides transparency and assists in understanding the margin of error associated with estimates.
Statistical Significance
Statistical significance plays a vital role in inferential statistics. It assesses whether the results of a study are likely to be due to chance. When a result is statistically significant, it suggests that the null hypothesis may not hold true, and there is a noteworthy effect.
Understanding statistical significance is crucial as it guides researchers in their interpretations. However, itβs important to note that significance does not equal practical relevance, meaning statistically significant findings may not always translate into significant implications in real-world applications. Hence, researchers must explore both statistics and context to make informed decisions.
In summary, inferential statistics enables researchers to extend findings from samples to broader populations, grounded in concepts like hypothesis testing and confidence intervals.
Data Visualization Techniques
Data visualization techniques are essential in effectively communicating and interpreting statistical data. These methods allow researchers to present complex data in a more understandable format. Visual representation can reveal trends, patterns, and insights that numeric data alone may obscure. Through various tools, one can enhance the interpretability of data, facilitating better decision-making and detailed analyses. This section elaborates on different visual formats that can be utilized in data analysis.
Graphs and Charts
Graphs and charts serve as the backbone of data visualization, encompassing various formats.
Histograms
Histograms are crucial for understanding the distribution of data. They display the frequency of data points within specific ranges, assisting in recognizing patterns within large datasets. One key characteristic of histograms is their use of bins, which group data into intervals, highlighting how often each range occurs.
Histograms are beneficial in showing the shape of the data distribution, including normality or skewness. The unique feature is their ability to represent continuous data effectively. While they provide clear visibility on data distribution, histograms can lack details regarding individual data points, which sometimes may lead to misinterpretation if not designed carefully.
Bar Charts
Bar charts are a popular choice for comparing different categories. They use rectangular bars to represent data values, making it easy to see differences at a glance. One key characteristic of bar charts is their ability to handle both qualitative and quantitative data. The bars' height or length correlates with their respective values, allowing for straightforward comparisons.
The benefit of using bar charts is their simplicity and ease of understanding. However, they may fall short if many categories are involved, which can lead to a cluttered appearance. Overlapping categories could dilute the message, requiring careful design to maintain clarity.
Pie Charts
Pie charts represent data in a circular format, dividing the circle into slices. Each slice's size is proportional to the data it represents. A notable characteristic of pie charts is their effectiveness in showing the part-to-whole relationships among categories.
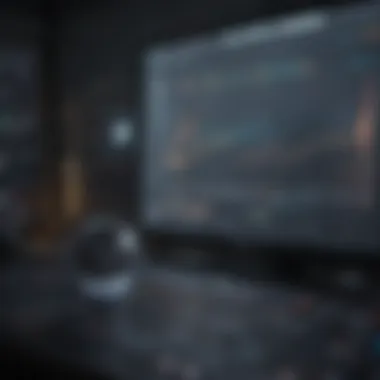
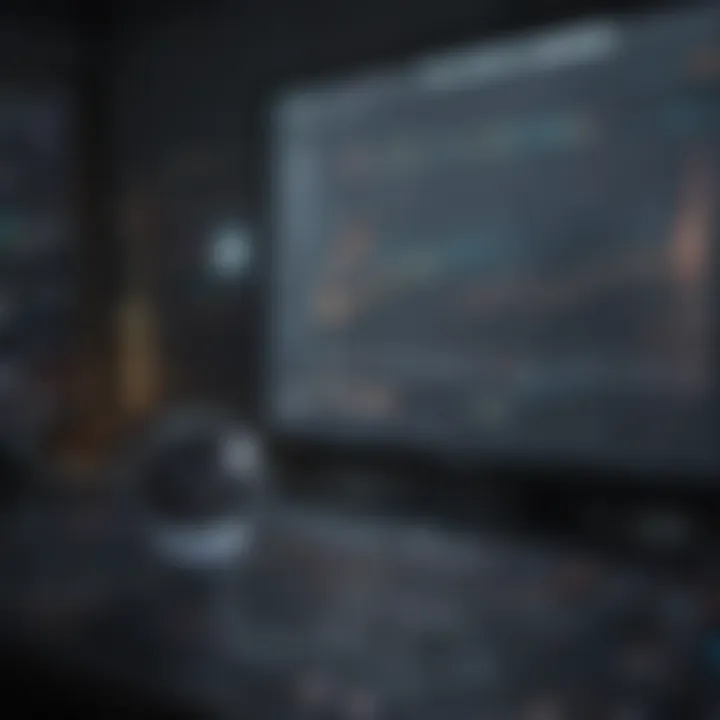
They are particularly beneficial for visualizing simple data distributions. Nevertheless, pie charts have limitations, especially in presenting a large number of categories, which can make it difficult to compare slices accurately. Care must be taken so that pie charts do not oversimplify complexities inherent in the data.
Scatter Plots
Scatter plots are vital for illustrating the correlation between two quantitative variables. Each point on the plot corresponds to a data point, revealing relationships or trends over a Cartesian plane. The most important feature of scatter plots is their ability to show the strength and direction of correlation.
This technique is beneficial for identifying outliers and understanding the data's behavior. However, scatter plots can become confusing with dense data. Too many data points can overlap, making it difficult to discern unique relationships clearly.
Heat Maps
Heat maps offer another level of data visualization by using color to represent different values in two dimensions. Each color indicates a range of values based on some metric, effectively providing a visual summary of information. Heat maps can display complex datasets in a condensed format, making it easier to identify areas of interest without extensive textual analysis.
This visualization method is notably beneficial for large datasets, such as those found in big data analytics across multiple variables. However, readers may misinterpret colors without a proper legend, highlighting the need for thoughtful design.
Practical Applications of Data Analysis
The field of data analysis plays a crucial role in various industries. Understanding these practical applications helps illuminate how data informs decisions and drives progress. Through targeted insights, data analysis enables organizations to optimize processes and outcomes. This section explores the importance of applying data analysis in real-world situations and highlights its relevance to industry-specific applications.
Industry-specific Applications
Healthcare
In the healthcare sector, data analysis is invaluable. It assists in patient care, treatment effectiveness, and operational efficiency. One key characteristic of healthcare is its reliance on real-time data. This capability allows for swift decision-making in critical situations. Furthermore, healthcare systems can leverage data to identify trends in patient populations and disease outbreaks. The unique feature of this industry is the use of predictive analytics. This technology forecasts patient needs based on historical data, ultimately leading to better healthcare outcomes. However, maintaining patient data privacy poses challenges and requires strict adherence to regulations.
Finance
Finance is another sector that integrates data analysis extensively. Financial institutions utilize data for risk assessment, fraud detection, and investment strategies. This industry's key characteristic is its emphasis on data accuracy. Accurate financial data ensures robust decision-making and enhances profitability. Additionally, finance employs techniques such as regression analysis for market predictions. A unique feature in finance is the use of algorithmic trading, which enhances trading efficiency. However, it is important to consider the disadvantage of over-reliance on automated systems, which may overlook critical human insights.
Marketing
Data analysis profoundly impacts marketing strategies. Marketers analyze consumer behavior, preferences, and trends to tailor their messages effectively. A significant characteristic of marketing is its dynamic nature. The fast-evolving landscape demands continuous data analysis to stay relevant. Moreover, marketers use A/B testing to optimize campaigns. A unique feature in marketing is the ability to segment audiences. This allows for focused communication, improving customer engagement. However, the challenge lies in managing vast amounts of data while avoiding analysis paralysis.
Case Studies
Examining specific case studies can illustrate how data analysis improves outcomes. For example, consider a healthcare organization that utilized predictive analytics to reduce hospital readmission rates. Their findings led to strategic interventions for at-risk patients. In finance, a firm may analyze trading patterns to identify successful investment avenues. Lastly, a marketing agency that analyzed social media interactions might discover key insights into consumer preferences, informing future campaigns.
In summary, understanding the practical applications of data analysis across industries informs how various sectors achieve their goals. By examining healthcare, finance, and marketing, it becomes clear that effective data analysis leads to informed decision-making, resource optimization, and improved outcomes.
Ethical Considerations in Data Analysis
In the realm of data analysis, ethical considerations serve as foundational pillars that guide researchers, analysts, and practitioners in responsibly handling data. The essence of ethical data analysis lies not only in the accuracy of results but also in ensuring that the rights and privacy of individuals are respected. This section aims to articulate these ethical dimensions, focusing on two critical areas: data privacy and responsible use of data.
Data Privacy
Data privacy is paramount in any analytical endeavor. It refers to the proper handling, processing, and storage of individuals' personal information. The importance of data privacy cannot be overstated; violations can lead to severe repercussions, including legal penalties and loss of public trust.
When engaging in data collection, it is crucial to obtain informed consent from participants. This means clearly explaining the purpose of data collection and how the data will be used. Failure to do so undermines the ethical integrity of the research. Moreover, anonymizing data is a best practice that helps protect individual identities.
Adhering to regulations like the General Data Protection Regulation (GDPR) ensures that organizations are legally compliant while safeguarding individual rights. Using secure storage methods and encryption further fortifies data privacy, preventing unauthorized access.
"Ethical data analysis is not solely about adherence to laws, but also about fostering trust and accountability in research practices."
Responsible Use of Data
Responsible use of data encompasses a variety of principles that emphasize accountability and integrity in analyzing data. Analysts must be aware of the implications of their findings and interpretations. Misrepresentation of data can lead to misguided decisions, affecting individuals and communities.
It is essential to maintain transparency throughout the analysis process. Analysts should document their methodologies, choices made, and potential biases. This allows for reproducibility and critical evaluation by peers. Furthermore, ethical considerations demand that analysts refrain from cherry-picking data or manipulating it to produce favorable outcomes.
Additionally, understanding the societal impact of data use is vital. Data-driven decisions can perpetuate systemic biases if not addressed carefully. Analysts must consider the consequences of their results on different demographics, ensuring equity in their applications.
In summary, ethical considerations in data analysis guide the conduct of researchers and analysts, fostering responsible practices that respect individual rights and promote trust in the findings.
Future Trends in Statistical Analysis
The field of statistical analysis is rapidly evolving, with new methodologies and technologies emerging continuously. Understanding the future trends in statistical analysis is crucial for practitioners, educators, and students alike. Mapping out these trends can provide insight into how analytics will become more sophisticated and integrated into various industries. Key elements include the rise of big data analytics and the integration of machine learning, both of which hold substantial promise for enhancing data interpretation and decision-making processes.
Big Data Analytics
Big data analytics refers to the process of examining large and complex datasets to uncover hidden patterns, correlations, and trends. This area is gaining momentum because of the exponential growth in data generation across multiple sectors, including healthcare, finance, and social media. Companies are increasingly leveraging big data analytics to gain a competitive edge. The benefits of big data analytics include:
- Enhanced Decision Making: Organizations can make informed decisions based on real-time data models that predict outcomes effectively.
- Improved Efficiency: Businesses can optimize workflows and reduce operational costs by utilizing data insights to streamline processes.
- Personalized Customer Experiences: Tailoring services or products to individual customer preferences leads to greater satisfaction and retention.
Incorporating big data into statistical analysis also brings challenges. Data privacy remains a significant concern, necessitating strict adherence to ethical standards.
Machine Learning Integration
The integration of machine learning in statistical analysis has the potential to revolutionize how data is processed and interpreted. Machine learning algorithms can learn from data patterns without explicit programming. This autonomous capability allows for:
- Automated Analysis: Routine data analysis tasks can be automated, allowing analysts to focus on higher-level interpretations.
- Predictive Modeling: Machine learning enhances predictive analytics, leading to more accurate forecasts in sectors like finance and healthcare.
- Adaptive Learning: Algorithms can continuously improve as new data becomes available, allowing for ongoing refinement of analyses.
Employing machine learning does come with considerations. The need for skilled professionals who can bridge the gap between statistical knowledge and technical expertise becomes more critical. Moreover, there is increasing emphasis on transparent algorithms to foster trust in automated outcomes.
As organizations adopt these advancements, the significance of continuous learning and ethical considerations in statistical practices cannot be overstated.