Harnessing Data Analytics in Psychology
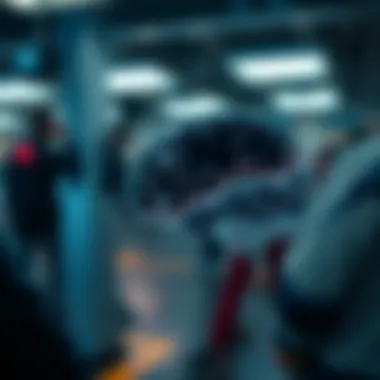
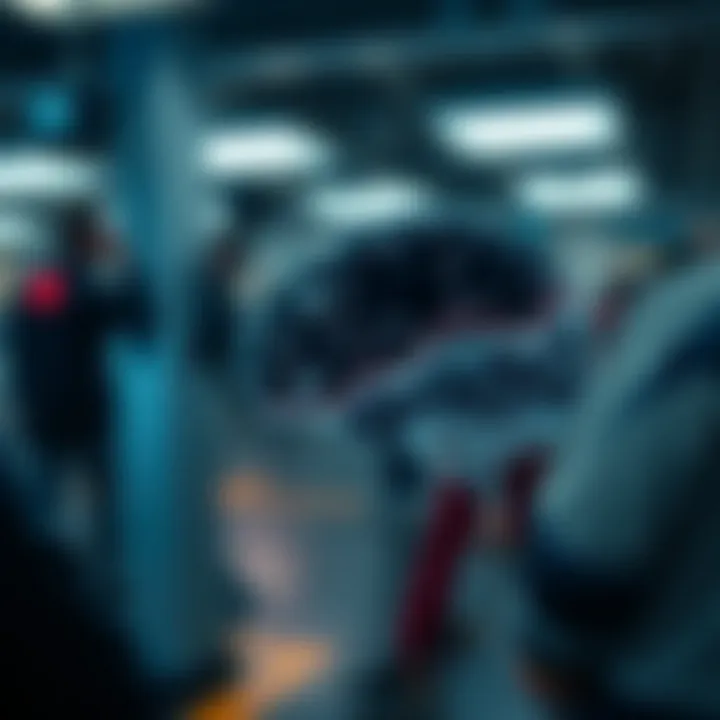
Intro
In recent years, the convergence of data analytics and psychology has sparked an invigorating dialogue within the realms of scientific exploration. As we navigate through the complexities of human behavior, the ability to extract meaningful patterns from vast troves of data has become an indispensable tool. This alliance not only enriches our understanding but also sheds light on various applications, from clinical psychology to consumer behavior and social dynamics. With data analytics painting the broader strokes of our inquiry, the nuanced palette of psychological insight emerges, revealing layers of interpretation that might have lain dormant without these analytical techniques.
Understanding human behavior is akin to piecing together a jigsaw puzzle—each data point represents a fragment that, when examined under the right lens, can contribute to a greater narrative. As we transition into the next sections, we will explore the significant findings, objectives, and methodologies that define this interdisciplinary intersection. Let’s delve into the core elements that illuminate the implications of data analytics within psychology, and how these insights can reshape our understanding of the mind.
Research Overview
Analyzing data within psychology isn't just about numbers; it's about stories, motivations, and understanding the very essence of what it means to be human. Amidst the myriad of studies, certain findings stand out as beacons of significance.
Summary of Key Findings
- Data analytics has enabled psychologists to identify behavioral trends at an unprecedented scale.
- The application of machine learning techniques has allowed researchers to predict outcomes in clinical settings more accurately—think of it as having a crystal ball but grounded in scientific rigor.
- Insights drawn from social media platforms have provided real-time data on public sentiment, facilitating a deeper grasp of collective human behavior in various contexts.
- Ethical considerations have emerged as paramount, emphasizing the importance of responsible data usage to protect individual privacy and ensure the integrity of psychological practices.
Research Objectives and Hypotheses
The primary objectives guiding this exploration include:
- Establishing a framework for understanding how data analytics can provide deeper insights into psychological phenomena.
- Identifying methodologies that effectively combine psychological theories with analytical techniques to enhance research outcomes.
- Exploring the ethical implications of data collection and analysis in psychological studies.
The preliminary hypothesis postulated in this article suggests that the integration of data analytics methods significantly improves the accuracy and depth of psychological research findings.
Methodology
Understanding how we approach research is crucial in solidifying the findings. So let's break down the methodology used in studies about data analytics in the realm of psychology.
Study Design and Approach
The studies reviewed often adopt a mixed-methods approach, combining qualitative insights with quantitative data. This way, researchers can engage more constructively with human experiences while also relying on numerical evidence. Often, longitudinal studies that track changes over time permit a more nuanced understanding of behavioral evolution.
Data Collection Techniques
In terms of data collection, various techniques are in play:
- Surveys and Questionnaires: Standard tools that gather self-reported data from participants about their thoughts, feelings, and behaviors.
- Observational Techniques: These involve direct observations of behavior in natural settings, providing rich context and spontaneous data.
- Digital Footprints: With the prevalence of online activity, data is increasingly sourced from social media interactions, web browsing patterns, and app usage statistics. This form of analytics demands careful consideration of ethical boundaries and privacy issues.
"In the realm of data and psychology, every byte of information is a potential revelation, waiting for the right analysis to bring it to light."
As we continue to unpack the implications and opportunities afforded by data analytics in psychology, it becomes clear that fostering collaboration between psychologists and data scientists is essential. This partnership could pave the way for innovations that uphold both scientific rigor and ethical standards.
Preface to Data Analytics and Psychology
Data analytics plays a pivotal role in modern psychology, transforming the way we understand and interpret human behavior. It merges numbers with emotions, offering insights that were once buried beneath layers of qualitative observations. In a world increasingly driven by data, this intersection becomes essential not just for academics, but for practitioners and policy-makers alike.
The main benefits of incorporating data analytics into psychology include improved decision-making, targeted interventions, and the unveiling of complex behavioral patterns. For instance, the utilization of analytical techniques can lead to more effective treatment plans in clinical settings, as professionals can now base strategies on robust data rather than solely relying on anecdotal experiences. Moreover, analytics can illuminate trends in consumer behavior, giving marketers and psychologists an edge in predicting how susceptible individuals might react under various circumstances.
As we navigate the intricacies of this field, it's crucial to consider the ethical implications of harnessing data for psychological purposes. Issues such as data privacy and user consent are paramount, as the information gathered can often include sensitive personal insights. Therefore, a delicate balance must be struck between leveraging data for meaningful insights and maintaining the integrity of individual privacy.
Understanding the historical context of capitalizing on data analytics in psychology sets the stage for appreciating its current applications. From early experimental designs to the latest machine learning algorithms, this evolution signifies not just advancements in technology, but a profound shift in how we interpret the human experience.
By unpacking this complex relationship, we hope to provide a roadmap for students, researchers, and professionals alike, guiding them through the ins and outs of data analytics in psychological research and application.
Methodologies in Data Analytics
In the ever-evolving landscape of psychology, methodologies in data analytics play a pivotal role in deciphering human behavior and improving mental health strategies. Understanding these methodologies provides a deeper insight into how psychological phenomena can be quantified, analyzed, and effectively addressed. The symbiotic relationship between data analytics and psychology allows researchers to navigate complex behavioral patterns, leading to the development of more nuanced and accurate theories. Each methodology is like a tool in a toolbox, adding precision to the exploration of the human psyche.
Quantitative vs. Qualitative Data
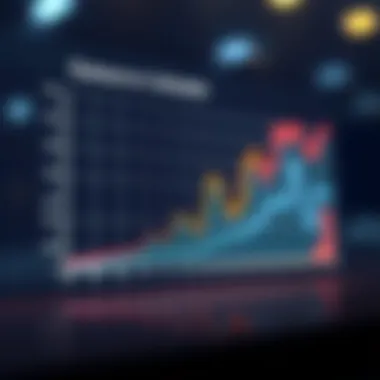
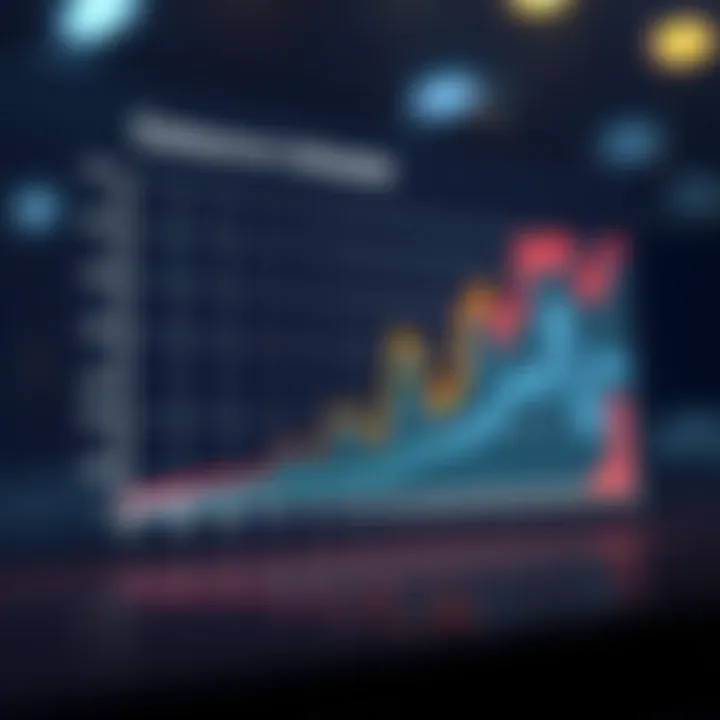
When diving into the methodological sea, one must grapple with the dichotomy of quantitative and qualitative data. Quantitative data embraces numbers, positing that relationships can be measured, scores can be calculated, and predictions can be made based on statistical findings. This approach is instrumental in studies seeking to quantify mental health outcomes, assess symptom severity, or even explore correlations between demographic factors and behavioral tendencies.
On the flip side, qualitative data brings a rich, narrative texture to psychological research, delving into the subjective experiences of individuals. Interviews, focus groups, and case studies furnish rich insights that numbers alone can't capture. For instance, understanding a patient's lived experience with depression requires more than mere symptom checklists; it necessitates hearing their voice, their story, their unique context.
Both types of data hold significance in psychological research:
- Quantitative data allows for broad analyses and generalizable findings.
- Qualitative data provides depth and context, enriching the understanding of human behavior.
Thus, the interplay between quantitative and qualitative methods creates a more comprehensive analytical approach, acknowledging the complexity of psychological phenomena.
Data Mining Techniques
Data mining techniques involve sifting through vast amounts of psychological data to uncover patterns that remain hidden. In a world increasingly reliant on data, this methodology is akin to finding diamonds in the rough. Techniques such as clustering, association rule learning, and anomaly detection are commonly employed in psychological studies to derive meaningful insights.
For instance, clustering can help therapists identify subgroups of patients who exhibit similar symptoms, allowing for more tailored interventions. Imagine a psychologist analyzing data from hundreds of patients, utilizing clustering algorithms to discover distinct patterns. This can lead to opportunities for targeted therapy that resonates with specific demographic or symptom-based groups.
Moreover, association rule learning can shine a spotlight on co-occurring behaviors or conditions. Picture researchers examining data sets that record patient habits; they might find that individuals who frequently experience anxiety also tend to engage in avoidance behaviors. Such revelations can inform clinical practices and preventive measures.
Data mining opens the door to analyzing data not just as isolated pieces but as interlinked webs of information, creating a more intricate understanding of human behavior.
Machine Learning in Analyzing Psychological Data
Machine learning has carved out a prominent space in the realm of psychological data analysis. By employing algorithms that learn from data patterns, researchers can make predictions about future behaviors and outcomes. This approach is particularly beneficial in tailoring interventions and understanding complex psychological constructs.
For instance, imagine a machine learning model trained on a diverse set of therapy session transcripts. This model can help identify language patterns that correlate with positive or negative therapy outcomes, enabling clinicians to refine their techniques. Suddenly, it’s not just about trial and error; it’s about informed decision-making grounded in evidence.
Here are a few ways machine learning is transforming psychological analysis:
- Predictive Analytics: Anticipate mental health crises based on historical data trends.
- Natural Language Processing: Analyze texts or transcripts to unearth emotional tones and underlying themes.
- Image Recognition: Study facial expressions in therapeutic settings to gauge emotional responses.
As machine learning continues to advance, its impact on psychology becomes increasingly profound. This methodological rigor not only enhances research accuracy but also opens pathways for proactive mental health strategies that respond to individual needs.
Through these methodologies, the field of psychology can leverage analytical power to unpack intricate human behaviors, illuminate hidden patterns, and ultimately, offer better psychological support tailored to the complexities of individual lives.
Applications in Psychological Research
Understanding human behavior relies heavily on rigorous methodologies in psychological research. Data analytics plays an increasingly significant role in this realm, influencing how researchers make sense of complex patterns and trends in human behavior. The integration of analytics not only refines existing practices but also opens new avenues for insights that were previously unfathomed. Through quantitative and qualitative techniques, researchers can derive far-reaching conclusions about cognitive, emotional, and social dimensions of individuals.
Clinical Psychology
In clinical psychology, data analytics aids in comprehensively evaluating patients' mental health issues. Clinical settings often gather large volumes of data, including survey responses, behavioral assessments, and treatment outcomes. Healthcare professionals can glean valuable insights from this data, ultimately enhancing patient treatment plans. For instance, machine learning techniques can help predict patient responses to certain therapies based on historical data, allowing therapists to customize interventions accordingly.
In recent years, research has illustrated how big data can refine diagnoses and improve therapeutic outcomes. By analyzing trends in symptoms and treatment success rates across diverse demographics, psychologists are becoming better equipped to address specific issues like anxiety, depression, or trauma.
Consumer Behavior Analysis
Consumer behavior analysis stands as a critical application within the domain of psychology, particularly in understanding how individuals make purchasing decisions. Data analytics offers robust tools for breaking down buying patterns, preferences, and motivations. Instead of relying solely on traditional surveys, businesses can now monitor online activity data, including clicks, interactions, and purchase history.
Such techniques allow marketers to track sentiment and tailor marketing strategies accordingly. Notably, the ability to segment consumer types based on their behavior enhances targeting and messaging, making campaigns more effective. For instance, a retailer might analyze purchasing behaviors to design personalized advertising that aligns with specific buyer personas. This not only improves the marketing efficiency but also deepens the customer relationship by making them feel understood and valued.
Social Psychology and Group Dynamics
When we pivot to social psychology, data analytics enables deeper understanding of group dynamics and interpersonal relationships. These aspects are crucial for dissecting how individuals behave in various social contexts—be it within organizations, communities, or social networks. By employing analytical tools, researchers can explore how group identities, biases, and social influences drive behavior.
An example includes analyzing social media interactions to understand how collective attitudes shift over time. Statistical data derived from platforms like Facebook or Twitter helps psychologists grasp the essence of social phenomena, from viral trends to public sentiments regarding particular issues. Moreover, network analysis can provide insight into how the structure of social connections impacts beliefs and actions.
Case Studies: Impact of Data Analytics
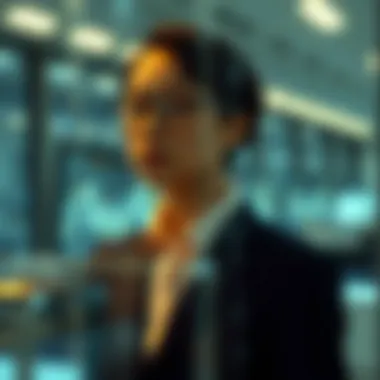
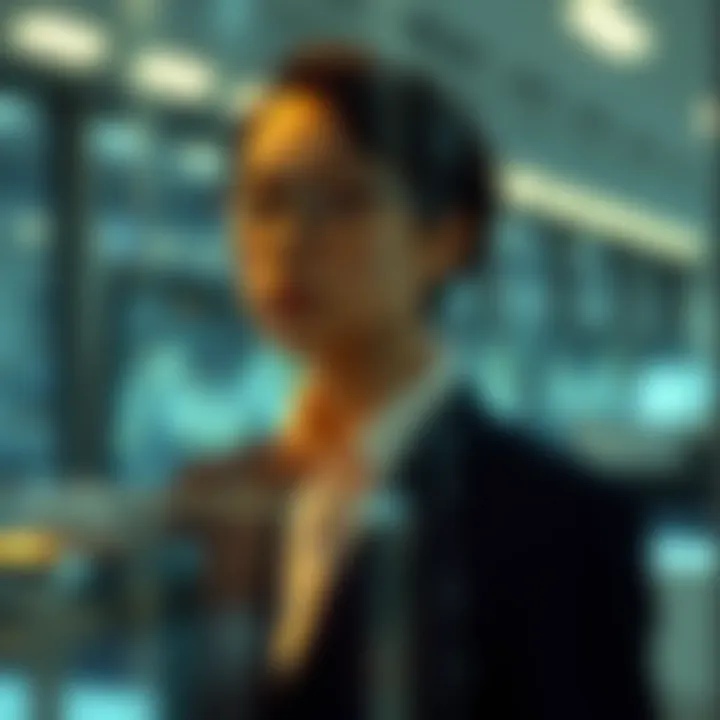
The integration of data analytics into psychology emerges as a transformative force. The impact of case studies within this intersection serves as a compelling narrative, illustrating how analytical insights can lead to tangible changes across various psychological domains. Such case studies act as a bridge, connecting theoretical knowledge with practical applications, thus offering a deep dive into methods that have proven effective in diverse settings. They highlight not only successes but also the lessons learned from endeavors that didn’t pan out as predicted. This rich tapestry of experience frames the discussion on how data-driven approaches can refine psychological practices and improve human well-being.
Success Stories in Clinical Settings
In the realm of clinical psychology, data analytics brings an array of success stories that resonate with practitioners and patients alike. For instance, a notable example can be found in the area of depression treatment. The use of predictive analytics can identify which patients are likely to benefit from specific forms of therapy, such as Cognitive Behavioral Therapy or medication. Researchers at Stanford University conducted a study employing machine learning techniques to analyze patient records, revealing patterns that helped optimize treatment plans. With this data at hand, clinicians can tailor approaches to meet individual needs, leading to better outcomes and higher satisfaction rates among patients.
Moreover, consider the work done in therapeutic settings that apply sentiment analysis to patient interactions. By analyzing speech patterns and word choices through natural language processing, therapists can gain insights into a patient’s emotional state. This analysis offers a more nuanced understanding of the patient’s mental health, allowing for more timely interventions when necessary. With cases supporting such innovative approaches, it’s clear that data can illuminate paths to healing in ways that traditional methods may not.
Innovations in Marketing Psychology
The influence of data analytics on marketing psychology is equally noteworthy. Companies are harnessing data to comprehend consumer behavior on deeper levels. A particularly innovative case involved a beverage company that employed advanced analytics to analyze purchasing patterns alongside social media engagement. They discovered that marketing strategies focused on emotional narratives significantly enhanced consumer response rates compared to conventional appeals.
When tapping into tools like cluster analysis, marketers can segment their audience more effectively. This segmentation allows for the creation of targeted campaigns that resonate with specific demographics, enhancing personal connection and ultimately driving sales. Additionally, the insights derived from analyzing customer feedback and reviews provide invaluable information that companies use to refine products and marketing strategies, leading to a more savvy approach to advertising that aligns with consumer sentiments.
Trends in Social Media Psychology
Social media platforms serve as rich veins for psychological data analysis, revealing trends that shape public perception and group dynamics. A compelling case to note arises from a study conducted by researchers at the University of Southern California, who used analytics to monitor discussions around mental health on platforms like Facebook and Twitter. This research unearthed critical insights into how social media influences individuals' perceptions of mental health and stigma.
The methodological approach involved sentiment analysis of posts and comments, revealing fluctuations in mood and attitudes toward therapy during specific events, such as Mental Health Awareness Month. The data gathered illustrated that with strategic communication, campaigns could effectively challenge stigmas and promote mental health resources. By harnessing trends from social media discussions, psychologists can better understand societal attitudes, shaping future interventions that resonate on a communal level.
"Case studies in data analytics allow us to visualize the stories of individuals and communities, illustrating the tangible effects of our analyses in ways numbers alone cannot convey."
In summary, the impact of case studies on data analytics in psychology not only sheds light on the successes achieved but also encourages ongoing exploration and adaptation within the field. These narratives reveal the intricacies involved in merging psychology and data, providing a firm foundation for future innovations.
Ethical Considerations
The advancement of data analytics within psychology opens up fascinating avenues for understanding human behavior. Yet, with great power comes great responsibility. Ethical considerations remain paramount as the psychological community increasingly relies on data-driven techniques. Addressing these elements not only safeguards individuals' rights but also upholds the integrity of research.
Data Privacy and User Consent
In the realm of data analytics, the protection of personal information is not just a legal mandate; it’s an ethical cornerstone. Individuals must know how their data is collected, used, and stored. Consent should not only be obtained but should be informed and revocable.
In practical terms, this means researchers need to explain the purpose of data collection in simple language. For example, if a study aims to analyze consumer behavior through online shopping patterns, participants should understand how their browsing history will contribute to the findings. If data is anonymized, it has to be done effectively to prevent re-identification later. Measures like data encryption and strict access controls can bolster this trust.
Moreover, the implementation of frameworks—like the General Data Protection Regulation (GDPR) in Europe—sets a strong precedent for ethical data handling in psychological research. As Peter Drucker once noted, "What gets measured gets managed." If data privacy is neglected, the measurement of human behaviors may not be managed ethically, leading to mistrust and potential harm.
Bias and Integrity in Data Collection
The integrity of data collected is vital for valid outcomes. Biases—whether introduced deliberately or inadvertently—can skew results and jeopardize the credibility of research. For instance, if data predominantly features responses from a specific demographic group, the findings may not accurately reflect broader trends.
Bias can creep into various stages of data collection, starting from survey design to the sampling process. A classic example involves online surveys that might exclude individuals without internet access, leading to an unrepresentative sample. Moreover, cultural biases in interpreting responses must be addressed to maintain the integrity of the findings.
Steps to Mitigate Bias:
- Implement diverse sampling techniques to ensure wide representation.
- Regularly audit data collection processes for unintentional biases.
- Train researchers on cultural competencies and recognition of personal biases.
As the saying goes, "an ounce of prevention is worth a pound of cure." Addressing bias is not merely a qualitative concern; it is critical for the empirical value of psychological studies.
Responsible Reporting of Findings
Once data is collected and analyzed, how researchers communicate their findings remains crucial. Responsible reporting should focus on transparency and avoid sensationalism. Misinterpretation or exaggeration can mislead the public, policymakers, and even practitioners. A study that reports a correlation without clarifying that it does not imply causation can generate incorrect assumptions about human behavior.
Additionally, conflicts of interest must be disclosed when reporting findings. For instance, if a researcher is funded by a corporation that stands to gain from positive results, bias may influence the outcomes. Honesty is the best policy—acknowledging limitations of the study is also essential. This fosters an environment of trust where other researchers and stakeholders can build upon previous work without falling prey to misleading statements.
Key Considerations for Responsible Reporting:
- Balance positive outcomes with limitations of the study.
- Disclose funding sources and potential conflicts of interest.
- Use clear language to explain findings without jargon.
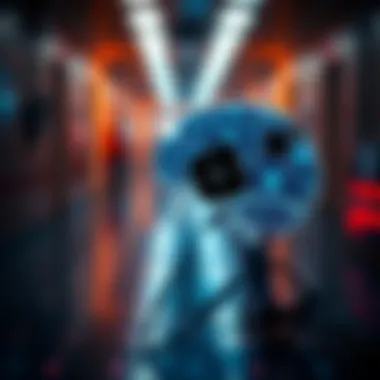
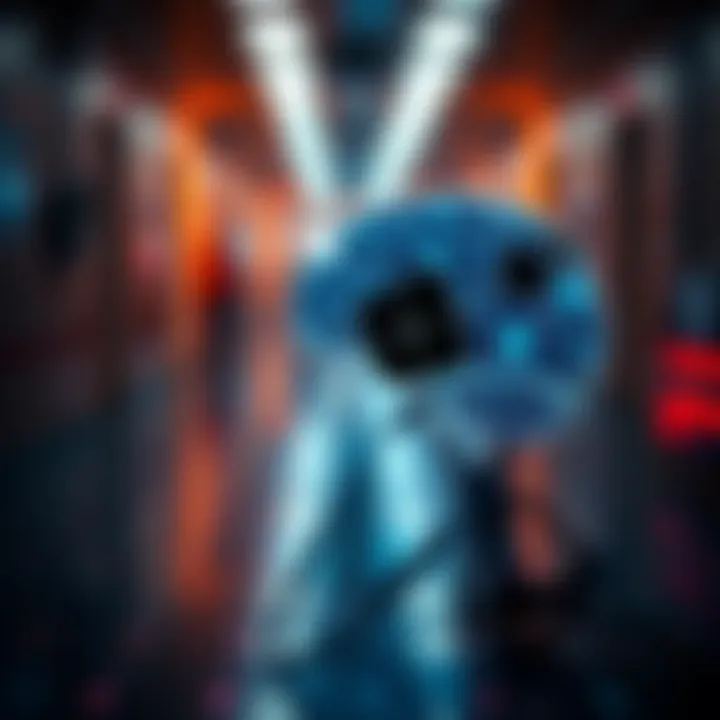
Overall, ethical considerations in data analytics shouldn’t just be an afterthought; they should be part and parcel of every stage of the research process. As psychology becomes more intertwined with intricate data techniques, fostering a culture of ethical mindfulness will ensure not only the protection of individuals but also the advancement of the field itself.
Future Directions in Data Analytics and Psychology
The field of psychology is witnessing unprecedented changes driven by data analytics. As we look forward, the potential for innovative methodologies and applications continues to grow. Understanding these future directions is crucial for professionals seeking to enhance their effectiveness in research and practice. This exploration highlights specific elements such as the integration of computing techniques, the implications for population health, and the necessity for collaborative research approaches.
Integrating Advanced Computing Techniques
In the coming years, the integration of advanced computing techniques is expected to revolutionize data analytics within psychology. Fields such as neural networks, deep learning, and natural language processing are showing promising results in analyzing complex psychological data.
"Many psychologists are not yet familiar with how these techniques can be directly applied to their work, but the potential benefits are enormous."
For instance, using machine learning algorithms, researchers can analyze vast amounts of data from surveys or social media to discern patterns of behavior that were previously hard to identify. These methods can also enhance predictive modeling for mental health disorders, allowing for earlier interventions and customized treatment plans based on individual profiles.
- Key benefits of integrating advanced computing techniques include:
- Enhanced data processing speed: This enables quicker insights into psychological trends.
- Greater accuracy in predictions: More accurate data analysis reduces biases inherent in traditional methods.
- Ability to uncover hidden patterns: Advanced analytics can reveal relationships between variables that aren’t immediately evident.
Population Health and Data-Driven Interventions
A decisive area of focus for future developments lies in leveraging data analytics for population health and targeted, data-driven interventions. By analyzing demographic data, medical records, and behavioral trends, psychologists can address mental health issues at a community level. This approach can significantly optimize public health initiatives by targeting specific populations based on their unique needs.
For instance, real-time data analysis can identify spikes in anxiety or depression within specific communities, enabling mental health professionals to deploy resources effectively.
- Possible applications include:
- Community outreach programs based on identified stressors within a population's socioeconomic profile.
- Utilization of predictive analytics to anticipate future trends in mental health issues among specific age groups or demographics.
- Development of tailored online resources and mobile apps that meet the needs of different population segments.
Collaborative Research Approaches
Looking ahead, fostering collaborative research approaches between data scientists and psychologists is absolutely essential. Such collaboration can bridge the gap between quantitative data analytics and qualitative understanding of human behavior.
Collaboration is not just an option; it's a necessity. By pooling expertise from different domains, we can gain a more holistic understanding of psychological phenomena.
- Effective collaborative strategies can include:
- Multidisciplinary research teams that include statisticians, psychologists, and software engineers.
- Joint workshops and seminars to share knowledge and best practices.
- Creation of shared databases that enhance accessibility and allow for broader analyses across various studies.
These collaborative efforts are fundamental for developing robust, evidence-based interventions that are not only scientifically valid but also ethically sound. As the fields of data analytics and psychology continue to merge, leveraging diverse expertise will be the cornerstone of groundbreaking research and practice.
Capturing the insights from these future directions ensures that the relationship between data analytics and psychology evolves into a dynamic partnership, driving improvements in mental health research, treatment, and education.
End
In closing, the intersection of data analytics and psychology is not merely an academic curiosity; it’s a transformative force that paves the way for personalized and precise approaches in understanding human behavior. The insights garnered through data-driven methodologies empower researchers and practitioners alike, facilitating a deeper understanding of psychological phenomena. This relationship not only enhances efficacy in treatment but also enriches theoretical frameworks applicable across several domains in psychology.
Recap of Key Insights
To summarize the essential aspects discussed in this article, we can reflect on several pivotal insights:
- Integration of Methodologies: Both quantitative and qualitative data approaches are crucial. Utilizing diverse methodologies enhances the richness of findings, allowing for comprehensive analysis.
- Real-World Applications: The use of data analytics has tangible beneficial outcomes in clinical settings, shaping interventions and improving patient outcomes. For instance, predictive algorithms can identify at-risk individuals before onset of severe psychological issues.
- Ethical Considerations: The responsible use of data cannot be overstated. Privacy, bias, and informed consent remain paramount in ethical discussions surrounding psychological studies, ensuring that data-driven findings translate into responsible practices.
- Future Innovations: The evolving landscape of technology promises new tools and frameworks to advance psychological research. As data analytics continue to develop, they can reveal patterns not currently comprehended, enhancing our grasp of the human psyche.
The Evolving Relationship between Data and Psychology
The bond between data analytics and psychology is in a constant state of flux, reflecting the dynamic nature of human behavior and technological progress. As we look to the horizon, it’s clear that integrating advanced computing techniques is not just beneficial but necessary. For instance, the application of machine learning algorithms has already shown significant potential in predicting psychological trends and behaviors.
The collaborative effort between data scientists and psychologists marks a crucial step forward. Multifaceted research approaches can lead to a more holistic understanding of psychological constructs and their application in real-world settings. This union not only enhances academic research but also lays a groundwork for innovations in therapeutic practices and consumer analysis.
In sum, as we continue to navigate this ever-evolving landscape, it will be essential to maintain a commitment to ethical standards and responsible data use to safeguard the integrity of research in psychology. The future is brighter when data and psychology harmonize, fostering a legacy of understanding, compassion, and innovation.
"True knowledge exists in knowing that you know nothing." - Socrates
This evolving relationship, framed within ethical considerations, can ultimately deliver insights that permanently alter our understanding of the human experience.