Data Interoperability Standards Explained
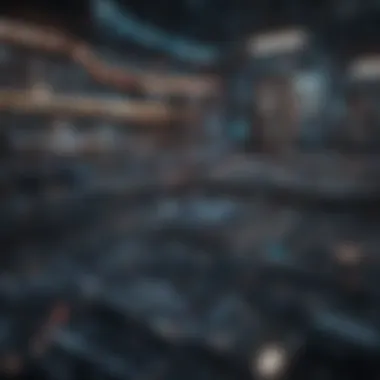
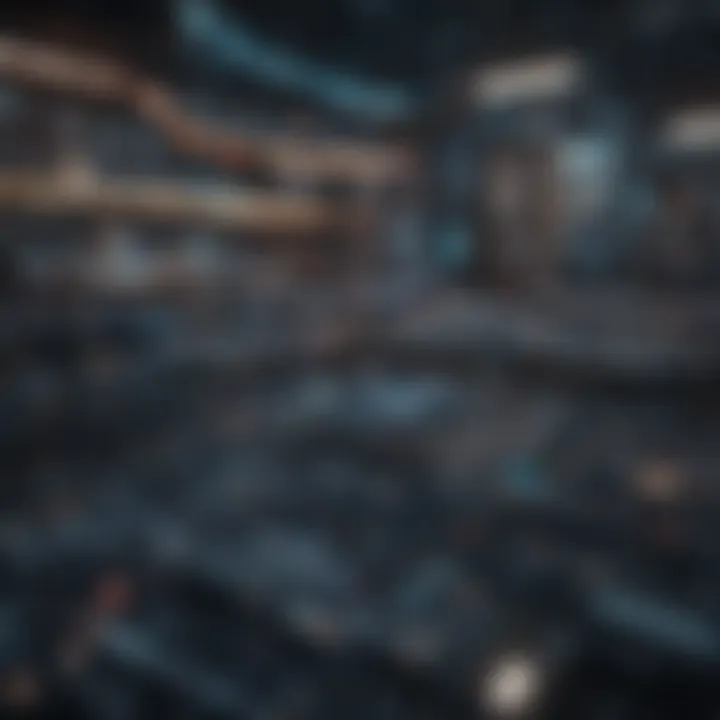
Intro
Data interoperability standards serve as the backbone of seamless data exchange across diverse platforms and disciplines. In an era where information is increasingly abundant yet fragmented, the necessity for these standards becomes glaringly apparent. Organizations and researchers rely on efficient mechanisms to enable coherent communication between different systems, datasets, and stakeholders. This exploration delves into the essence of data interoperability standards, examining their evolution, current frameworks, and future trends while addressing the multifaceted challenges faced during implementation.
Understanding data interoperability involves recognizing its relevance in today's data-driven world. Increasingly, organizations require the ability to share and integrate data sources efficiently to enhance decision-making processes, foster collaboration, and drive innovation. Data interoperability standards not only facilitate this sharing but also establish trust among various data stakeholders.
The upcoming sections will meticulously outline the significant aspects of data interoperability standards, providing a comprehensive examination centered around their importance, methodologies, and future perspectives.
Research Overview
Summary of Key Findings
Data interoperability standards are critical to enhancing the exchange of information in various fields. Research indicates that organizations adopting these standards experience improved collaboration and efficiency. Key findings from recent studies include:
- Enhanced data sharing capabilities lead to quicker decision-making.
- Organizations using interoperability standards report lower operational costs and increased productivity.
- The presence of clear standards fosters innovation by enabling the development of new applications and services.
Research Objectives and Hypotheses
The primary objective of this examination is to provide an in-depth understanding of data interoperability standards, particularly focusing on:
- The evolution and historical context of these standards.
- Current frameworks and their applicability in real-world scenarios.
- The challenges faced by organizations in implementing these standards.
- Anticipated future trends in data interoperability that may reshape industry practices.
In addition, this research tests the hypothesis that firms with established interoperability standards face fewer barriers in data sharing and integration efforts than those without such frameworks.
Methodology
Study Design and Approach
The study employs a qualitative approach, rooted in extensive literature reviews, case studies, and expert interviews. This combination allows for a nuanced understanding of the implications and realities surrounding data interoperability standards. By synthesizing perspectives from diverse sectors, the findings contribute to a comprehensive overview of existing frameworks and their impact.
Data Collection Techniques
To garner relevant insights, this investigation utilizes various data collection techniques, including:
- Literature Review: Analysis of existing academic and industry publications to contextualize the research.
- Case Studies: Examination of organizations that have successfully implemented interoperability standards to glean best practices.
- Expert Interviews: Conducting discussions with industry professionals to capture firsthand experiences and challenges in establishing interoperability.
Through these techniques, the study aims to produce a coherent and rich narrative that establishes the significance and nuances of data interoperability standards in today's fast-paced digital landscape.
"The future of data interoperability is not merely an option but a necessity for organizations aiming to thrive in a connected world."
Intro to Data Interoperability
Data interoperability is a critical concept in the realm of data management. It refers to the ability of different systems or organizations to access, exchange, and make use of data in a seamless manner. As technologies evolve, the need for effective data interoperability becomes increasingly vital. Without it, organizations can encounter significant obstacles in communication, data sharing, and overall operational efficiency.
Definition of Data Interoperability
At its core, data interoperability means that diverse systems can work together effectively. This often involves standardizing the way data is formatted and exchanged. Two main types of interoperability exist: syntactic and semantic. Syntactic interoperability focuses on the format, ensuring systems can parse and render the data properly. Semantic interoperability goes deeper, ensuring that the data retains its meaning across different contexts. For instance, consider how the term "customer" might differ in significance between a retailer and a bank. Understanding and addressing these differences is central to achieving true interoperability.
Importance in the Modern Landscape
In today’s digital world, data is an invaluable asset. Organizations generate, collect, and analyze vast amounts of data daily. This data typically resides in silos, making it difficult to share and integrate across departments or with external partners. Data interoperability provides a critical solution to this issue. By establishing common standards and protocols, organizations can ensure that data flows freely and efficiently. This not only improves operational workflows but also enhances decision-making based on comprehensive data insights. For research, collaboration, and innovation to flourish, data interoperability must be prioritized.
Goals of Interoperability Standards
The primary goals of interoperability standards are to ensure consistency, enhance data exchange, and facilitate collaboration. These standards aim to create a common language that different systems can understand. Some key objectives include:
- Establishing norms for data formats and protocols: Harmonizing how data is structured enhances accessibility.
- Promoting efficiency: Reduced duplication of efforts and minimized errors can be achieved through standardized processes.
- Encouraging innovation: Clear standards provide a foundation that allows new technologies to emerge, driving progress in various fields.
Achieving these goals sets the stage for a robust data ecosystem where information can be shared across platforms and industries.
Effective data interoperability is not just beneficial; it is essential for operational excellence in any data-driven organization.
Historical Context
Understanding the historical context of data interoperability standards provides crucial insights into their development and current relevance. History shows us the gradual evolution of these standards from rudimentary data-sharing methods to sophisticated frameworks that allow versatile data exchanges across diverse platforms. This subsection is essential as it informs us of how past challenges and innovations have shaped present methodologies.
Evolution of Interoperability Standards
The emergence of data interoperability standards can be traced back to the growing need for effective communication between systems. Various sectors, including healthcare and finance, encountered significant barriers in sharing information due to incompatible systems. Initially, organizations relied on proprietary formats that limited collaboration and increased operational costs.
Over the years, several influential bodies recognized the necessity for universally accepted standards. Various frameworks were proposed, with notable initiatives from entities like the World Wide Web Consortium (W3C) and the Institute of Electrical and Electronics Engineers (IEEE). The development of Extensible Markup Language (XML) in the late 1990s marked a tipping point in data representation, allowing for better data interchange across different systems. This period also saw a push for open standards, allowing broader access and reducing the dependency on specific vendors.
As technology evolved, so did the requirements for interoperability. The introduction of Application Programming Interfaces (APIs) in the early 2000s and the advent of cloud computing further enhanced the need for standardized communication protocols. This evolution reflects a shift toward more flexible, scalable solutions catering to the data needs of increasingly interconnected systems.
Key Milestones in Development
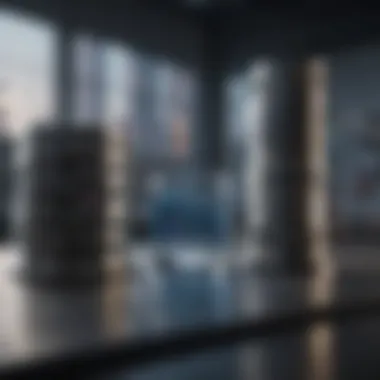
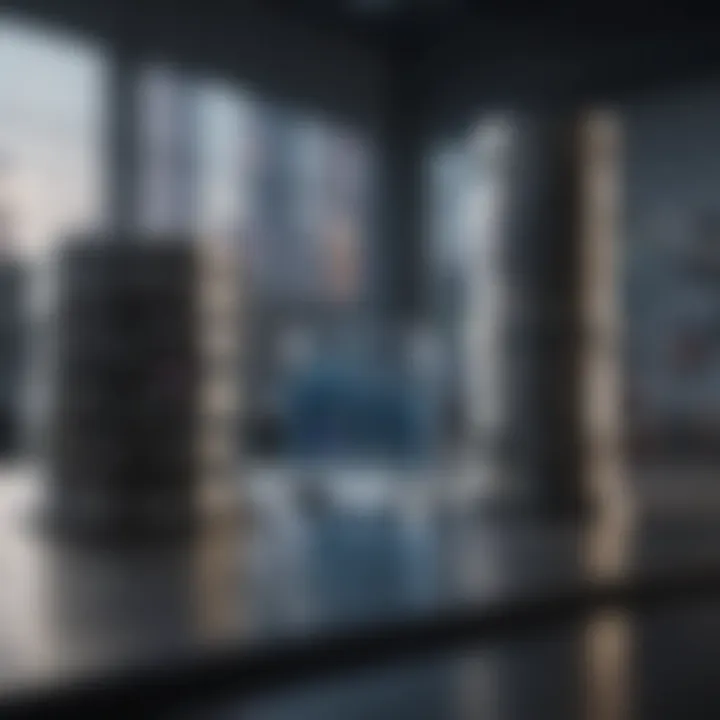
Several milestones have been pivotal in the development of interoperability standards. The adoption of HL7 in healthcare serves as one of the earliest examples. This standard emerged to ensure that systems could efficiently share patient data, leading to significant improvements in the healthcare sector.
Another noteworthy development was the introduction of the Data Catalog Vocabulary (DCAT), designed to enhance data discovery and sharing for datasets published on the web. It was a step toward making government and academic data more accessible to the public house, promoting transparency and collaboration.
Moreover, the emergence of the Internet of Things (IoT) necessitated new standards to address the unique data-sharing challenges posed by connected devices. The Open Connectivity Foundation was established to standardize communication between IoT devices, emphasizing the importance of interoperability in real-time data exchanges.
"Data interoperability standards are not just technical requirements but are essential for fostering collaboration and innovation across sectors."
These milestones represent collective attempts to create a cohesive framework for data interchange across multiple domains, thus highlighting the necessity for continued advancements in interoperability standards. As industries become more interconnected, the historical context of these developments reveals the ongoing significance of these standards in shaping future collaborations and innovations.
Current Frameworks and Standards
Current frameworks and standards are pivotal for achieving data interoperability across diverse fields. These frameworks provide the necessary structure to facilitate seamless data exchange and understanding among various systems and platforms. The evolution of these standards reflects ongoing advancements in technology and changing user needs. Additionally, they are instrumental in ensuring data comprehension and utilization in an increasingly data-driven world.
Overview of Existing Standards
Data interoperability relies on a variety of established standards that govern how data is shared and interpreted across systems. Notable frameworks include the ISO/IEC 11179 standard, which focuses on metadata registries, and the Open Geospatial Consortium (OGC) standards that aim to enhance geospatial data sharing. These standards aim to address different domains but share a common goal of enabling effective communication among disparate systems.
Some existing standards include:
- ISO/IEC 11179: Pertains to metadata and provides guidelines for data element definitions.
- HL7: This standard is crucial in the healthcare sector, facilitating the exchange of clinical and administrative data.
- Dublin Core: A simple yet effective way to describe a wide range of resources, enhancing searchability.
"Standards are crucial not only for exchanging data but also for ensuring that the meaning of that data is preserved across different systems."
Comparison of Prominent Standards
When comparing prominent standards, it's essential to understand their specific applications and effectiveness in various industries. Each standard has unique attributes that render it suitable for particular contexts. For instance, HL7 is tailored for healthcare, while the World Wide Web Consortium (W3C) offers standards applicable across web technologies.
Comparative highlights include:
- HL7 vs. FHIR: Both aim at healthcare interoperability. HL7 focuses on established data transfer methods, while Fast Healthcare Interoperability Resources (FHIR) emphasizes modern web technologies and APIs.
- ISO vs. IEEE: While ISO broadly covers a wide range of industries, IEEE often focuses on technical specifications pertinent to computing and telecommunications.
- W3C Standards: Includes standards such as HTML, CSS, and RDF that ensure web data interoperability across various platforms.
Sector-Specific Standards
Sector-specific standards are tailored to meet the unique demands of particular fields. They are essential for ensuring that data exchange is not only possible but also meaningful within a given context. For instance, in the finance sector, the Financial Industry Regulatory Authority (FINRA) implements standards that govern how financial data should be reported and shared.
Key sector-specific standards include:
- FHIR in Healthcare: A modern standard facilitating the exchange of healthcare information using APIs.
- DICOM: Used in medical imaging to ensure that images and related data can be shared seamlessly.
- XBRL: For financial reporting, this standard enhances the exchange of business information.
By recognizing the variety of standards operational in respective sectors, organizations can better plan and implement a strategy for data sharing that aligns with their objectives.
Challenges in Implementing Interoperability Standards
Implementing data interoperability standards is a complex process that poses several challenges. Understanding these hurdles helps in navigating the intricate landscape where various protocols and systems must work together. This section addresses key challenges that organizations encounter, which can inhibit the effective adoption of standards essential for smooth data exchange and collaboration.
Technical Barriers
Technical barriers are amongst the foremost challenges in implementing data interoperability standards. These barriers arise due to differences in system architectures, data formats, and communication protocols. Legacy systems often lack the ability to support modern standards, making integration arduous. Data quality and consistency must also be managed, as discrepancies could lead to faulty data exchanges and misinterpretations. Moreover, the sheer variety of technologies in use complicates the establishment of uniform standards, causing fragmentation in how data is shared across different platforms.
Effective data interoperability requires a concerted effort to bridge gaps between disparate systems and promote uniformity in data handling.
Organizational Resistance
Organizational resistance is another significant hurdle. Many organizations are reluctant to change established processes that have been in place for years. This hesitance often stems from a lack of awareness about the benefits of interoperability standards. Employees may fear additional training or perceive new systems as a threat to their day-to-day activities. Additionally, resistance can be fueled by competing interests within organizations that prioritize short-term goals over long-term benefits of data sharing. To overcome this challenge, effective communication is crucial, as is demonstrating the tangible benefits of interoperability in enhancing organizational efficiency.
Financial Constraints
Financial constraints also play a role in limiting the adoption of interoperability standards. Implementing new systems and technologies comes with a financial burden. Organizations often have limited budgets, which can restrict their ability to invest in necessary tools for maintaining compliance with interoperability standards. The costs associated with training employees, upgrading infrastructure, and performing necessary integration projects can be daunting. To deal with these limitations, organizations must prioritize investments that support interoperability, viewing them as essential for future growth and competitiveness in a data-driven world.
In summary, addressing the challenges of technical barriers, organizational resistance, and financial constraints is critical for the successful implementation of data interoperability standards. Each element requires careful consideration and strategic planning to ensure that organizations can thrive in an increasingly interconnected environment.
Case Studies of Successful Implementations
Case studies of successful implementations of data interoperability standards provide invaluable insights into how such standards can enhance data sharing and collaboration across various sectors. Analyzing these real-world examples enables organizations to identify best practices, understand the potential challenges, and see the measurable benefits that can arise from effective interoperability. These case studies not only illustrate the practical application of standards but also serve as a testament to their necessity in today’s interconnected world.
Healthcare Sector
The healthcare sector has long been at the forefront of adopting data interoperability standards due to the urgent need for efficient patient data exchange. The Fast Healthcare Interoperability Resources (FHIR) standard developed by HL7 International is a notable success story. FHIR allows different healthcare systems to communicate seamlessly, improving patient outcomes and streamlining processes.
For instance, hospitals using electronic health records (EHRs) can share patient information in real time. This real-time data exchange ensures that healthcare providers have access to the most current patient data, enhancing decision-making during critical moments.
Benefits realized through the implementation of interoperable systems include:
- Improved Patient Care: Enhanced access to patient data leads to better-coordinated care.
- Cost Efficiency: Reduced administrative burdens allow healthcare providers to allocate resources more effectively.
- Patient Empowerment: Patients can gain more control over their health data, fostering a patient-centered approach.
The challenges, however, have included difficulties in integrating legacy systems and pushback from organizations wary of sharing sensitive information. Despite these hurdles, the success of FHIR and similar standards demonstrates the transformative potential of interoperability in healthcare.
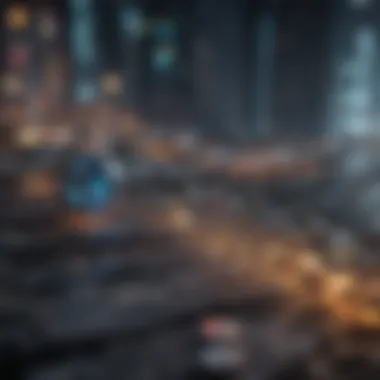
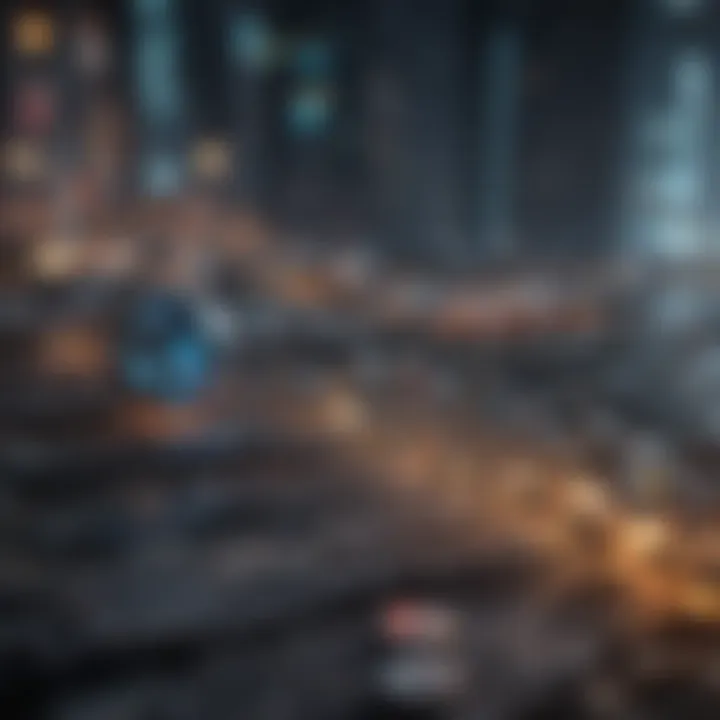
Finance and Banking
In the finance and banking sector, interoperability plays a critical role in facilitating smooth transactions and ensuring compliance with regulatory frameworks. The adoption of ISO 20022, a universal financial industry message standard, is a prime example of how interoperability can optimize financial operations.
ISO 20022 enhances data exchange between banks, payment processors, and regulatory bodies. The standard provides a common platform where different financial entities can communicate effectively, thus reducing the risk of errors in monetary transactions. A practical implication of this standard is seen in cross-border payments, where timely and accurate information sharing is crucial.
Key outcomes of implementing ISO 20022 include:
- Faster Transactions: Streamlined processes lead to quicker payments and settlements.
- Increased Transparency: Enhanced data granularity improves visibility into financial transactions.
- Regulatory Compliance: Meeting international compliance standards becomes simpler with a unified messaging framework.
Adopting such standards does not come without challenges, particularly regarding the transition from older systems and the initial costs of implementation. Nonetheless, the benefits often outweigh these risks, leading many institutions to embrace interoperability as a strategic asset.
Research Collaborations
Research institutions increasingly recognize the power of interoperability standards in fostering collaboration across disciplines. The implementation of DataCite and DOI (Digital Object Identifier) systems exemplifies this outcomes in academic research.
Using these standards, researchers can share datasets and publications effectively, ensuring that data is easily discoverable and citable. A relevant case is the collaboration among universities and open data initiatives, which promote shared access to research findings.
Benefits derived from these standards include:
- Enhanced Research Visibility: Researchers gain greater exposure for their work, which can lead to increased citations.
- Interdisciplinary Collaboration: Easier data sharing encourages collaborations that might not have been possible previously.
- Better Resource Utilization: Shared resources prevent duplication of efforts and promote innovation.
Challenges such as persistence in maintaining data quality and concerns about intellectual property rights remain significant. However, many institutions are discovering that the resulting collaborative opportunities far exceed these limitations.
"Case studies illustrate that when standards are embraced, the impact on efficiency and innovation can be profound."
These case studies highlight the essential role that data interoperability standards play in diverse sectors. By examining specific implementations, organizations can glean insights that may inform their own strategies for building interoperable systems.
Future Trends in Data Interoperability
As the digital landscape continues to evolve, data interoperability is becoming increasingly vital. This section explores the key future trends shaping how data systems interact, aiming to provide clarity on emerging innovations and the broader implications for various sectors. Significant factors include technological advancements, regulatory adjustments, and the possibility of such global standards establishing common ground across different spheres.
Emerging Technologies
The emergence of new technologies like blockchain, artificial intelligence, and machine learning is fundamentally changing the arena of data interoperability. These innovations not only enhance data sharing processes, but they can also increase data security. For example, blockchain technology offers a decentralized framework that ensures data integrity and transparency. This is vital in sectors such as finance and health care, where trust and verification are paramount.
Moreover, artificial intelligence facilitates the analysis of vast datasets, enabling systems to learn and adapt dynamically. Integrating AI into interoperability solutions allows for improved data matching and more intelligent data flow management. The adoption of Application Programming Interfaces (APIs) is also rising, creating smoother pathways for data exchange between disparate systems.
Regulatory Changes
Regulatory environments are adjusting to keep pace with technological advancements. As governments and regulatory bodies recognize the importance of data interoperability, new laws and guidelines are being established. These regulations often focus on issues like data privacy, cybersecurity, and ethical use of data. Recent developments like the General Data Protection Regulation (GDPR) in Europe show how regulatory frameworks can impact data interoperability. Compliance with such regulations is critical for organizations to ensure they do not face legal repercussions or loss of public trust.
Additionally, regulations can influence the adoption of interoperability standards by promoting or mandating their use. Organizations that align their data strategies with regulatory expectations not only improve compliance but also enhance operational efficiency.
Potential for Global Standards
The idea of global interoperability standards is gaining traction. As a surge of data flows across borders, the need for universally accepted frameworks becomes apparent. Global standards can simplify data exchange, help avoid fragmentation, and enable collaborations among countries and organizations.
However, reaching consensus on these standards poses challenges. Different regions may have unique data governance policies and cultural considerations. Despite that, the establishment of global standards could lead to significant benefits:
- Interoperability Across Industries: A common framework could streamline operations across sectors like healthcare, finance, and education.
- Cost Efficiency: Standardization may reduce the costs associated with data management and integration.
- Enhanced Collaboration: Researchers and professionals can share insights more easily, fostering innovation.
"The pursuit of global data interoperability standards marks a critical step towards a cohesive ecosystem in an increasingly connected world."
In summary, the future trends of data interoperability are influenced by emerging technologies, changing regulatory landscapes, and the drive for global harmonization of standards. Understanding these components will be crucial for stakeholders aiming to navigate the complexities of modern data exchange effectively.
Role of Open Standards
The concept of open standards remains central to the discussion around data interoperability. Essentially, these standards foster an environment where different systems, applications, and technologies can work together seamlessly. The role of open standards in data interoperability cannot be overstated. They enable communication across diverse platforms, allowing for greater flexibility and innovation in data sharing.
One significant benefit of open standards is their potential to lower barriers to entry for new technologies. When standards are open, they allow smaller enterprises and startups to participate in the data ecosystem without incurring hefty licensing fees. This leads to increased competition and drives technological advancement.
Moreover, open standards can enhance collaboration between various organizations and sectors. By providing a common framework, they encourage data exchange and integration that may not have been possible otherwise. This collaborative spirit is especially important in fields like healthcare or finance where data sharing can lead to improved outcomes.
Benefits of Open Standards
- Interoperability: Open standards allow systems from different vendors to communicate effectively. This reduces the friction involved in integrating new technologies into existing infrastructures.
- Cost Efficiency: As mentioned earlier, open standards significantly reduce costs. Organizations do not have to pay for proprietary technologies, enabling them to allocate resources more efficiently.
- Innovation: With open access, developers can create new applications or services that build on existing technologies. This fosters an environment of innovation and makes it easier to explore new use cases.
- Flexibility: Open standards provide flexibility in choosing software and hardware. Organizations are not locked into a specific vendor's technology.
- Community Support: Many open standards benefit from community-driven support, leading to faster resolution of issues and improvements in the standards over time.
Challenges with Adoption
Despite these benefits, there are challenges associated with the adoption of open standards. Some organizations may resist change due to established practices and infrastructure. This resistance can stem from fear of the unknown or lack of understanding of the benefits open standards can provide.
Additionally, coordination among stakeholders can be difficult. Various organizations may have differing priorities and agendas, which can complicate the development and adherence to common standards.
Another hurdle is ensuring the security of open standards. Since these standards are accessible to anyone, they may also be subject to vulnerabilities. Organizations must balance the openness of standards with the necessity for robust security measures to protect sensitive data.
Interoperability in the Internet of Things
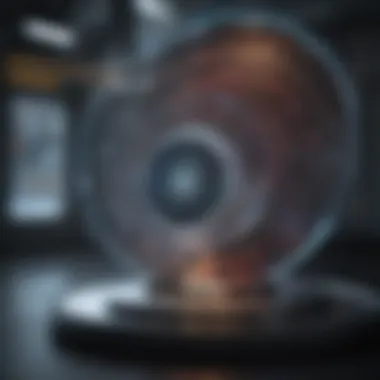
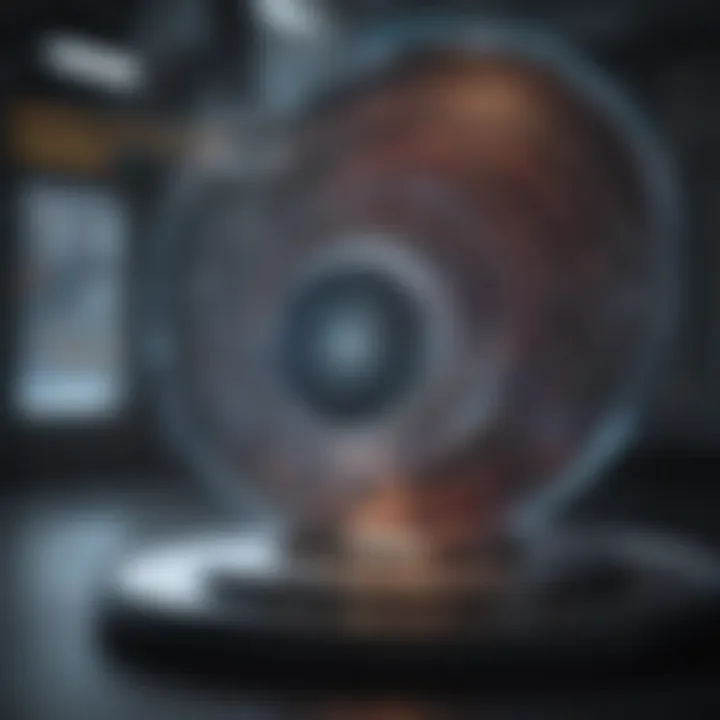
Interoperability is a crucial aspect of the Internet of Things (IoT). It refers to the ability of different devices and systems to communicate and work together seamlessly. In the context of IoT, this means ensuring that a diverse range of devices—from smart thermostats to industrial sensors—can share and interpret data effectively. The significance of interoperability in IoT cannot be overstated.
Importance of Interoperability in IoT
The importance of interoperability in IoT lies in its ability to enable a connected environment. Without it, devices would operate in isolation, limiting their functionality. Here are several key reasons why interoperability is essential:
- Enhanced Connectivity: Interoperability facilitates the connection of devices from different manufacturers. This connectivity allows for a richer user experience and smarter automation, as devices can respond to each other’s data.
- Improved Data Sharing: The ability to share data in real-time is critical. Interoperable systems can provide a more comprehensive view of operations, whether in homes, industries, or cities.
- Cost Efficiency: By using standardized communication protocols, organizations can save on costs related to integration and maintenance. Interoperability also reduces the chances of vendor lock-in.
- Scalability: As new devices are added, interoperability ensures they can integrate into existing systems without requiring extensive changes. This is vital in dynamic environments like smart cities where technology constantly evolves.
- Innovation and Competition: A standard interoperable framework drives innovation. It allows different developers to create solutions that can easily integrate with existing devices and platforms, fostering a competitive market.
Standards Governing IoT Communications
To achieve effective interoperability, several standards have been developed. These standards designate how devices communicate and exchange data. Some of the most notable standards include:
- MQTT (Message Queuing Telemetry Transport): A lightweight messaging protocol designed for devices with limited processing capabilities. It enables efficient communication over networks with low bandwidth.
- CoAP (Constrained Application Protocol): This protocol is optimized for use in resource-constrained devices and networks. It allows IoT devices to communicate efficiently, particularly in home automation.
- Zigbee and Z-Wave: These are wireless protocols used in home automation. They enable devices from different manufacturers to work together within a smart home ecosystem.
- HTTP/HTTPS: Though traditionally used for web communication, these protocols are also relevant in IoT since devices often connect to cloud services.
- WebSockets: This standard facilitates real-time communication between clients and servers, making it ideal for applications where timely data exchange is critical.
Having comprehensive standards for IoT communications ensures that devices can interoperate regardless of the manufacturer. This is crucial for a genuinely connected ecosystem where devices need to function together harmoniously.
"Interoperability is the cornerstone of a functioning Internet of Things ecosystem, driving efficiency and innovation across industries."
In summary, interoperability is fundamental to the effectiveness of IoT. It enhances connectivity, enables real-time data sharing, promotes cost-efficiency, and supports scalability. By adhering to established communication standards, organizations can foster an environment that maximizes the potential of IoT technology.
Data Interoperability and Artificial Intelligence
Data interoperability standards are essential in the context of artificial intelligence (AI). As AI relies heavily on data for training algorithms, the quality and accessibility of this data are paramount. Without proper interoperability, various systems may not communicate effectively, which can hinder machine learning processes and limit the potential of AI applications. This section will explore how interoperability impacts AI development and the standards that guide data usage within AI systems.
Impact on AI Development
The development of artificial intelligence fundamentally relies on the ability to share and integrate data across different platforms and systems. Interoperability allows AI systems to access diverse datasets, improving the robustness and reliability of machine learning models.
A few key reasons highlight the importance of data interoperability for AI:
- Diverse Training Data: AI systems benefit from training on varied datasets. This diversity fosters better generalization of models, making them more capable in real-world scenarios. Interoperability ensures that AI applications can leverage data from various sources, enhancing their learning capabilities.
- Collaboration Between Systems: Many AI applications require real-time data from multiple sources. For instance, autonomous vehicles rely on input from sensors, maps, and other vehicles. Strong interoperability standards help achieve seamless data exchange, improving system responsiveness and safety.
- Research Advancements: In academic contexts, the sharing of data is crucial for validating and replicating research findings. Interoperable data allows researchers to build upon each other’s work more effectively, leading to more rapid advancements in AI technology.
"Data interoperability is not only a technical requirement; it is a strategic advantage in developing cutting-edge AI solutions."
Standards for Data Used in AI Systems
Establishing standards for data used in AI systems is essential for ensuring quality, consistency, and ethical usage. Several frameworks guide the implementation of interoperability in AI environments:
- Structured Data Standards: Formats such as JSON, XML, or CSV facilitate data exchange between different systems. These standards outline how data should be formatted and organized, allowing for more straightforward integration into AI models.
- Semantic Interoperability: This concept refers to the ability of different systems to understand and interpret shared data correctly. Standards such as the Resource Description Framework (RDF) and Web Ontology Language (OWL) support semantic interoperability by providing mechanisms for describing data meanings.
- Data Governance Frameworks: Ethical considerations are central when dealing with AI and interoperable data. Frameworks like the General Data Protection Regulation (GDPR) set standards for data privacy and user consent, ensuring that AI systems use data responsibly.
In summary, the role of data interoperability in AI is multifaceted. It not only influences the development and effectiveness of AI systems but also ensures that such systems operate within a framework of ethical and responsible data use.
Ethical Considerations in Data Interoperability
Data interoperability involves exchanging data seamlessly across diverse systems and platforms. However, this interconnectedness raises significant ethical considerations that must be addressed. These concerns center on privacy, data ownership, and the impact on different stakeholders. Understanding these aspects is crucial, as they shape not only the technical landscape but also the moral foundations of data sharing practices.
Privacy Concerns
Privacy is a paramount concern in the realm of data interoperability. As diverse systems communicate and share information, the risk of exposing sensitive personal data increases. Organizations must ensure that they do not inadvertently share confidential information. This requires robust privacy standards, which protect user data from unauthorized access.
The significance of these privacy measures cannot be understated. Users need assurance that their data is handled correctly and that their rights are respected. With reputable standards like the General Data Protection Regulation (GDPR) in Europe, companies are required to adopt stringent privacy practices. Maintaining transparency about how data is used and shared builds a foundation of trust between organizations and individuals.
Moreover, continuous monitoring and auditing processes support compliance with privacy regulations. Without such efforts, organizations risk facing not only legal penalties but also reputational damage that can result from data breaches. A collaborative approach can help strike a balance between functionality and privacy.
Data Ownership Issues
Data ownership is another complex ethical consideration in interoperability. When data is shared across systems, questions about its ownership can become murky. Who owns the data? The entity that collected it, the organization that processed it, or the individual it belongs to?
These questions highlight the need for clear agreements and terms that delineate data ownership. Organizations should define who holds rights to the data and under what conditions it can be used and shared. This clarity helps mitigate potential disputes and aligns stakeholders' expectations.
Furthermore, considerations around data rights apply not only to organizations but also to individuals. Users may want to control how their data is used, including opting out of data sharing where feasible. By incorporating user feedback into policies, organizations can foster a more ethical approach to data sharing that respects individual rights.
The intersection of data interoperability and ethics demands careful consideration to ensure that all stakeholders are treated with respect and fairness.
To summarize, ethical considerations in data interoperability significantly impact how organizations approach data sharing. By addressing privacy concerns and defining data ownership clearly, businesses can create a more transparent and responsible environment. This approach not only protects individual rights but also establishes a strong foundation for ethical data practices.
End
The conclusion serves as a pivotal element in our exploration of data interoperability standards. This section emphasizes the critical importance of understanding these standards in the context of the current and evolving data landscape. With data becoming increasingly integral to various sectors such as healthcare, finance, and academia, the establishment of robust interoperability standards is essential.
Summary of Key Insights
Throughout this article, several key insights have emerged regarding data interoperability standards:
- Definition and Importance: Data interoperability relates to the ability of different systems and organizations to communicate and exchange information seamlessly. As organizations navigate the complexities of data management, interoperability enhances efficiency and collaboration.
- Implementation Challenges: While the benefits are clear, implementing these standards is fraught with challenges. Technical barriers, organizational resistance, and financial constraints often hinder progress.
- Evolving Frameworks: The landscape of interoperability standards is dynamic. New technologies and regulatory changes continue to shape existing frameworks, demanding adaptability from all stakeholders.
- Ethical Considerations: The implications of data interoperability extend beyond technical and organizational aspects. Issues regarding privacy and data ownership are crucial for ensuring ethical practices.
- Future Trends: The trajectory for data interoperability suggests an increased emphasis on global standards and integration with emerging technologies.
This summary encapsulates the essential elements that bind the overarching theme of data interoperability.
Final Thoughts on Future Directions
Looking into the future, the direction of data interoperability standards appears promising yet complex. Several factors will influence this domain:
- Emerging Technologies: As artificial intelligence, machine learning, and blockchain technology evolve, these can significantly impact how interoperability standards are developed and implemented. There is a potential for these technologies to streamline processes and enhance data sharing capabilities.
- Global Collaboration: To address the challenges of interoperability on a larger scale, international cooperation among different nations, sectors, and organizations will be crucial. Collaborative efforts can lead to the establishment of universally accepted standards.
- Regulatory Evolution: Regulators will likely adapt to the changing landscape of data use. Ethical considerations surrounding privacy and data ownership must be at the forefront of future legislation to protect individuals and organizations alike.