Understanding Fractal Markets: Theory and Applications
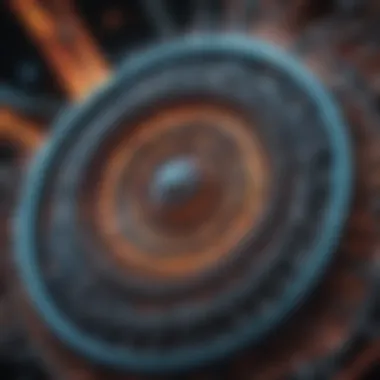
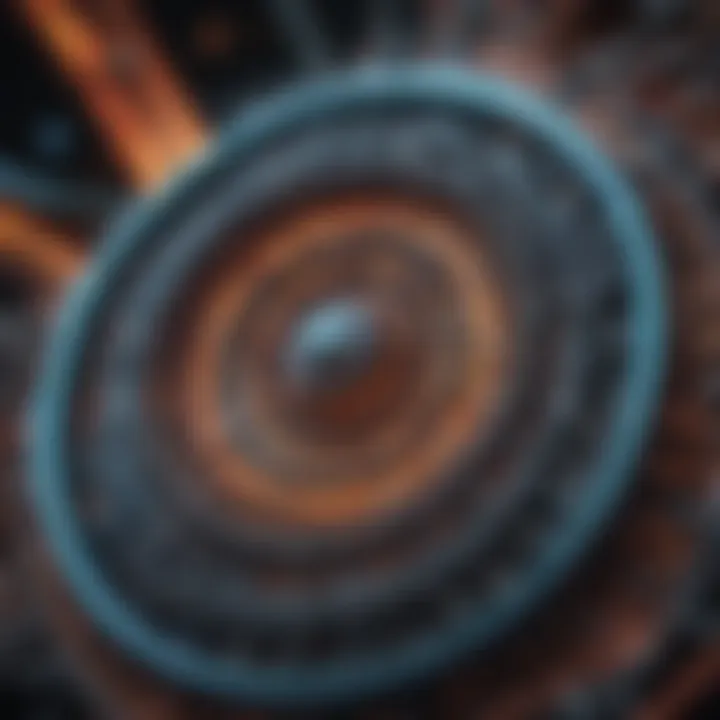
Intro
The concept of fractal markets presents a nuanced perspective on the complexities of financial systems. By integrating principles from fractal geometry, the theory encompasses a deeper understanding of market dynamics that traditional financial theories often overlook. This exploration reveals how self-similarity and market behaviors intertwine, providing insights that extend beyond mere statistical models.
The article aims to dissect these intricate ideas, making them accessible to a diverse audience that includes students, researchers, and professionals in economics and finance. The forthcoming sections will lay the groundwork for comprehending the foundational principles of fractal markets, the inherent implications for economic modeling, as well as real-world applications and critical perspectives on the theory.
Preface to Fractal Markets
Fractal markets present a compelling framework for exploring the complex dynamics of financial systems. Understanding these markets is essential for scholars, professionals, and even casual investors. This section serves to establish a strong foundation for the broader discussion on fractals and their implications in the financial world.
In essence, fractal markets challenge traditional economic theories, such as the Efficient Market Hypothesis. They suggest that markets do not behave in a random or entirely predictable manner. Instead, market phenomena can be observed as patterns that repeat across different scales. This self-similarity is crucial to grasping why prices may fluctuate in erratic but discernible ways.
Fractal theory provides several benefits. First, it enables analysts to better understand market behaviours that appear chaotic at first glance. It also allows for more nuanced risk management strategies, as practitioners can anticipate potential market shifts based on fractal patterns. Furthermore, recognizing fractal elements could lead to improved decision-making in portfolio diversification and algorithmic trading.
In considering the introduction to fractal markets, it is crucial to acknowledge the various dimensions that influence market behaviour, including investor psychology, liquidity dynamics, and information dissemination. These are not just background factors; they interact in complex ways with fractal patterns.
As we delve deeper into this topic, the subsections will sharpen the focus further, leading to a complete comprehension of fractal markets and their significance in today's economic landscape.
Defining Fractal Markets
Defining fractal markets is not merely an academic exercise; it determines how investors perceive risk, predict trends, and structure their trading strategies. A fractal market can be characterized by its self-similarity and complexity across various scales of observation.
The term "fractal" was popularized by mathematician Benoit Mandelbrot, who studied how irregular shapes and patterns recur within larger systems. Applying this to financial markets means recognizing that asset prices may not follow a simple pathway. Instead, they exhibit characteristics that can repeat and evolve, leading to price formations that mimic their historical selves over different time frames.
Fractal markets incorporate elements of chaos theory, which underscores unpredictability but also highlights a certain order amidst the chaos. They encourage market participants to consider how short-term movements can reflect long-term trends.
Historical Context and Evolution
The evolution of fractal market theory dates back several decades. Initially, traditional economic models dominated financial thought. The Efficient Market Hypothesis was widely accepted, positing that all available information is reflected in stock prices. This view, however, faced increasing scrutiny.
In the 1970s and 1980s, Benoit Mandelbrot, alongside other mathematicians and economists, began to articulate an alternative perspective. They introduced the notion that financial markets display fractal characteristics. Mandelbrot's work laid the groundwork for what would later become the field of fractal finance, a critical aspect of understanding market complexity.
The evolution of computational technologies further facilitated this exploration. As data collection methods improved, researchers began to observe and analyze patterns within market data more effectively. They discovered that volatility often clusters and that extreme movements are more common than traditional models suggested.
This historical context is key to appreciating how far the concept of fractal markets has come. It provides insight into contemporary applications within finance and emphasizes the need for an adaptive approach to market analysis.
Theoretical Framework
The theoretical framework is essential in understanding how fractal markets operate. By providing a structured environment within which concepts of fractal theory can be elucidated, readers can appreciate the significance of this framework in financial behavior. A solid theoretical foundation elaborates the interdependent nature of market dynamics, enabling a nuanced exploration of how these principles manifest in practical scenarios.
In grasping these theories, it becomes easier to analyze various market functions, offering insights into both the predictability and unpredictability inherent in financial environments. In this section, we delve into the core components of this framework that form the bedrock for further exploration of fractal markets.
Principles of Fractal Geometry
Fractal geometry is the mathematical underpinning of fractal markets. Unlike traditional Euclidean geometry, fractals define structures that display self-similarity across various scales. This principle holds that similar patterns recur at progressively smaller or larger magnifications. In financial markets, this translates into price movements that display similar patterns over different time frames.
Key principles of fractal geometry include:
- Self-Similarity: Patterns repeat across different scales, making the analysis of financial time series more complex but also more insightful.
- Complexity: Financial markets are not linear; they involve intricate relationships that can be better understood through fractal analysis.
- Scaling Laws: These laws describe how various market behaviorsβsuch as volatilityβexhibit uniform characteristics regardless of the scale of observation.
Understanding these principles is vital for those aiming to model market behavior accurately. They allow researchers and practitioners to better predict market actions based on historical patterns, highlighting the effectiveness of fractal approaches in financial analysis.
Self-Similarity in Financial Markets
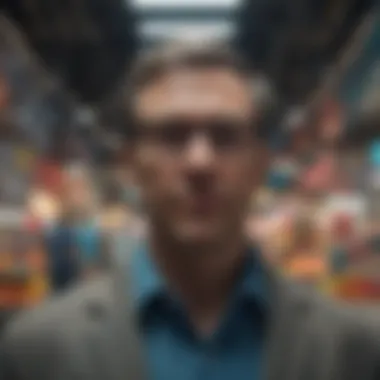
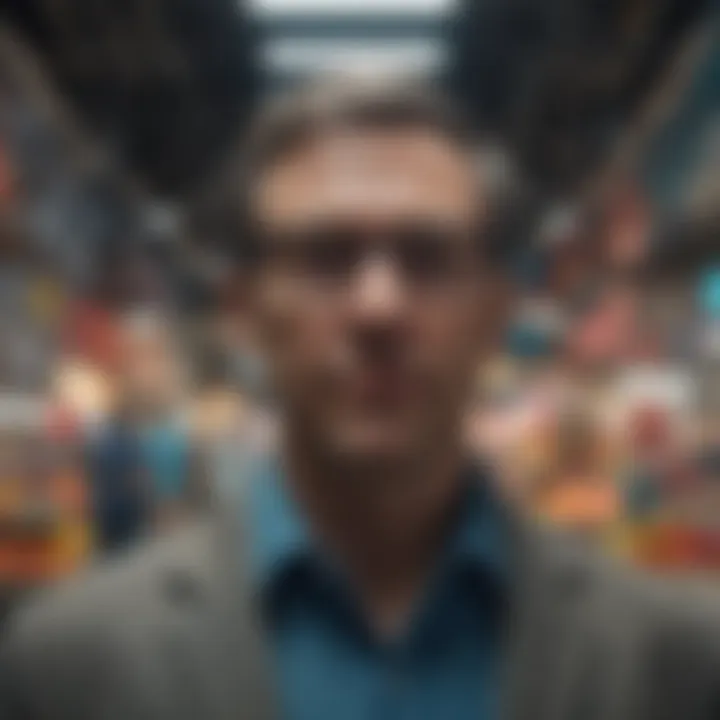
Self-similarity serves as a cornerstone in the analysis of fractal markets. In essence, this aspect indicates that market behaviors follow recognizable patterns, regardless of the observation timeframe. For instance, if one looks at minute-to-minute price movements, fluctuations over hours, or even days, similar trends may emergeβdemonstrating that market dynamics repeat themselves over various lengths.
This property has significant implications for traders and economists. By recognizing these patterns, stakeholders can interpret market indicators more effectively, which can lead to better decision-making. Understanding self-similarity can support the development of strategies that account for historical price movements and predict future trends more reliably.
"The fractal nature of market behavior allows for a more nuanced understanding of economic phenomena, illustrating how local fluctuations can have broader implications across scales."
In summary, exploring self-similarity enhances our comprehension of market structures. It fosters a framework where investors and analysts can appreciate how seemingly random market fluctuations are underpinned by deeper, recurring patterns.
Fractals and Market Dynamics
Fractals and their application in market dynamics provide a transformative perspective on how markets operate. This topic is integral in bridging the understanding between traditional economic theories and modern cognitive insights. Financial markets have often been perceived as linear and efficient, but this does not always capture their inherent complexity. Fractal market dynamics shed light on these complexities, highlighting the role of chaotic behaviors and self-similarity present in market trends.
Market dynamics influence the decision-making processes of traders, investors, and policymakers. By employing fractal analysis, one can better grasp the oscillations and fluctuations in asset prices. Unlike conventional models, which often assume a normal distribution of returns, fractal models recognize a pradoopularity of extreme events. Understanding this behavior leads to more informed strategies regarding risk assessment and investment.
Understanding Market Fluctuations
Market fluctuations are crucial to comprehend, as they signify the very pulse of trading environments. Most traders focus mainly on price movements, often neglecting the underlying patterns that govern these fluctuations. Fractal analysis utilizes the concept of self-similarity, suggesting that market activity appears similar at different scales. For instance, daily price changes can echo patterns observed over longer time frames, such as weeks or months.
This observation influences trading strategies. Traders utilizing fractal theories may identify entry and exit points more accurately. They can anticipate potential reversals or continuations in price trends based on historical patterns that reveal themselves across various time scales. The fractal nature of markets informs us that human behavior and collective trading actions are not random; they often follow recognizable patterns.
Implications for Market Efficiency
The implications of fractals for market efficiency challenge established financial theories. Traditional Efficient Market Hypothesis (EMH) posits that prices reflect all available information. However, fractal models suggest that markets are often influenced by behavioral biases and nonlinear patterns that defy efficiency.
"Market efficiency is not a uniform concept; it varies across different time frames and market conditions."
This idea paves the way for enhanced trading strategies. Investors and analysts can exploit inefficiencies arising from market behavior that fractal geometry brings to light. The ongoing development of risk management techniques based on fractal analysis is particularly highlighting. Such knowledge allows for the construction of robust portfolios that account for extreme fluctuations and non-normal distributions, moving beyond traditional mean-variance optimization.
In summary, the synthesis of fractal analysis in understanding market dynamics offers profound insights. It reshapes how we perceive price movements and market trends, advocating for methods that encompass the chaotic nature of financial systems. By understanding both market fluctuations and their implications for market efficiency, individuals and organizations can develop sharper analytical frameworks that adapt to the complexities of contemporary financial environments.
Modeling Fractal Markets
Modeling fractal markets is a critical aspect of understanding the complexities inherent in financial systems. It offers valuable insights into how markets operate, respond to changes, and how patterns emerge from chaotic phenomena. Effective modeling of fractal markets helps investors, researchers, and policymakers predict market behavior more accurately and develop strategies that align with observed dynamics.
The primary benefit of modeling fractal markets lies in its ability to capture irregularities in market behavior that traditional models often overlook. Markets exhibit self-similar patterns across different time scales, which means that they can behave similarly regardless of whether one is analyzing minute-by-minute fluctuations or yearly performance. Recognizing this self-similarity is essential for creating robust financial models.
When developing models for fractal markets, several specific elements must be considered:
- Understanding Market Structure: Financial markets contain clusters of activity that may shift and change unpredictably. Identifying these clusters helps create more adaptive models.
- Non-Linear Dynamics: Markets often display non-linear behaviors, which means relationships between variables are not always proportionate. This aspect complicates forecasting and necessitates advanced analytical techniques.
- Noise and Volatility: Market data is inherently noisy. Acknowledging the role of noise enables better handling of fluctuations in market data, allowing for a clearer analysis of underlying trends.
Statistical Approaches
To effectively model fractal markets, one may employ various statistical approaches. These techniques allow for the quantification of market fluctuations and help assess the degree of chaotic behavior present in financial data. Here are some common statistical tools:
- Hurst Exponent: This metric measures the long-term memory of time series data, indicating whether trends are persistent or mean-reverting. A Hurst exponent above 0.5 suggests a trending market, while below 0.5 indicates mean-reversion.
- Fractal Dimension: This statistical measure describes how detail in a pattern changes with the scale at which it is measured. A higher fractal dimension indicates a more complex market structure.
- Time-Series Analysis: Techniques such as ARIMA (AutoRegressive Integrated Moving Average) and GARCH (Generalized Autoregressive Conditional Heteroskedasticity) models can be adapted to account for fractal behavior in market data.
Adoption of these statistical tools enables researchers to build and validate assumptions about market movements and the potential for returns, thereby providing a more nuanced view of risk and opportunity.
Application of Chaos Theory
Chaos theory is another key component in the modeling of fractal markets. It emphasizes the sensitivity of systems to initial conditions, where small changes can lead to significant consequences in market outcomes. This aspect of chaos theory provides profound implications for financial modelling.
Key applications of chaos theory in fractal markets include:
- Risk Assessment: By understanding the chaotic nature of markets, investors can better gauge the risks associated with various investment strategies and the underlying market conditions.
- Predictive Modelling: Incorporating chaos theory allows for the development of models that can anticipate sudden shifts or trends in the market that traditional forecasting may not predict accurately.
- Systematic Trading: Tailoring trading strategies based on insights gathered from chaos theory can lead to better responses to market movements, making trading more effective.
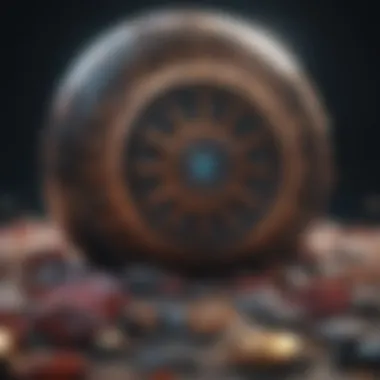
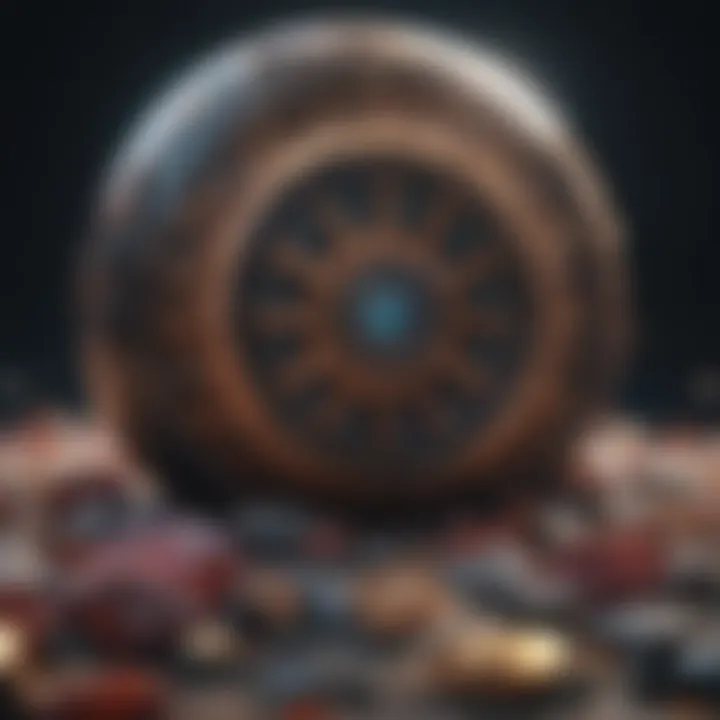
In summary, modeling fractal markets through statistical approaches and chaos theory enhances our comprehension of market dynamics. This understanding can lead to more informed decisions by reflecting the real-world complexities of financial systems. By analyzing and predicting these markets with fractal theories, investors and researchers can achieve a competitive edge.
Empirical Evidence
Empirical evidence plays a crucial role in substantiating the principles of fractal markets. This evidence helps bridge the gap between theoretical constructs and real-world financial phenomena. By analyzing actual market data and behavior, researchers can validate or challenge the assumptions made by fractal market theory. This evaluation allows for a deeper understanding of market dynamics, leading to better forecasting and modeling.
Key elements of empirical evidence in this context include:
- Validation of Theoretical Constructs: Empirical studies can affirm or refute existing theories, ensuring that they align with observed behaviors.
- Case Analysis: Real-world case studies provide concrete examples of how fractal behavior manifests in various markets.
- Longitudinal Studies: Ongoing data collection over extended periods yields insights into market trends and shifts.
Overall, empirical evidence is essential for refining fractal market theory, adapting it to evolving market conditions, and enhancing its practical applicability.
Case Studies in Financial Markets
Case studies serve as a powerful method to illustrate the application of fractal market theory in actual financial settings. They offer nuanced perspectives on how market participants react to volatility and change. One notable case is the analysis of the U.S. stock market during periods of economic upheaval.
In such cases, patterns of self-similarity can often be observed. For instance, investors display similar behavioral responses during both minor fluctuations and severe crashes. These patterns can be identified through various indicators, including price movements and trading volumes. The significant volatility during the financial crisis of 2008 has often been analyzed through this lens, revealing fractal characteristics in market responses.
Additionally, currency markets provide ample data for case studies. The foreign exchange market, with its high liquidity and rapid trading, often highlights fractal patterns. Analyzing these cases equips researchers with critical insights into how fractal structures influence trading strategies and investor decisions.
Longitudinal Studies and Data Analysis
Longitudinal studies are vital in understanding how market characteristics evolve over time. By examining data over extended horizons, researchers can detect patterns that are not apparent from short-term observations. These studies can provide insight into recursive behaviors and the persistence of fractal properties in financial markets.
For example, analyzing data from indices like the S&P 500 over several decades can illustrate the changing nature of market cycles. Researchers may observe that while short-term dynamics are erratic, longer-term trends often reveal a fractal structure marked by self-similar behavior across different time frames.
Data analysis techniques such as wavelet transforms and Hurst exponent calculations are employed to identify these fractal characteristics rigorously. These techniques facilitate the breakdown of market data into manageable segments, allowing for a detailed assessment of self-similarity and multifractality.
Critiques of Fractal Market Theory
Critiques of fractal market theory play a crucial role in understanding its limitations and potential. By examining these critiques, one can grasp the complexities and any gaps present in its application to financial markets. Although the fractal market hypothesis provides valuable insights, it is essential to critically assess its claims and consequences. This section will cover two vital aspects: limitations and challenges, as well as alternative perspectives that challenge this theory.
Limitations and Challenges
Fractal market theory, despite its innovative approach to modeling market dynamics, has notable limitations. Some of these limitations include:
- Empirical Verification: One of the most significant challenges is the difficulty in empirically validating the theory. While self-similarity and fractal patterns can be visually recognized in financial data, establishing rigorous statistical evidence is often complicated.
- Complexity of Models: The fractal models can be highly intricate, making them difficult for practitioners to apply. Many finance professionals may find that they require extensive training to fully understand these models and their implications.
- Market Non-Linearity: The theory assumes that market behavior is inherently fractal. However, real-world market movements can be influenced by numerous unpredictable factors. Major economic events, geopolitical tensions, or sudden shifts in investor sentiment can disrupt fractal patterns.
- Behavioral Factors: Fractal markets often overlook the influence of irrational behavior among investors. Emotional factors frequently drive market decisions, complicating predictions based solely on mathematical models. Would that be considered in a truly fractal market?
These limitations contribute to skepticism regarding the universality of fractal market theory. Nevertheless, awareness of these challenges allows researchers and practitioners to approach the concept with a balanced perspective.
Alternative Perspectives
Alternative perspectives provide a fresh lens through which one can view and critique fractal market theory. Some notable perspectives include:
- Efficient Market Hypothesis: This classical theory posits that markets are efficient, with all available information reflected in asset prices. In contrast to the fractal perspective, which accepts market inefficiencies, the efficient market hypothesis suggests that systematic errors in pricing cannot persist.
- Behavioral Finance: Behavioral finance emphasizes how psychological factors and cognitive biases affect market outcomes. This perspective often contrasts with the rational underpinnings of fractal markets, questioning whether investor behavior can be accurately modeled mathematically.
- Agent-Based Models: These models simulate the actions of individual agents to understand market behavior. They often reveal emergent phenomena that may not align neatly with fractal predictions, as they account for the heterogeneous behaviors of different market participants.
- Alternative Theories: Other theories such as Data-Driven Models also question the assumptions made in fractal markets regarding predictability and structure. These alternatives often emphasize the use of vast datasets and machine learning techniques to identify patterns without adhering strictly to fractal principles.
The diversity of critique illustrates that while fractal market theory offers unique insights into financial behavior, it faces significant opposition. This discourse enriches the overall understanding of market dynamics, encouraging further research and development in financial theories.
Applications of Fractal Market Theory
The applications of fractal market theory are significant in financial analysis and have practical uses that can influence investment strategies. This theory allows a deeper understanding of market behaviors, especially during turbulent times. Financial professionals can apply fractal concepts to enhance risk management, optimize algorithmic trading, and improve portfolio diversification. It gives insights into how market participants behave, providing a framework to analyze complex market patterns.
Risk Management Strategies
Risk management remains a cornerstone of effective financial practice. In the context of fractal market theory, understanding the self-similar nature of market movements allows for more refined risk assessments. For instance, risk metrics can be adjusted based on historical patterns that showcase fractal behavior.
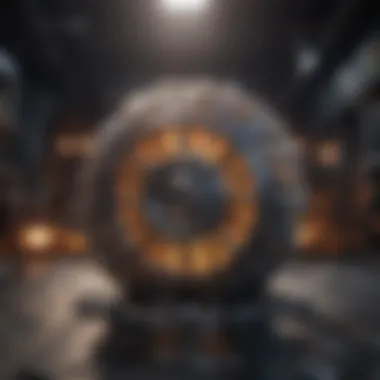
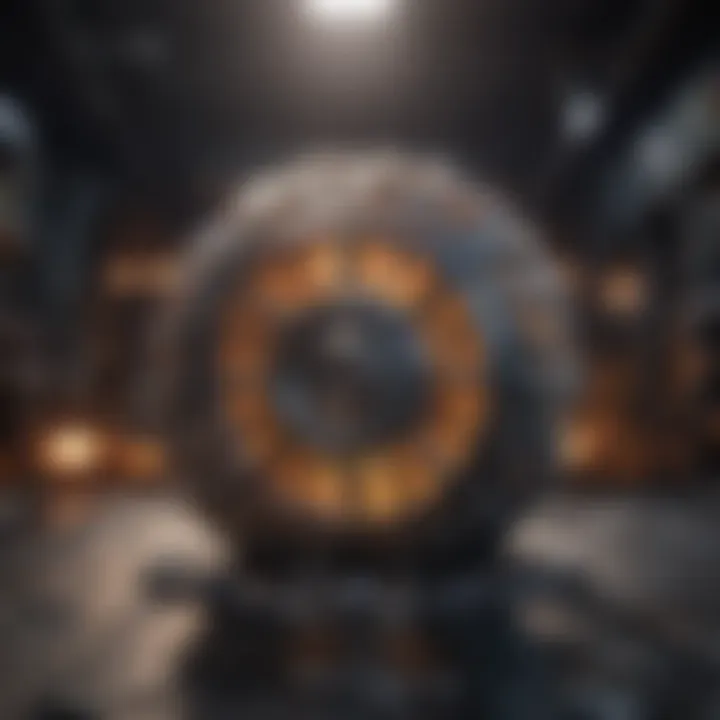
- Identifying Patterns: Fractal analysis can help in identifying recurring patterns in market data. These patterns can serve as indicators for risk levels, which enables traders to take preemptive actions.
- Dynamic Risk Models: By incorporating fractal principles, risk models can become more dynamic, adapting to volatility in real-time rather than relying on static historical data.
- Tail Risk: Understanding that extreme events are more common in fractal markets aids in preparing for tail risks, which are critical for long-term success.
In essence, integrating fractal analysis into risk management strategies allows for a more nuanced approach, ultimately resulting in better protective measures against unforeseen market shocks.
Algorithmic Trading
Algorithmic trading refers to the use of computer algorithms to execute trading orders. Fractal market theory provides valuable insights into market dynamics that can enhance algorithmic strategies.
- Precision in Execution: Algorithms can be designed to recognize fractal patterns, ensuring trades are executed with greater precision during different market conditions.
- Adaptive Strategies: Algorithms that utilize fractal analysis can adapt to changes in market behavior, allowing for real-time adjustments. This adaptability can lead to improved profitability.
- Risk-Adjusted Returns: By considering fractal characteristics, traders can create algorithms that focus on achieving risk-adjusted returns, optimizing overall performance.
This application of fractal market theory can transform the efficiency of trading and lead to more sustainable trading practices.
Portfolio Diversification
Portfolio diversification is essential for mitigating risk and optimizing returns. Fractal market theory informs how assets behave in different market conditions, guiding effective diversification strategies.
- Asset Selection: Insights gleaned from fractal analysis assist in selecting assets that are likely to behave differently in various market scenarios, promoting true diversification.
- Correlation Insights: Fractal behavior often reveals unexpected correlations between assets. Recognizing these correlations helps in developing a more resilient portfolio.
- Dynamic Rebalancing: The principles of fractals can lead to strategies for dynamic rebalancing of portfolios, allowing investors to take advantage of shifts in market trends.
Thus, by leveraging fractal concepts, investors can achieve a balanced portfolio that is less susceptible to market volatility, ultimately driving better long-term results.
The Future of Fractal Market Theory
The future of fractal market theory stands at a compelling intersection of finance and mathematics. Its advances promise to reshape our understanding of market behaviors and dynamics. As financial markets continue to evolve in response to technological innovation and globalization, the application of fractal theory offers new insights into these complex systems. This exploration looks to address the potential developments in theoretical frameworks and practical applications that fractal market theory offers.
Innovations in Financial Theory
Fractal market theory highlights certain innovative aspects in its approach to financial theory. One of the most significant innovations is the recognition of non-linear behavior in markets. Traditional financial theories often assume a linear relationship between risk and returns, which may not accurately capture the erratic nature of real-world market movements. In contrast, fractal theory acknowledges multiple scales of time and volatility. This encourages a more tailored approach to market analysis and risk assessment.
Moreover, practitioners are now leveraging advanced computational techniques to analyze fractal patterns within market data. These innovations lead to more accurate forecasting models that account for the chaotic nature of market fluctuations. For example, machine learning algorithms applied to historical price data can uncover hidden fractal patterns, revolutionizing how financial predictions are made.
Some potential innovations include:
- Enhanced Predictive Models: Utilizing fractal analysis for improved stock price forecasting.
- Complexity Theory Applications: Integrating insights from chaos and complexity theory into financial decision-making.
- Behavioral Finance Integration: Acknowledging human behavior in market dynamics as another layer of complexity.
Integration with Other Financial Models
Integration of fractal market theory with other financial models presents an opportunity for comprehensive market analysis. The complexities of modern financial systems demand an interdisciplinary approach. By uniting fractal concepts with established models, such as the Efficient Market Hypothesis and Behavioral Finance, a more robust understanding of market phenomena emerges.
For instance, combining fractal theory with behavioral finance may provide insights into how irrational behaviors can generate market anomalies. Similarly, integrating fractal analysis with risk management frameworks can enhance the ability to predict and mitigate potential financial crises. This holistic view ensures that market participants can better navigate uncertainties.
Key considerations for integration include:
- Synthesizing Existing Theories: Merging various theories to create comprehensive models that better reflect market realities.
- Creating New Analytical Tools: Developing tools that harness insights from both fractals and traditional finance, potentially yielding new methodologies.
- Collaborative Research Initiatives: Encouraging partnerships between mathematicians, economists, and psychologists to amplify the practical applications of fractal markets.
"The continued evolution of fractal market theory has the potential to redefine our approach to financial markets, offering deeper insights and more effective tools for analysis."
End
The conclusion section serves a critical role in encapsulating the insights gained throughout the exploration of fractal markets. It allows the reader to reflect on the complexities discussed and to appreciate the broader implications of fractal theory in financial contexts.
Summary of Key Insights
Fractal market theory provides a nuanced understanding of financial phenomena. Key insights from the article include:
- Self-Similarity: Market behavior exhibits self-similarity, suggesting that patterns of price movements on smaller time scales replicate larger trends.
- Market Dynamics: Traditional models often fall short when accounting for the complexities of market fluctuations, a gap that fractal analysis seeks to address.
- Risk Management: Understanding the fractal nature of markets allows for improved risk management strategies, enabling traders and investors to make informed decisions.
- Future Integration: As financial models evolve, integrating fractal theories with other methodologies may yield more comprehensive frameworks for understanding market behavior.
The significance of these insights cannot be overstated. They challenge conventional thinking about market efficiency and provide a framework for addressing real-world financial challenges.
Final Thoughts on Fractal Markets
The exploration of fractal markets reveals the intricate web of relationships that underlie financial systems. By appreciating the fractal nature of markets, investors, analysts, and academics can gain deeper insights into market behaviors. The path forward should involve further research and a commitment to integrating fractal theory with existing financial models.