Exploring AI Face Reader Technologies and Their Impact
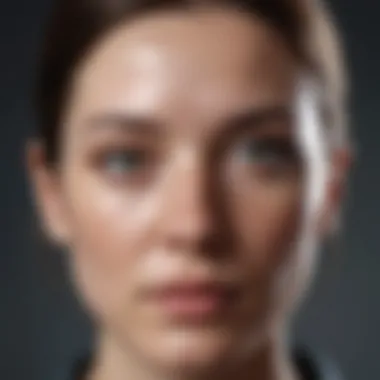
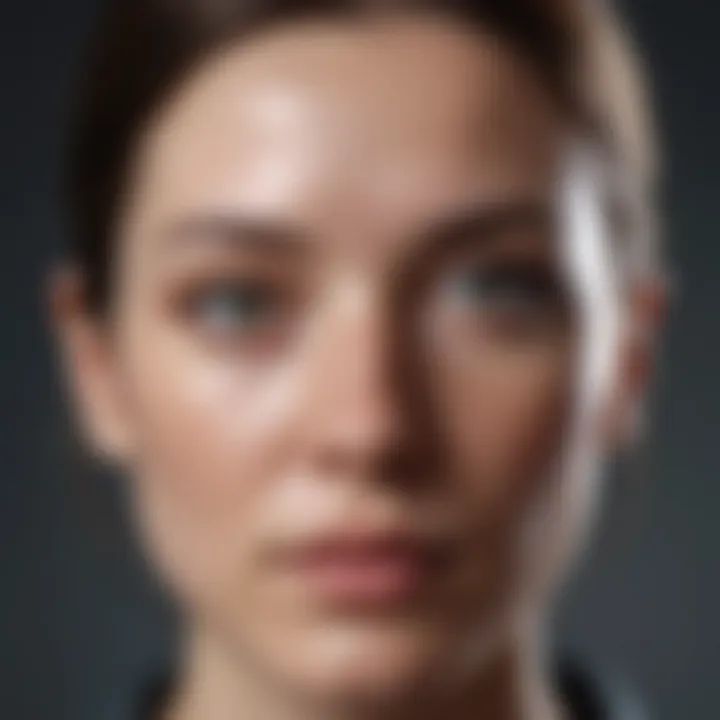
Intro
The world of artificial intelligence (AI) continues to evolve rapidly, affecting numerous domains and creating new opportunities and challenges alike. Among the areas gaining traction is AI face reader technology, a field that merges psychological insights with advanced computational techniques. This promising technology aims to decipher human emotions and traits based on visual cues detected in facial expressions. As we embark on this exploration, we will not only investigate how these systems function but also address their importance across various sectors, ranging from marketing to mental health.
The relevance of this topic stems from the ongoing integration of AI into our everyday lives. Companies leverage these technologies to tailor experiences, while researchers employ them to gain insights into human behavior. However, with great power comes great responsibility. Ethical dilemmas surrounding privacy and misuse loom large, making it crucial to examine both sides of the coin.
This article will present a thorough overview of the principles underlying AI face reader technologies, their practical applications, and the ethical considerations they provoke. Each facet will contribute to a deeper understanding of how these systems are reshaping society and influencing the direction of future innovations.
Get ready to navigate through a landscape where technology meets humanity, exploring both the innovative potential and the cautionary tales that come with it.
Prelims to AI Face Reading
The field of AI face reading has emerged as a pivotal area of study and application in recent years, capturing the attention of researchers, educators, and industry professionals. Its relevance spans across numerous sectors, making it a fascinating subject of exploration. As we increasingly integrate artificial intelligence into our daily lives, understanding how AI interprets human facial features can open doors to new innovations and improvements in various fields.
Definition and Scope of AI Face Reader
AI face readers, at their core, are systems designed to analyze and interpret human facial expressions through sophisticated software and algorithms. This technology aims to decode emotional states based on facial movements and structures. In practical terms, it means that a device can, say, determine if a person is happy, sad, or stressed by scrutinizing their facial cues. It’s worth noting that the scope does not end with emotions; these systems can also draw conclusions about age, gender, and sometimes even health conditions concerning the individual being observed.
The applications are as diverse as they are intriguing. In healthcare, AI face readers can assist in diagnostics by recognizing symptoms often evident in facial expressions. Meanwhile, businesses can leverage this insights for customer engagement, tailoring experiences that resonate on a deeper emotional level. When we consider privacy implications, however, we’re reminded that the deployment of such a technology comes with significant responsibilities.
Historical Development of Facial Recognition Technology
The journey toward today’s advanced AI face reading technologies has a rich history that dates back several decades. Initially, facial recognition was more of an academic pursuit, primarily used in laboratory settings for psychological studies and basic identification tasks. The early systems relied on primitive algorithms; they were far from accurate and often struggled with even mild variations in faces due to lighting and angles.
As computing power advanced in the 1990s and early 2000s, researchers began to refine facial recognition technologies significantly. One key milestone was the introduction of the Eigenfaces method, which utilized principal component analysis (PCA) to reduce the complexity of facial data. However, the algorithms still faced challenges, especially in identifying faces in uncontrolled environments.
The introduction of machine learning, particularly deep learning in recent years, marked a turning point. This shift allowed for training algorithms using vast datasets, enhancing their ability to recognize patterns and improve accuracy remarkably. With big data and improved image processing capabilities, AI systems could analyze not just static images but also live video feeds.
As the technology evolved, the debate surrounding its ethical use began to heat up, raising questions about privacy, consent, and accuracy in real-world applications.
From security systems employing facial recognition for crime prevention to smartphone technology incorporating face unlock capabilities, the evolution illustrates both the potential and the challenges faced by this innovative field. The historical context serves as a reminder to critically examine how we embrace these emerging technologies, ensuring that they are developed and used responsibly.
Fundamental Principles of AI Face Reading
Understanding the Fundamental Principles of AI Face Reading is essential for grasping how these sophisticated systems operate and the broader implications of their use. At its core, AI face reading blends advanced algorithms with vast amounts of data to interpret human emotions, identities, and behaviors. This intersection of technology and human expression raises numerous questions about both functionality and ethics. Let's delve into the critical components that underpin this technology.
Algorithmic Foundations
The algorithms that power face-reading technologies are the backbone of their functionality. Typically, these algorithms rely on machine learning and deep learning techniques, enabling them to autonomously refine their capabilities over time through exposure to more data. For example, convolutional neural networks (CNNs) serve as a primary construction piece in many facial recognition systems.
These networks mimic the way humans perceive visual information, breaking down images into layers for extraction and processing. By utilizing extensive datasets, such algorithms learn to identify minute variations in facial features, enabling algorithms to differentiate between numerous faces, even in challenging conditions. Not only does this enhance recognition accuracy, but it also enables real-time analysis and response.
Data Types and Inputs
The effectiveness of AI face-reading technologies hinges on the quality and variety of data fed into them. Various data types play a crucial role, including images, video feeds, and even three-dimensional models of faces. Raw pixel data collected from cameras is the starting point. However, the magic lies in the preprocessing stages.
For instance, data normalization and augmentation techniques are crucial in preparing images for analysis. Factors such as lighting conditions, angles, and expressions can dramatically affect recognition outcomes. Additionally, incorporating biometric data like eye movement or heart rate can provide a deeper context when interpreting emotional states.
- Sources of data include:
- Surveillance cameras
- Mobile devices
- Custom-built facial recognition systems
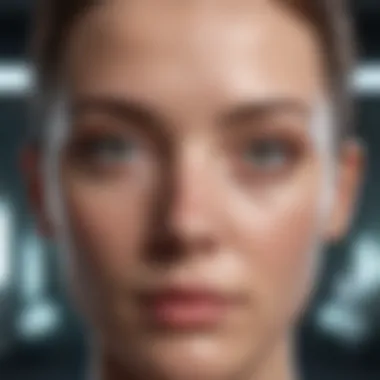
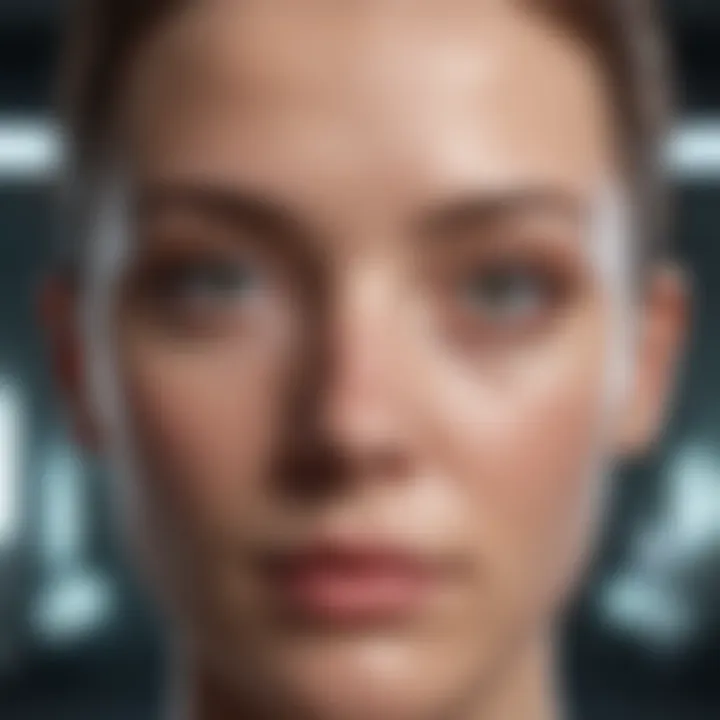
Each input source offers unique advantages and challenges, collectively shaping AI's understanding of human facial expression.
Feature Extraction Techniques
Feature extraction is another vital aspect of AI face reading. This process involves identifying and isolating key facial attributes to create a signature for recognition or emotional analysis. Effective feature extraction techniques focus on the essential elements that distinguish one face from another.
Facial landmarks like the position of the eyes, nose, and mouth are common focal points during this stage. Techniques like eigenfaces or local binary patterns help convert complex image data into manageable formats for easier interpretation by algorithms. Beyond just recognizing faces, advanced methods can gauge emotions through subtle changes in features like eyebrow shape or lip movement.
"Feature extraction is not merely about recognizing faces; it's about unlocking the emotional dialect they convey through expression."
In summary, the fundamental principles behind AI face reading technologies create a framework that merges human expression with advanced computational capabilities. As these technologies continue to evolve, understanding their key components will prove vital in navigating the various applications and implications they hold for society.
Applications in Various Sectors
The relevance of AI face reader technologies stretches across multiple fields, reflecting their transformative potential. From enhancing patient outcomes in healthcare to securing environments in public spaces, these technologies exhibit a wide range of applications. In this section, we will discuss key elements, benefits, and considerations surrounding these applications, providing a comprehensive overview of how AI face readers are reshaping various sectors.
Healthcare and Medical Diagnostics
In healthcare, AI face reader technology holds promise as a tool for improving diagnostics and patient care. With the ability to analyze facial expressions and features, AI systems can aid in detecting conditions such as depression or pain. For example, a recent pilot program in a children's hospital used facial recognition software to assess pain levels in young patients unable to articulate their discomfort. This real-time feedback helped medical professionals adjust treatment plans promptly.
The significance of this application cannot be understated. AI technologies can minimize human error, offer insights into patient emotions, and enable quicker interventions. However, it is crucial to approach these implementations with caution. The accuracy of diagnosing based solely on facial analysis must be validated through rigorous research, and considerations about patient privacy must be navigated carefully.
Security and Surveillance Systems
Insecurity domains, AI face reading technology is a double-edged sword. On one hand, it aids law enforcement in identifying individuals in crowded areas, potentially averting crimes before they happen. For instance, many airports worldwide have integrated facial recognition systems to speed up passenger processing and enhance security measures.
However, the same technology raises concerns related to privacy infringement and misuse. When facial recognition is deployed without strict guidelines or transparency, it can lead to surveillance overreach. Examining how law enforcement agencies use these tools is vital; conversations about regulations and ethical practices in this context cannot be overlooked.
Marketing and Consumer Behavior Analysis
In the marketing realm, AI face reader technologies are increasingly leveraged to decipher consumer behavior patterns. By interpreting facial expressions, brands can gauge customer reactions to products in real-time. Companies like Apple and Nike have started using these insights to enhance customer experiences and tailor marketing strategies effectively.
The advantages of this application include understanding emotional triggers that drive purchasing decisions. This can result in highly personalized marketing initiatives, which, according to recent studies, can improve customer engagement and loyalty significantly. Nevertheless, brands should tread lightly. Ethics in collecting and analyzing consumer data must be a priority, as misusing emotional data can lead to reputational harm and consumer backlash.
Personalized User Experiences
AI face reader technologies also play a pivotal role in crafting personalized user experiences across various digital platforms. From dating apps to e-commerce websites, businesses are now harnessing facial recognition to adjust content and suggestions based on real-time user expressions.
Imagine scrolling through a movie app; the software picks up on your cues of disinterest or excitement, recommending films accordingly. This level of personalization can significantly enhance user satisfaction. Still, similar to previous applications, users must be aware of how their data is being utilized. Transparency and consent are paramount in fostering a trustworthy relationship with consumers.
Technical Limitations of AI Face Reading
When hatching an understanding of AI face reading, acknowledging its technical limitations is crucial. These limitations can impact efficacy and trust of the technology. While these systems bring forward ground-breaking innovations, it’s necessary to tread carefully and comprehend the underlying challenges that come with them. Exploring bias, demographic challenges, and environmental factors can help in grasping both the potential and the pitfalls of these technologies.
Bias and Accuracy Issues
Bias within AI systems has drawn increasing attention over the past few years. Essentially, biases arise when the data sets used to train AI algorithms do not adequately represent the diversity of human faces. This leads to discrepancies in how accurately faces are recognized based on characteristics like race, gender, and age. For instance, studies have shown that some facial recognition systems exhibit a greater error rate for darker skin tones compared to lighter ones. Even more troubling, a report from the National Institute of Standards and Technology highlighted that face recognition algorithms were almost 100% more likely to misidentify African-American women than white men.
This bias not only leads to accuracy issues but also risks serious repercussions. Misidentifications can easily lead to wrongful accusations or missed opportunities in various sectors, especially in law enforcement and hiring processes. Stakeholders must prioritize data inclusivity to mitigate harm and enhance the technology's adaptability.
Challenges in Diverse Demographics
Different demographic scenarios require a nuanced understanding of human facial characteristics. Factors such as cultural expressions, variations in facial structures, and environmental contexts can skew the performance of AI face reading systems. When deployed across varied geographical locations, these systems might misinterpret cultural signals or emotional expressions. For example, the way a smile is interpreted can differ vastly between cultures, yet the AI may lack this cutural nuance.
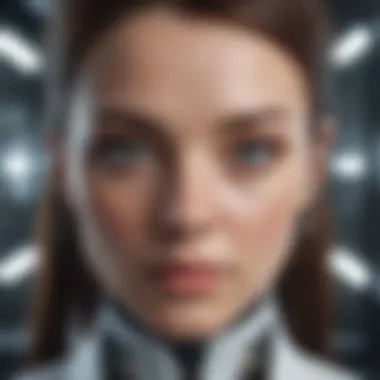
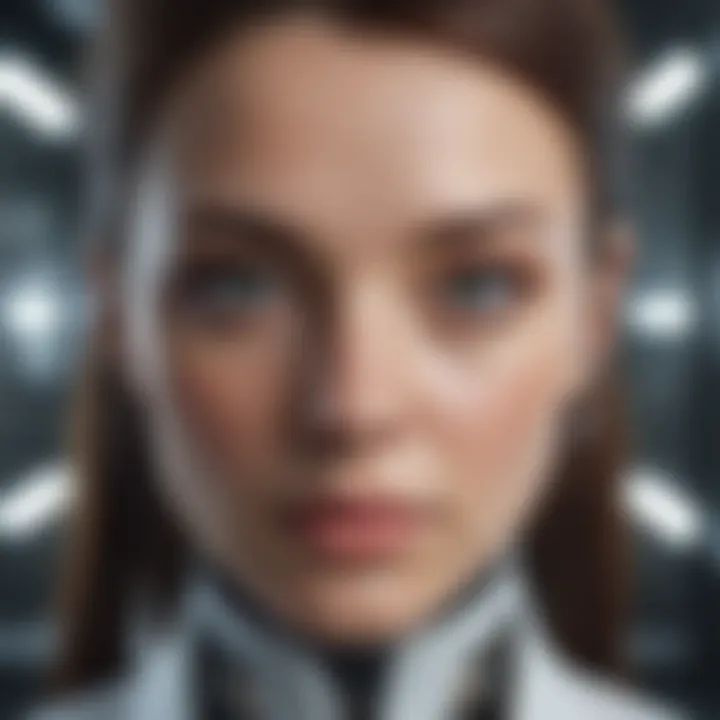
The limitations become evident in practical applications:
- Healthcare: An algorithm misreading the emotions of a patient due to cultural differences could lead to inappropriate medical responses.
- Marketing: Brands employing facial recognition in advertising could fail to engage effectively with diverse consumer bases.
Tackling these challenges is not a one-size-fits-all task. Developers must dive into local specifics and adjust systems accordingly, ensuring they are relevant and accurate in different demographics.
Environmental Influences on Performance
AI face recognition can often be more finicky than it appears on the surface. Environmental factors play a big role in performance. Lighting conditions are one of the primary hurdles. Consider this: a well-lit environment can work wonders for facial recognition, while dim or overly bright conditions can create havoc. Low-light settings, think nighttime outside events or poorly lit rooms, can obscure facial features making accurate reading increasingly complex.
Additionally, background noise, such as distortion or reflections, can confuse systems. Face recognition is supposed to be as crisp as a well-prepared report, yet with environmental noise, its performance becomes akin to deciphering a garbled message.
To conclude, understanding and addressing these environmental challenges is paramount. Training models on diverse datasets, increasing adaptability, and recognizing specific environmental variables go a long way in improving the efficiency of AI face reading technologies. Efforts here can significantly enhance the technology's practicality and effectiveness, ultimately leading to a more robust application in various fields.
Ethical Implications of AI Face Reader Use
As society increasingly adopts AI face reader technologies, understanding the ethical implications surrounding their use becomes crucial. These systems, while offering significant benefits in a variety of sectors, also pose serious questions about privacy, consent, and regulation. Engaging with these issues is vital for ensuring that technological advancements do not overshadow fundamental human rights and societal norms.
Privacy Concerns
Privacy stands at the forefront of ethical debates regarding AI face readers. As they integrate more into daily life, questions arise about who is watching whom. The ability of these systems to analyze facial expressions and identity can lead to pervasive surveillance, where individuals feel like they are constantly under scrutiny. Different regions have varying approaches to privacy laws, which can complicate how these technologies are deployed.
For instance, if facial recognition technology is used in public spaces without proper safeguards, it can infringe on individual privacy and personal boundaries. Concerns about data breaches or unauthorized access to facial data - a deeply personal identifier - further amplify these worries. It’s essential for discussions on privacy to focus on establishing clear guidelines and protocols that protect individuals from misuse.
Consent and Data Ownership
The issues of consent and data ownership are intertwined with privacy issues, yet they warrant their own deliberation. With the collection of facial data comes the question of whether individuals have truly consented to their likeness being captured and analyzed. Often, data is harvested in ways that aren't fully transparent, leaving users in the dark regarding how their information will be used.
Moreover, who owns the data once it’s collected? Is it the individual, the organization that collects it, or the developers who create the algorithms? This murky territory requires a solid framework. Establishing clear consent mechanisms and ownership rights is imperative. One avenue could be developing standards that require explicit consent from individuals before their facial data is recorded or analyzed.
Regulatory Frameworks and Compliance
Regulatory frameworks play a significant role in ensuring ethical compliance with AI face reader technologies. Currently, many countries are grappling with the rapid pace of technological advancements, leading to a patchwork of regulations that may not adequately address the complexities involved.
Effective regulations should aim to balance innovation with ethical considerations. They might include:
- Defining clear boundaries for the application of face recognition technology.
- Mandating transparency in how systems operate and make decisions.
- Ensuring accountability for organizations that misuse or mishandle data.
Furthermore, ongoing compliance assessments can help organizations remain aligned with established guidelines while adapting to new challenges as technologies evolve. Only through robust regulatory frameworks can the use of AI face readers align with the ethical standards expected of modern technology.
"In the absence of stringent regulations and frameworks, we risk losing sight of our ethical obligations as we integrate AI more deeply into society."
In summary, the ethical implications of AI face reader technologies are vast and multifaceted. Addressing privacy, consent, and regulation is not just a technical requirement; it reflects our commitment to uphold human rights in an increasingly digital age.
Future Directions in AI Face Reading
The realm of AI face reading technologies is on the cusp of significant evolution. As the industry continues to advance, understanding the pathways it may take is paramount. It holds not just potential for further accuracy but also opens avenues for multidisciplinary applications. The aspects discussed in this section will highlight the nuances involved, from enhanced machine learning techniques to its possible implications on society at large.
Advancements in Machine Learning Techniques
The significance of machine learning in AI face reading cannot be overstated. As developers harness the power of algorithms that learn from data, the accuracy and efficiency of facial recognition tools improve drastically. Advanced deep learning architectures, such as convolutional neural networks (CNNs), are leading the charge in recognizing patterns in facial images that the human eye may overlook.
New methods, including transfer learning, allow models trained on vast datasets to adapt quickly to smaller, more specific datasets with minimal retraining. This adaptability is invaluable in improving recognition systems, particularly in diverse demographic contexts. Imagine a world where even the nuances of micro-expressions can be interpreted with precision, enhancing applications across security, mental health diagnostics, and marketing. This evolution isn't just about enhancing existing techniques; it is also about fostering innovation in how these technologies are developed and deployed.
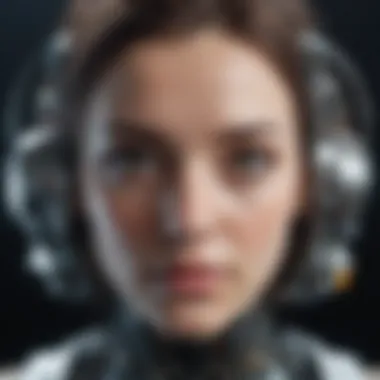
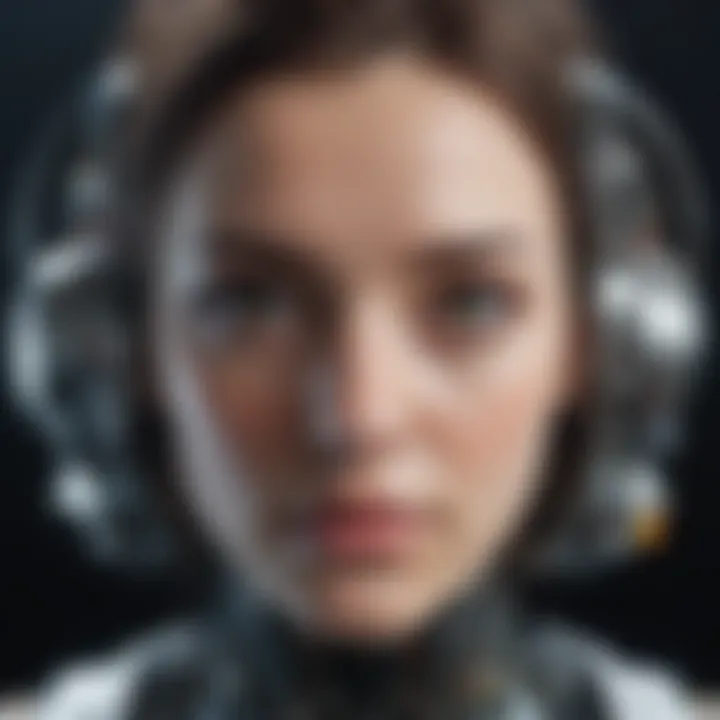
Integration with Other AI Technologies
The integration of AI face reading with other AI technologies paves the way for groundbreaking innovations. When combined with natural language processing (NLP), for instance, AI systems can leverage facial cues to interpret emotional states, tailoring interactions accordingly. A simple chat interface could become a personal therapist, analyzing users' expressions while conversing to offer insights or emotional support.
Additionally, coupling face reading with Internet of Things (IoT) devices may reshape user experiences in smart homes or offices. Picture a smart home that adjusts lighting based on the occupants’ emotional states as inferred by facial recognition. Key benefits of such integrations include:
- Enhanced user personalization
- Improved security protocols
- Greater accessibility for disabled individuals
- More effective mental health monitoring systems
Potential Societal Transformations
Looking further afield, the impact of advancements in AI face reading technology could manifest in significant societal transformations. The ability to derive insights from facial cues can help build bridges in communication, especially in intercultural contexts. Increased understanding fostered by accurate sentiment analysis could reduce misunderstandings and conflict.
However, alongside these benefits lie serious considerations. Societal norms around privacy, emotional expression, and consent will inevitably evolve. Regulations must be developed that address the ethical complexities emerging from these technologies.
"The advancement in AI face reading will not just reshape industries; it will redefine how humans interact with machines and, ultimately, with each other."
In summary, the future of AI face reading technologies hinges on the intertwining of cutting-edge machine learning advancements, holistic integration with other AI technologies, and the potential societal shifts that may arise from their widespread adoption. Each of these elements will shape the industry and influence standards of ethical and responsible usage.
Case Studies of AI Face Reader Implementation
The exploration of case studies in AI face reader implementations provides vital insights into how these technologies are applied in real-world scenarios. Analyzing success stories and setbacks not only showcases the potential benefits of AI face reader systems but also highlights crucial elements such as ethical considerations, technical challenges, and user acceptance. Understanding these nuances enables researchers and practitioners to navigate the complexities of this emerging technology more effectively.
Real-World Applications in Law Enforcement
In recent years, law enforcement agencies have increasingly turned to AI face reader technologies to enhance their investigative capabilities. One noteworthy example is the use of the software by police departments in major cities like Chicago and Los Angeles. These departments have implemented facial recognition systems to assist in solving crimes and identifying suspects. By comparing facial features captured on surveillance cameras with mugshot databases, law enforcement can rapidly locate individuals wanted for crimes.
However, the implementation is not without controversy. The benefits of improving public safety are weighed against concerns about invasions of privacy and potential bias against marginalized communities. The accuracy of the technology often hinges on the quality of the data used for training, exposing issues inherent in the demographic diversity of the training sets. For instance, studies have shown that facial recognition systems tend to struggle with accurately identifying individuals from non-Caucasian backgrounds. This calls for a more nuanced approach to deployment, ensuring that systemic biases do not exacerbate existing social inequalities.
Success Stories in Healthcare
Turning to the healthcare sector, AI face reader technologies have proven beneficial, particularly in patient management and diagnostics. One prominent case is the use of facial recognition in assessing patients with neurological conditions. For example, some hospitals have employed machine learning algorithms to analyze facial expressions indicative of specific conditions, such as autism or Parkinson’s disease. This innovative approach not only streamlines diagnostics but also fosters personalized healthcare plans.
An example that stands out is the partnership between a leading medical research institute and a tech company to develop a tool that recognizes early signs of emotional distress in children. By analyzing facial cues, clinicians can intervene sooner and tailor treatment programs effectively. As these success stories unveil more possibilities, they also indicate a need for strict ethical guidelines in patient data management and consent.
Challenges Highlighted by Failed Implementations
Despite promising applications, AI face reading technologies have not always seen smooth sailing. Failed implementations serve as cautionary tales, illustrating the limitations and risks associated with relying on this technology. Notable incidents have occurred where systems malfunctioned, leading to wrongful accusations and misidentifications, often fueled by an insufficient understanding of how these systems operate.
One high-profile example emerged when a facial recognition system was rolled out in a New York City subway system. Initially designed to enhance security, the system faced backlash after a series of false positives resulted in wrongful detentions. The public outcry raised significant ethical issues regarding accountability and transparency. The aftermath emphasized the necessity for robust testing protocols and comprehensive audits prior to any large-scale deployment of AI technologies.
By dissecting these varied experiences, it’s clear that while AI face reader implementations can lead to breakthroughs in diverse fields, they must be approached with care in design, execution, and ethical consideration. This holistic understanding can chart a safer, more effective path forward as the technology continues to evolve.
Finale and Recommendations
In wrapping up our examination of AI face reading technologies, it is crucial to recognize their profound significance in modern society. These systems offer innovative ways to enhance various sectors, from healthcare to security, yet they come with a set of challenges and ethical considerations that cannot be overlooked. The intersection of technology and human interaction raises questions that merit serious attention, such as privacy, data ownership, and societal implications.
Summary of Key Findings
After an extensive look into the workings and applications of AI face reading, a few key findings stand out:
- Technological Advancement: The evolution of machine learning and data processing has significantly improved the accuracy and efficiency of face-reading systems.
- Diverse Applications: These technologies are making inroads in multiple sectors, including law enforcement, marketing, and healthcare. For instance, their use in identifying medical conditions by analyzing facial expressions holds a promise for future diagnostics.
- Ethical Considerations: There are pressing concerns regarding bias in data, privacy issues, and the lack of clarity on data ownership. Regulatory frameworks are still playing catch up in many cases, which can result in misuse or overreach.
- Limitations and Challenges: Despite the benefits, there are still pitfalls. Environmental factors, demographic diversity, and inherent algorithm biases can undermine the effectiveness of AI face reader technologies.
Future Research Directions
To fully leverage the benefits of AI face reading, future research should focus on several key areas:
- Bias Reduction: Investigating methods to identify and mitigate biases within datasets to ensure equitable performance across varied demographics.
- Regulatory Framework Development: Advocating for the establishment of robust regulatory structures that protect privacy while promoting innovation.
- User Education and Training: Developing programs that educate users about the implications of detecting and utilizing facial data can empower individuals to make informed decisions about their privacy.
- Integration with Other Technologies: Analyzing how AI face reading can work in tandem with other emerging technologies, such as voice recognition or behavioral analytics, to create more holistic user experiences.
- Longitudinal Studies: Conducting long-term studies that assess both societal impacts and technological effectiveness over time can lead to better methodologies and applications.
In summary, while AI face reading technologies promise substantial advancements in many fields, a balanced approach that prioritizes ethical implications, privacy, and diversity is vital for sustainable development in this area. Future investigations must delve deeper into these aspects to guide the responsible evolution of this powerful technology.