Exploring the Dynamics of the Black Box
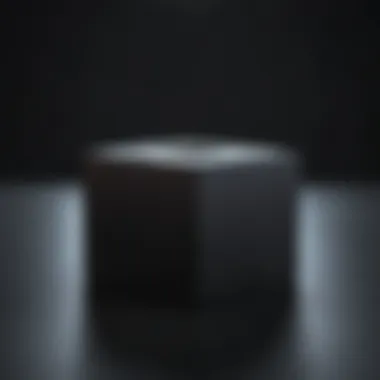
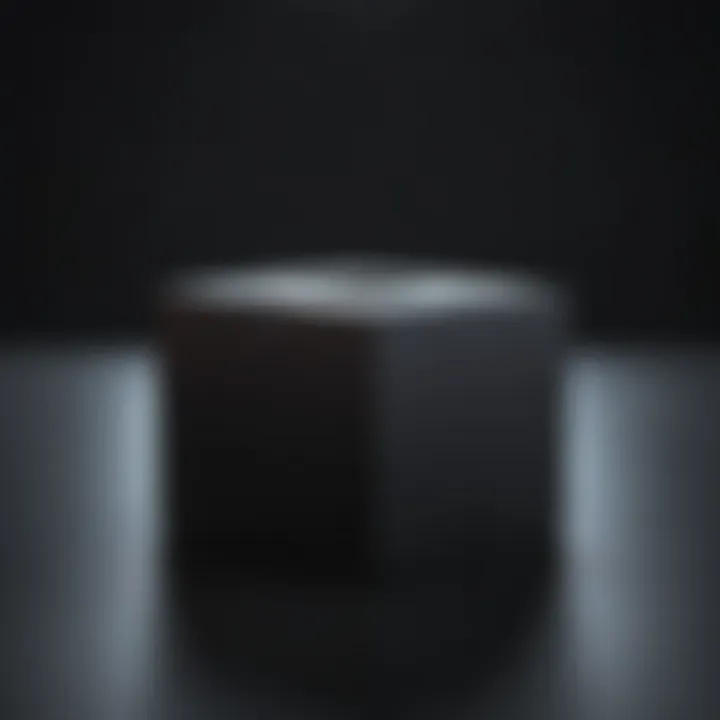
Intro
The concept of the black box is ubiquitous in scientific discussions today. Defined broadly, it refers to any system whose internal workings are not accessible or understandable from the outside. This characteristic leads to significant implications in various fields such as analytics, machine learning, and theoretical models.
Researchers encounter black boxes as they analyze vast amounts of data or implement artificial intelligence systems. The power of these systems lies in their ability to process data efficiently, often leading to insightful conclusions. However, the opaque nature of these systems raises critical questions about the reliability of their outputs. How can stakeholdersβscientists, policymakers, and the general publicβtrust the conclusions drawn from these systems without understanding their inner mechanisms?
This article seeks to illuminate the perspectives surrounding black boxes, addressing both their advantages and limitations. It provides a detailed exploration of their implications for research, decision-making, and accountability, all while considering the ethical dimensions of our reliance on such systems.
Through this discourse, readers will gain a deeper understanding of the complexities involved in interpreting the outputs of black boxes. The need for critical engagement with these systems cannot be overstated, especially as reliance on them continues to grow across various domains.
Understanding these dynamics is not only relevant for students and educators, but also crucial for professionals who navigate the ethical landscape shaped by black boxes. By analyzing the intricate interplay between transparency and accountability, we aim to foster a comprehensive dialogue on these topical issues.
Understanding the Concept of the Black Box
The concept of the black box is essential to grasp when analyzing its applications across various fields. A black box represents systems or processes whose internal workings are not fully transparent or understood. This opacity presents unique challenges and advantages, particularly in fields such as machine learning, engineering, and social sciences.
Understanding the black box can lead to more effective solutions in problems where intricate processes are involved, ultimately enhancing both research and practical applications. By dissecting this concept, researchers and practitioners can navigate the complexities of systems that rely heavily on algorithms and predictive models.
Definition and Origins
The term "black box" originated from engineering and computing disciplines, describing a device or system that can be viewed solely in terms of its input-output behavior. Essentially, whatever happens inside the black box remains hidden from the user. This characteristic leads to significant implications in various sectors. The origins of the term can be traced back to the early 20th century, linking it primarily to electrical engineering, where it described complex electronic systems. Today, its relevance has expanded into many disciplines, specifically in data analysis and artificial intelligence.
Black boxes, especially in machine learning, often employ algorithms that operate on large datasets. Users input data into the system, and the algorithms produce outputs or predictions. The specific methodologies, however, are not always disclosed. This lack of clarity can deter users from trusting the results, especially if the applications affect critical domains such as healthcare and finance.
Relevance Across Disciplines
The relevance of black boxes spans numerous disciplines, each applying the concept to unique issues and challenges. For instance, in economics, black boxes can represent market predictions based on unseen variables. Similarly, in biology, a black box may describe complex biological systems where inputs and outputs can be measured, but the internal processes remain obscure.
In the realm of computer science, black boxes are fundamental to machine learning models, where the determination of outcomes relies on vast amounts of data without revealing the intricate details of the underlying algorithms. This can be beneficial in that it allows for rapid advancements and practical applications, but it also raises questions around accountability and transparency.
Furthermore, in the context of decision-making, black boxes can affect policy formulation. When policymakers rely on black box analyses, they might inadvertently overlook crucial biases or inaccuracies. This concern emphasizes the necessity for a comprehensive understanding of black boxes to ensure that their use benefits society as a whole.
Individuals and organizations must critically engage with the dynamics of black boxes. This engagement will not only lead to more informed decision-making but also foster the development of systems that prioritize transparency and ethical considerations.
The exploration of black boxes goes beyond mere analysis; it delves into the ethical implications and accountability in modern technology.
The Role of Black Boxes in Machine Learning
Machine learning systems often function as black boxes. This term indicates how these models can produce outcomes without showing how they arrive at those decisions. The significance of understanding this black box nature is crucial for several reasons. It addresses not only the innovative aspects of machine learning but also the ethical considerations and implications for real-world applications.
Algorithmic Foundations
At the heart of machine learning lies algorithms that process data. These algorithms can range from simple linear models to complex neural networks. Each has its own underlying structures which determine how inputs translate into outputs.
The choice of algorithm impacts both the accuracy and interpretability of results. For instance, decision trees provide a more transparent view of decision-making, while deep learning networks can achieve higher accuracy but lack clarity. The balance between performance and explainability needs careful consideration.
Implications for Predictive Analytics
Black box methods in machine learning significantly enhance predictive analytics. They help in fields like finance, healthcare, and marketing by providing predictions based on data patterns. These predictive tools can uncover insights that might be missed by traditional analytical methods.
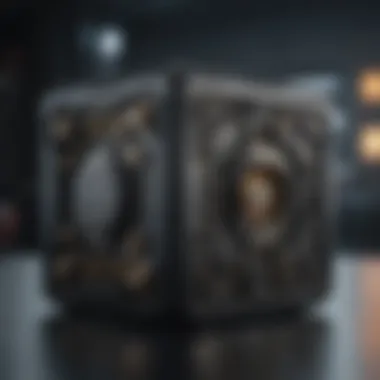
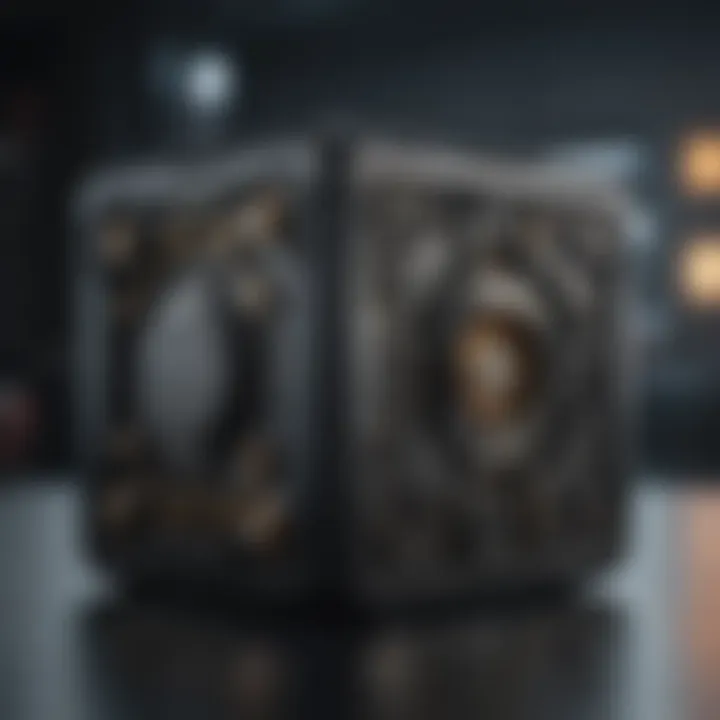
However, the opaque nature of certain algorithms can limit trust. Stakeholders often question the reliability of the output. Thus, ensuring that predictions are accurate while also being interpreted correctly is essential for their acceptance in decision-making contexts.
Challenges in Interpretation
Understanding how black box algorithms operate presents notable challenges.
Overfitting and Generalization
Overfitting occurs when a model learns the noise in the training data rather than the actual signal. This means that while the model may perform well on training data, it generally fails on unseen data sets. This characteristic makes overfitting crucial to analyze when discussing machine learning's reliability.
In practical scenarios, models must generalize well to be useful. Hence, researchers seek methods to balance fitting with generalizability. Techniques such as cross-validation and regularization can mitigate overfitting. Still, identifying the right degree of complexity becomes essential to ensure robust performance.
Lack of Transparency
The lack of transparency in black box models raises further issues. Users often have no clear insight into how decisions are made. This ambiguity can be problematic in critical applications, such as healthcare, where the justification of decisions is necessary.
The core of this issue lies in the complexity of the algorithms and their intricate internal processes. The lack of transparency can lead to mistrust among users and stakeholders, thereby limiting the broad acceptance of machine learning applications. Addressing this challenge requires ongoing discussion and innovation around the creation of interpretable models.
Black Box Systems in Theoretical Models
Black box systems in theoretical models present a nuanced intersection of observation and abstraction. Understanding these systems is essential for unraveling complex phenomena across various scientific disciplines. They allow researchers to explore relationships and outcomes without directly revealing the internal mechanics of the models employed. This aspect simplifies analysis but also raises critical questions about the potential for misinterpretation and over-reliance on opaque systems.
Applications in Physics
The application of black box systems in physics provides a powerful way to model and predict behavior in complex systems, particularly in areas like quantum mechanics and thermodynamics. Researchers leverage these models when straightforward analytical solutions are unattainable. In many scenarios, physicists utilize mathematical abstractions to represent systems while only focusing on observable outcomes.
For instance, in particle physics, the behavior of subatomic particles can be modeled using black box approaches. The intricate interactions among particles lead to outcomes that can be measured, yet the underlying processes remain difficult to observe directly. This method not only aids in predicting results of experiments but also facilitates exploration of concepts like time dilation and quantum entanglement, where conventional interpretations fall short.
- Advantages:
- Considerations:
- Simplifies complex problem solving.
- Facilitates effective communication of concepts in abstract environments.
- Helps in pattern recognition in chaotic systems.
- Risks of oversimplification.
- Potential loss of detailed understanding of system dynamics.
Biological Implications
In the realm of biology, black box systems play a significant role in modeling intricate life processes and ecosystems. For example, they are frequently used in ecological studies to simulate interactions within populations, examining how changes in one species impact others within the ecosystem. The use of black box models here enables researchers to develop predictions about behavior based on measurable data without requiring exhaustive knowledge of all factors involved.
The complexity of biological systems means that a vast number of variables must be considered. Black box models help to identify important correlations that might not be immediately apparent through direct observation. Besides, these models are beneficial in medical research, where certian diseases or conditions may not have straightforward etiologies. Predictive models can be developed to estimate outcomes based on symptoms or treatments, thereby assisting in clinical decision-making.
- Key Benefits:
- Challenges:
- Enables focusing on critical biological interactions without overwhelming complexity.
- Assists in large-scale data analysis, especially in genomics and proteomics.
- Can mask important biological mechanisms.
- Misinterpretations can lead to ineffective or harmful interventions.
Black boxes in theoretical models are essential tools, but their limitations must be considered to ensure valid conclusions and ethical applications.
Black Boxes and Decision Making
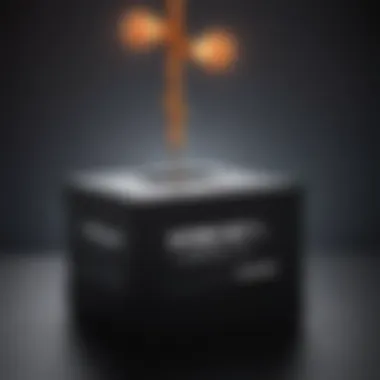
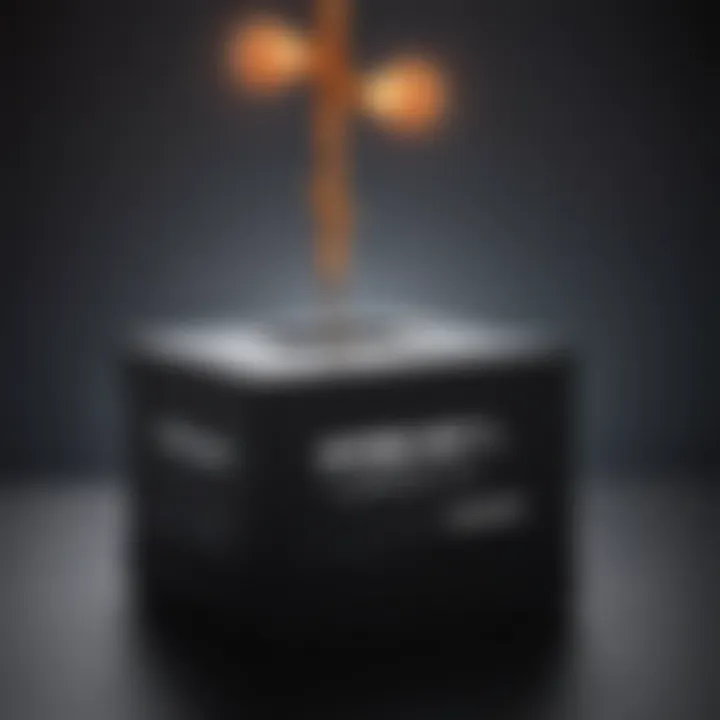
The interplay between black boxes and decision making is crucial in understanding their widespread implications. Black boxes, often representing complex algorithms, shape outcomes in policy, healthcare, finance, and more. The outputs from these systems, though opaque, can significantly influence strategic choices.
Understanding how these outputs are utilized is essential. The reliance on black boxes can streamline processes and enhance efficiency, but it also raises concerns about accountability, fairness, and transparency. It is important to navigate the benefits and challenges tied to these systems to make informed decisions.
Utilizing Outputs in Policy Formulation
The outputs generated by black box systems can inform policy decisions in numerous ways. First, these systems offer data-driven insights, allowing policymakers to base decisions on empirical evidence rather than intuition alone. For instance, in urban planning, predicting traffic patterns through AI algorithms can enhance public transport systems. This predictive power allows for dynamic adjustments to policies, enhancing urban infrastructure, and potentially improving citizens' quality of life.
However, the application of these outputs in real-world scenarios requires careful consideration. The data used within black boxes may reflect historical biases, which, when left unchecked, could perpetuate inequities. To use outputs effectively, policymakers must critically analyze the context and datasets feeding into these models.
Furthermore, different stakeholders might interpret the outputs differently. This multiplicity of interpretations necessitates collaborative dialogues among experts and the public to establish the best course of action based on black box outputs. Striking this balance is central to effective policy formulation that acknowledges the limitations of opaque systems while still embracing their potential benefits.
Impacts on Accountability
The reliance on black boxes in decision-making frameworks introduces a complex layer to accountability. When decisions are made based on outputs from an algorithm, it can obscure the rationale behind those decisions. This lack of transparency complicates the ability to assign responsibility when outcomes are unfavorable. For example, in the context of judicial or hiring decisions powered by algorithms, it may be unclear how certain criteria influenced the final verdict.
The challenge of accountability does not lie solely in the algorithms themselves; human oversight is equally essential. Organizations must foster an environment where the processes related to black boxes are scrutinized, and outputs are validated against ethical standards. This can involve using additional tools that enhance transparency or establishing clear protocols for reviewing decisions made with the help of black box systems.
βThe opacity of some decision-making algorithms can erode public trust, necessitating the demand for more interpretable models.β
The Ethical Considerations of Black Boxes
The ethical implications surrounding black boxes are increasingly relevant in today's data-driven world. As black box systems become more prevalent in diverse fields such as machine learning, healthcare, and finance, understanding their ethical dimensions is essential. Ethical considerations primarily focus on issues like equity, transparency, accountability, and bias. Each of these elements plays a vital role in shaping the societal impact of black box systems. Ignoring these ethical factors can lead to significant inequalities and deeply rooted biases. Therefore, it is critical to engage with these discussions when analyzing black box technology and its applications.
Equity and Bias in Algorithm Design
Equity and bias in algorithm design are significant concerns when discussing black boxes. Many algorithms use vast datasets that may inadvertently reflect existing societal biases. For instance, facial recognition technologies have been known to exhibit higher error rates for minority groups. This discrepancy can be attributed to the datasets used for training, which often lack diverse representation. As such, the decisions made by these algorithms can perpetuate existing inequalities.
Bias in algorithm design arises not only from data selection but also from the design choices made by developers. These biases can be unintentional, yet they can have profound implications. The consequences may result in unfair treatment of certain groups in critical applications such as hiring, lending, and law enforcement. It is therefore essential for data scientists and developers to scrutinize their models and datasets carefully. Discussions around bias also raise questions about accountability. Who is responsible when a black box system leads to discriminatory outcomes? This uncertainty complicates the ethical landscape around algorithmic decision-making.
Societal Implications
The societal implications of black boxes extend far beyond the technical realm. A profound reliance on these systems raises questions regarding trust and reliance on technology. Citizens often feel the effects of these algorithms in their day-to-day lives without understanding how decisions are made. This lack of transparency can foster distrust in institutions that implement black box systems. If individuals cannot comprehend how their data is used to inform decisions, they may feel alienated.
Moreover, the interaction between technology and society invites scrutiny regarding its broader implications on democracy and civil rights. Black box systems can influence important areas, including public policy and law enforcement practices. As these systems become more integrated into critical decision-making processes, ensuring their ethical deployment becomes increasingly urgent.
Advancements in Interpretable Models
The significance of advancements in interpretable models has gained much traction, particularly in the wake of increasing reliance on complex black box systems. These models strive to balance predictive power with an essential quality: interpretability. As data-driven decisions become more integral in various fields, understanding how these systems generate their outputs becomes crucial. The relevance of interpretable models cannot be overstatedβclarity in these processes enhances trust, accountability, and ultimately, effective decision-making.
Techniques for Demystifying Outputs
Several techniques are employed to enhance the interpretability of black boxes. These methods can transform previously inscrutable outputs into clear insights. Some common techniques include:
- Model Distillation: Simplifying complex models into easier-to-understand forms while retaining essential characteristics. This allows for performance comparable to original models but with improved transparency.
- LIME (Local Interpretable Model-Agnostic Explanations): A technique that explains individual predictions by approximating the black box with a simpler model in the vicinity of the instance being scrutinized. This provides local insights about why certain predictions were made.
- SHAP (SHapley Additive exPlanations): An approach based on cooperative game theory. It quantifies the contribution of each feature to a prediction, thus enabling users to understand the influence of different inputs.
- Visualization Tools: These tools help in representing complex data and model decisions visually. Visualization can range from feature importance plots to decision trees, offering an intuitive understanding of the model's processes.
"The benefits of interpretable models extend beyond just understanding; they promote confidence in algorithmic decision-making processes, fostering an environment conducive to ethical practices."
By adopting these techniques, researchers and practitioners can make strides toward unveiling the opaque nature of black box systems. Each method presents its advantages and unique trade-offs, indicating that choices in technique often depend on specific contexts and requirements.
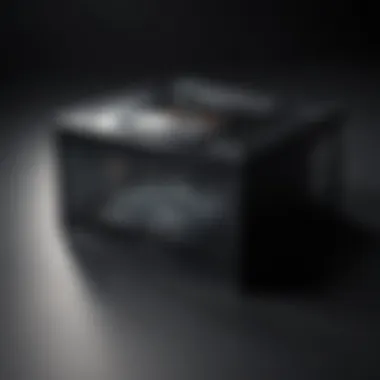
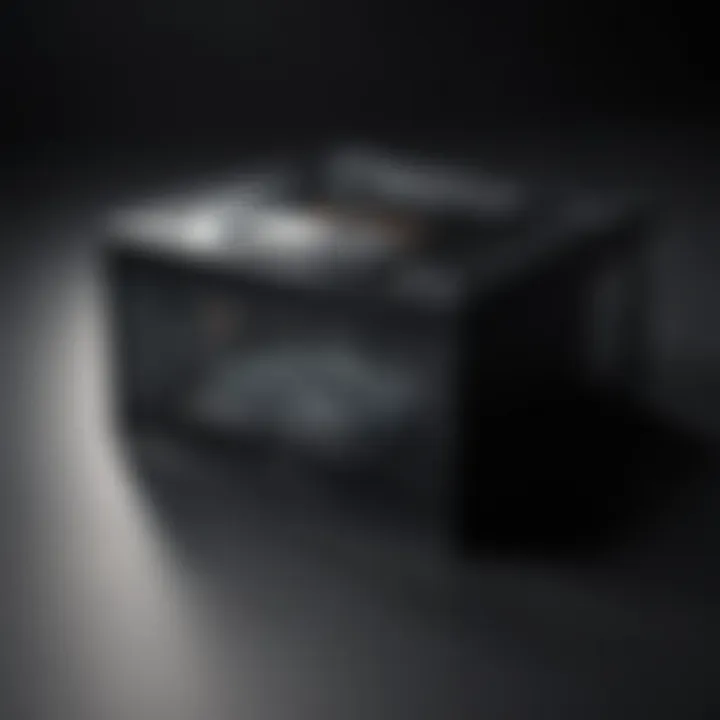
Case Studies in Transparency
There are numerous case studies that exemplify the successful application of interpretable models. These examples highlight the effects of prioritizing transparency within various domains.
Healthcare: Interpretability becomes critical when AI systems assist in diagnosing conditions. For instance, a study on using interpretable models in predicting heart disease allowed clinicians not just to view results but to comprehend the reasoning behind them, improving patient care and trust in AI-assisted decisions.
Finance: In risk assessment for loans, companies such as ZestFinance leverage interpretable algorithms to understand credit risks better. The models not only provide predictions but also comprehensive insights into the assessment factors, ensuring compliance with regulations and fostering consumer trust.
Autonomous Vehicles: Companies working on self-driving technology, such as Waymo, advocate for interpretable models to ensure safety. These vehicles must provide transparent reasoning for their choices while navigating, allowing engineers to understand and rectify any anomalous behaviors.
Advancements in interpretable models signify a promising shift in how we handle complexity in algorithms. This approach fosters a dialog between users and machines, yielding systems that are both powerful in their calculations and understandable in their logic. As technology continues to evolve, the interplay between sophistication and interpretability will become paramount.
Future Trends in Black Box Research
The study of black box systems is at a pivotal stage, where emerging technologies usher in new capabilities but also introduce unforeseen complexities. Recognizing the trends in black box research is essential, as these advancements directly influence analytics, engineering, and decision-making processes across various domains. This section addresses the technological innovations on the horizon and the possible obstacles that researchers and practitioners may encounter.
Emerging Technologies
Recent advancements in artificial intelligence and machine learning have sparked the development of tools aimed at interpreting black box models. Technologies such as interpretable machine learning, explainable AI (XAI), and advanced visualization techniques are becoming increasingly prevalent. The use of natural language processing for model interpretation enhances communication between models and users, allowing non-technical stakeholders to engage meaningfully with predictive analytics.
- Interpretable Models: Researchers are continuously designing models that maintain predictive power while being transparent. Such models mitigate the risks of reliance on opaque systems by ensuring outputs are easier to understand and validate.
- Explainable AI: XAI methods, such as LIME (Local Interpretable Model-agnostic Explanations) or SHAP (SHapley Additive exPlanations), provide insights into why a model makes certain decisions. This helps to demystify predictions and supports ethical responsible use.
- Advanced Visualization Techniques: As datasets grow in complexity, visualization tools must evolve. Technologies enabling interactive and multi-dimensional representations are making it easier to explore model behavior and outputs visually.
These innovations hold great promise for improving transparency and fostering trust among users. However, the implementation of these technologies requires careful consideration to avoid merely masking the black box problem with superficial solutions.
Anticipated Challenges
As the landscape of black box systems evolves, several challenges remain pertinent. Addressing these can significantly impact the practicality of emerging technologies.
- Maintaining Accuracy vs. Interpretability: A persistent balance must be struck between the accuracy of predictive models and their interpretability. While more transparent models may sacrifice some predictive power, it is crucial to ensure that simplifying explanations do not lead to misinterpretation.
- Scalability Issues: As models grow larger and more complex, existing interpretability techniques may struggle to scale effectively. Developing robust solutions that remain useful across varied applications will be critical.
- Regulatory and Ethical Concerns: With increased scrutiny on algorithmic decisions comes the challenge of ensuring compliance with regulations that govern fairness and accountability. Developers must anticipate regulatory requirements and societal expectations surrounding algorithmic transparency.
The future of black box research presents both opportunities and hurdles. Proactively addressing these challenges will shape the integration of emerging technologies in society, ultimately determining how these models can be employed responsibly.
In summary, the future trends in black box research signify both a promising evolution of tools and methods, as well as a call to navigate complexities with caution and rigor. Engaging with these trends will help address the inherent limitations of black boxes, making strides toward a clearer understanding of their role in analytics and decision-making.
Concluding Thoughts
The topic of 'Concluding Thoughts' is crucial in this article as it serves to distill and summarize the varied insights presented about black boxes. In a landscape where opaque systems increasingly influence decisions in numerous fields, understanding the implications of these mechanisms becomes essential. The conclusion synthesizes key findings, offering a reflective overview that ties back to earlier discussions about black boxes in machine learning, theoretical models, and ethical considerations.
Synthesis of Insights
Black boxes play a pivotal role in numerous disciplines, demonstrating both benefits and challenges. They provide a means to process vast amounts of data, enabling predictive analytics and informed decision-making. However, their opacity can lead to significant issues, such as the lack of accountability and potential biases in algorithms.
One key insight is that the complexity of black boxes cannot be ignored. As systems grow more intricate, the need for clear interpretation and transparency increases. Understanding how these models function is essential for improving their usability and ethics.
- Key Takeaways:
- Black boxes influence various fields including machine learning and policy formulation.
- The balance between innovation and ethical responsibility is delicate.
- Critical engagement is necessary for the advancement of transparent systems.
"The efficacy of our reliance on black boxes hinges on our ability to understand their dynamics and implications."
Call for Critical Engagement
As we conclude, there is a strong call for critical engagement with black box systems. Researchers, educators, and practitioners should develop a nuanced understanding of these constructs, moving beyond passive acceptance.
Discussions around transparency, accountability, and ethics must remain at the forefront of any evaluation of black boxes. Moreover, a collaborative effort among stakeholders is vital in addressing these challenges.
- Areas for Engagement:
- Development of frameworks for transparency in algorithm design.
- Ongoing training and education about the implications of black box systems.
- Encouraging interdisciplinary collaboration to tackle black box issues.
In light of this, a future-oriented perspective is necessary. Engaging critically is not just about identifying flaws; it is also about harnessing the potential of these systems while ensuring fairness and equity in their application.