Understanding RNA Sequencing: Purpose and Implications
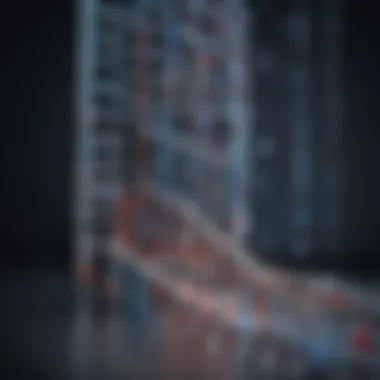
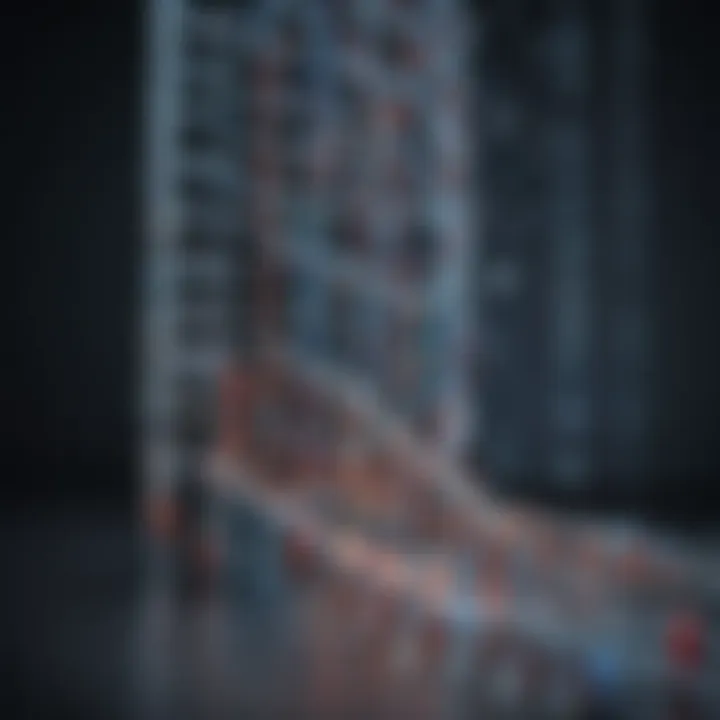
Intro
RNA sequencing, often abbreviated as RNA-seq, has emerged as a pivotal technology in the field of molecular biology. It offers a profound insight into the complexities of gene expression, enabling researchers to investigate how genes are turned on or off in response to a myriad of biological stimuli. This process involves capturing the entire transcriptome, which includes all the messenger RNA molecules within a cell at a given time. As a result, RNA-seq not only sheds light on gene activity but also reveals intricate details about cellular functionality.
Understanding the purpose of RNA sequencing goes beyond mere academic interest. It has vast implications across various fields, including developmental biology, cancer research, and personalized medicine. By unraveling how genes interact with each other and respond to environmental changes, RNA-seq is a powerful tool that enhances our comprehension of health and disease.
As the following sections will elucidate, RNA sequencing involves specific methodologies and approaches that enhance the depth of insights gained from genetic data. This article aims to explore those methodologies along with the challenges that accompany RNA-seq projects. We will also take an in-depth look at how data analysis methods are evolving, ultimately allowing for advancements in precision medicine and genomics.
Research Overview
Summary of Key Findings
The exploration of RNA sequencing reveals several critical findings:
- RNA-seq can identify not just gene expression levels but also splice variants, giving insights into alternative splicing events.
- This technology supports applications in identifying biomarkers for diseases, particularly in complex diseases like cancer.
- RNA-seq proves essential in understanding the impact of drugs on cellular pathways, paving the way for novel therapy strategies.
Research Objectives and Hypotheses
The primary objectives of this article focus on:
- Elucidating the methodologies employed in RNA sequencing to provide a clear understanding of the processes involved.
- Addressing the challenges faced in RNA-seq projects and how these can affect outcomes.
- Discussing the implications of RNA-seq technology in the realm of personalized medicine and genomics.
The hypotheses posited include:
- RNA sequencing provides a more comprehensive view of gene expression compared to traditional methods.
- The evolution of data analysis techniques will make RNA-seq data increasingly accessible and interpretable for diverse applications.
Methodology
Study Design and Approach
For a thorough understanding, a qualitative approach is employed to explore the current landscape of RNA sequencing. This investigation focuses on literature reviews, case studies, and expert consultations to gather a wide spectrum of insights into RNA-seq applications.
Data Collection Techniques
Data collection for this article involves synthesizing information from reputable sources, including published research articles, reputable websites, and scholarly databases. Key resources include Wikipedia, Britannica, and community insights from various platforms such as Reddit and Facebook. By integrating findings from multiple channels, this article provides a nuanced discussion of RNA sequencing.
Preamble to RNA Sequencing
RNA sequencing, or RNA-seq, has emerged as a crucial technique in the field of molecular biology due to its ability to provide detailed insights into gene expression and RNA dynamics. It serves as an invaluable tool not only for academic researchers but also for medical professionals and industry experts. RNA sequencing enables a comprehensive understanding of how genes are expressed in various conditions, which underpins many biological processes and disease mechanisms.
The importance of RNA sequencing lies in its multifaceted applications. Whether it is profiling gene expression in cancer research, identifying novel transcripts in developmental biology, or analyzing environmental response genes, RNA-seq is at the forefront. Precision in measuring RNA expression levels helps in devising targeted therapeutic interventions in personalized medicine.
Furthermore, RNA-seq offers enhanced sensitivity and resolution compared to traditional methods, allowing the detection of low-abundance transcripts and splice variants. As such, it is a potent alternative to older hybridization-based techniques. In this section, we will delve into the definition and historical context of RNA sequencing, laying the foundation for understanding its core purposes and applications.
Definition and Overview
RNA sequencing is a next-generation sequencing technique that captures and sequences the transcriptome of a given sample. The transcriptome represents all the RNA molecules expressed in specific cells or tissues under defined conditions. By utilizing this technology, researchers can quantify gene expression levels and obtain insights into RNA structure and function.
One of the key features of RNA-seq is its ability to provide a qualitative and quantitative overview of the transcriptome. It not only detects known transcripts but also identifies previously unknown RNAs, splicing patterns, and post-transcriptional modifications. With these capabilities, RNA-seq offers a depth of information that traditional methods do not provide. This approach facilitates a comprehensive understanding of how RNA transcripts correlate with biological functions and responses.
Historical Context and Development
The path to the current state of RNA sequencing began with the advent of first-generation sequencing technologies in the late 1970s. However, the real transformation occurred in the 2000s with the development of next-generation sequencing platforms. This innovation allowed for massively parallel sequencing, resulting in a drastic reduction in costs and time needed to sequence RNA.
In 2008, the first major RNA-seq publications emerged, highlighting its potential for high-throughput gene expression analysis. Since then, advancements in sequencing technologies have exponentially increased the resolution and capabilities of RNA-seq. The integration of bioinformatics tools has further enhanced data analysis, making it easier for researchers to interpret complex datasets.
As RNA sequencing continues to evolve, its relevance in biomedical research grows, driving forward innovations in various disciplines. Understanding its historical context helps underscore how RNA-seq has become a pivotal method for understanding gene expression patterns, ultimately shaping our approach to research and medicine today.
Core Purpose of RNA Sequencing
RNA sequencing (RNA-seq) serves several essential purposes within molecular biology research. Its value lies in its ability to provide deep insights into the functioning of genes. By analyzing the RNA present in cell samples, researchers can draw conclusions about gene activity under various conditions. This capability has made RNA-seq a fundamental tool in understanding biological processes like development, disease progression, and cellular responses to stimuli.
Gene Expression Profiling
Gene expression profiling is one of the primary purposes of RNA sequencing. It allows researchers to quantify the levels of mRNA within a cell, thus reflecting which genes are active at a given moment. This information can help in comparing gene expression across different conditions, tissues, or even species. For instance, in cancer research, scientists can identify which genes are over- or under-expressed in tumor cells compared to normal cells.
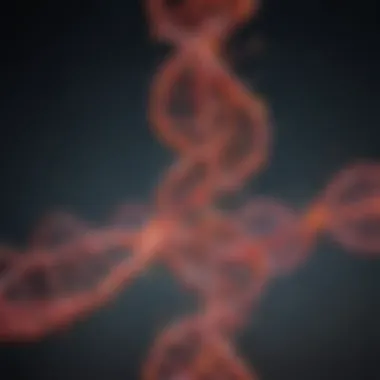
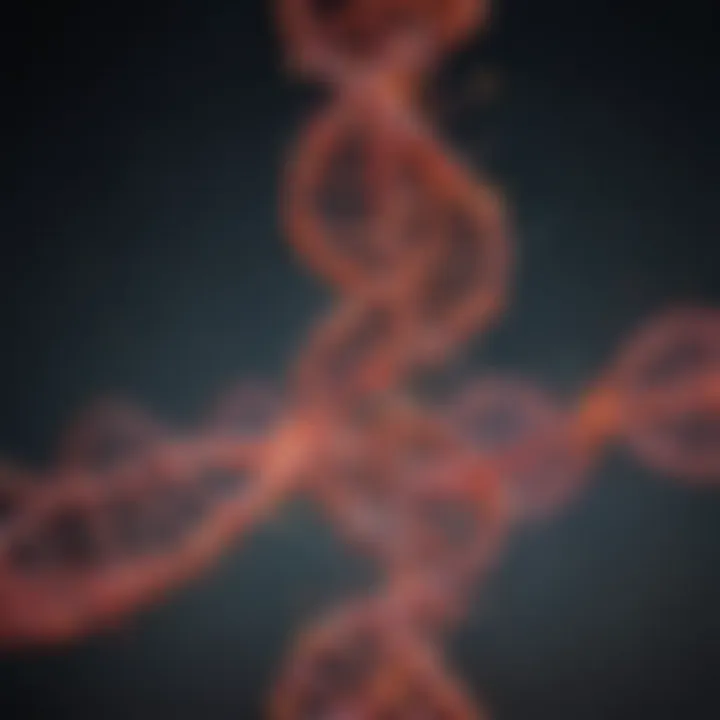
Key benefits of gene expression profiling include:
- Identification of biomarkers: Establishing key markers for diseases and conditions.
- Understanding disease mechanisms: Offering clarity on how genes interact in pathological states.
- Guiding therapeutic strategies: Aiding in the development of targeted treatments based on expression patterns.
Identification of Novel Transcripts
Another significant aspect of RNA sequencing is its ability to identify novel transcripts. Traditional gene annotation based on DNA sequence often misses new RNA molecules that may not fit established categories. RNA-seq can reveal previously uncharacterized non-coding RNAs or alternative isoforms of known genes. This identification can lead to new insights into gene functionality and evolution.
The importance of identifying novel transcripts lies in:
- Expanding gene catalogs: Building a more comprehensive repository of genetic information.
- Understanding genetic diversity: Allowing researchers to explore variations and effects on phenotypes.
Discovery of Splice Variants
RNA sequencing excels at discovering splice variants. Alternative splicing is a process where a single gene can lead to multiple proteins. RNA-seq can capture the complexity of splicing events and quantify different isoforms. This understanding has crucial implications for both basic research and clinical applications.
Understanding splice variants helps:
- In elucidating protein functions and interactions.
- In diagnosing diseases linked to splicing errors, contributing to personalized medicine developments.
Exploration of Regulatory Mechanisms
The exploration of regulatory mechanisms is also a core purpose of RNA sequencing. By examining RNA profiles under different conditions, researchers can infer the roles of regulatory sequences and proteins. This can unveil the controls behind gene expression and reveal how cells react to external signals.
Insights gained from these data can lead to:
- Uncovering gene regulatory networks: Mapping out how different components interact to control gene activity.
- Identifying potential targets for therapy: Leading to innovative strategies in drug design.
RNA sequencing is not just a tool for measurement; it is a gateway to understanding the intricate dance of life at a molecular level.
Applications of RNA Sequencing
RNA sequencing has emerged as a powerful tool in molecular biology, enhancing our comprehension of biological processes and disease mechanisms. The various applications of RNA sequencing illustrate its importance in multiple fields of research, allowing for advancements in understanding complex biological systems and facilitating the development of innovative strategies for diagnosis and treatment.
Cancer Research
One of the most significant applications of RNA sequencing is in cancer research. Traditional approaches for studying cancer often focused solely on DNA mutations. However, RNA sequencing allows researchers to assess gene expression levels, which can uncover insights into how certain genes contribute to cancer progression. By analyzing the RNA profiles of tumors, scientists can identify specific gene signatures that distinguish between different types of cancer. This is crucial for developing targeted therapies and personalized treatment plans.
Key Points in Cancer Research with RNA-seq:
- Identification of Biomarkers: RNA-seq can reveal upregulated or downregulated genes that may serve as potential biomarkers for diagnosis and prognosis.
- Understanding Tumor Microenvironment: RNA sequencing helps in studying the interactions between tumor and immune cells, advancing the knowledge of tumor microenvironments.
- Tracking Treatment Response: By observing how gene expression changes in response to treatment, RNA-seq can aid in monitoring efficacy of therapies.
The ability to analyze RNA changes not only informs cancer genetics but also enhances therapeutic strategies.
Developmental Biology
In developmental biology, RNA sequencing plays a critical role in understanding the underlying processes that dictate organism development. Researchers utilize this technique to analyze how gene expression changes during different stages of development. This can reveal key regulatory mechanisms and pathways involved in the formation of various tissues and organs.
Benefits of RNA-seq in Developmental Biology:
- Temporal Dynamics: RNA sequencing can capture dynamic gene expression changes across developmental time points, providing insights into crucial developmental transitions.
- Cell Type Identification: It allows scientists to precisely identify different cell types present in a developing organism, advancing the understanding of lineage specification.
- Gene Regulatory Networks: The data generated helps to elucidate networks of interactions that regulate developmental processes, enhancing our grasp of biology.
Comparative Transcriptomics
Comparative transcriptomics is an essential application of RNA sequencing that enables scientists to compare gene expression profiles across different species or conditions. By examining similarities and differences at the transcriptome level, researchers can draw conclusions about evolutionary relationships and functional adaptations.
Advantages of Comparative Transcriptomics:
- Evolutionary Insights: Comparative analyses can identify conserved genes and regulatory elements, shedding light on evolutionary processes.
- Functional Annotation: Such studies help in annotating gene functions by understanding how similar genes can exhibit varied expression patterns across species.
- Adaptation Mechanisms: Researchers can investigate how different organisms adapt to their specific environments, leading to discoveries in ecology and evolutionary biology.
Environmental Response Studies
RNA sequencing also finds extensive use in environmental science, where it is applied to study how organisms respond to environmental changes. This includes understanding the transcriptional responses to stressors such as temperature shifts, pollutants, and other ecological pressures.
Key Contributions of RNA-Seq for Environmental Studies:
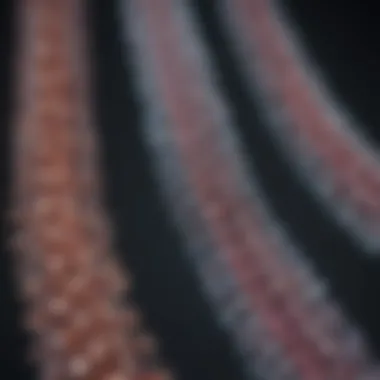
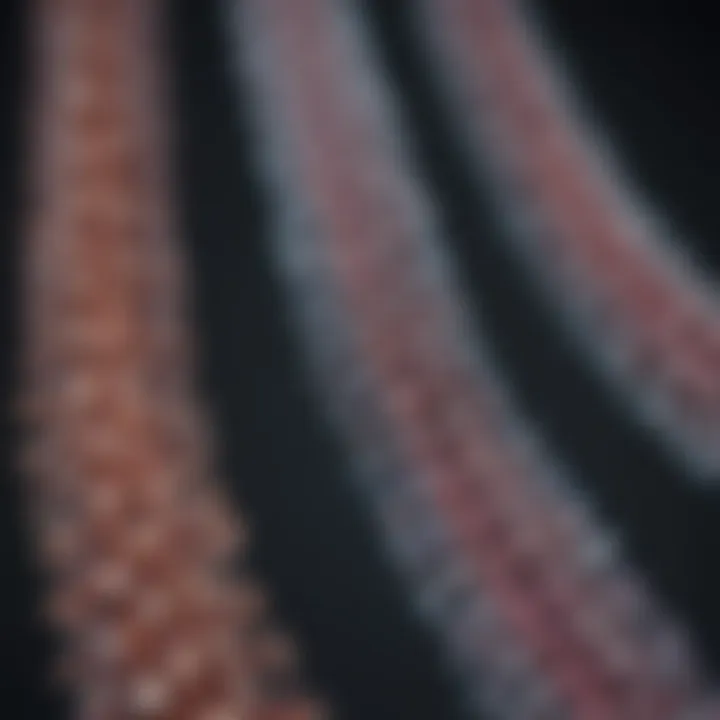
- Stress Response Mechanisms: It enables a detailed assessment of how organisms activate specific pathways in reaction to environmental stress, providing insights into their survival strategies.
- Biodiversity Assessment: RNA sequencing can help in cataloging the diversity of microbial communities and their functional capabilities in various ecosystems.
- Conservation Biology: Understanding gene expression under stress conditions aids conservation efforts, informing strategies to protect endangered species.
In summary, the applications of RNA sequencing extend far beyond just understanding gene expression. It plays a pivotal role across various fields including cancer research, developmental biology, comparative transcriptomics, and environmental response studies. By leveraging this technology, researchers are equipped to tackle complex biological questions, paving the way for advancements across multiple disciplines in molecular biology.
Methodological Approaches to RNA Sequencing
The methodological approaches to RNA sequencing are fundamental to the overall success and reliability of the data generated. Different stages in the RNA sequencing processβranging from sample preparation to the sequencing itselfβdetermine the quality and interpretability of the results. Each methodological element plays a significant role in elucidating gene expression and other molecular mechanisms. When strategizing RNA-seq experiments, researchers must consider factors such as sample type, desired depth of coverage, and the specific questions they aim to answer.
Sample Preparation Techniques
The initial step of RNA sequencing involves sample preparation, which is crucial for obtaining high-quality RNA. Proper collection and preservation methods are essential to prevent RNA degradation. Techniques often used include the use of TRIzol reagent or RNeasy kits to extract RNA from various biological samples.
- Quality Control: It is vital to assess RNA quality before proceeding with further steps. The Agilent Bioanalyzer or similar systems can quantify RNA integrity and ensure suitability for sequencing.
- Depletion vs. Enrichment: Depending on the research goals, whether to deplete ribosomal RNA or enrich for specific RNA populations must be considered. Depletion removes unwanted ribosomal RNA, increasing the detection sensitivity of low-abundance transcripts.
Library Construction Methods
Once the RNA is prepared, constructing a sequencing library is the next step. This process involves converting RNA into cDNA (complementary DNA). This step introduces specific adapters that are critical for sequencing.
- Methods of Library Construction: Researchers can select from several methods, including:
- PolyA selection: Targets mRNA with polyadenylated tails.
- rRNA removal: Ensures only the transcripts of interest are sequenced.
- Stranded vs. unstranded libraries: Stranded libraries retain the information about the original strand, which can be vital for interpreting gene expression accurately.
Sequencing Technologies
Advancements in sequencing technologies have significantly enhanced RNA-seq methods. Various platforms, such as Illumina, Ion Torrent, and PacBio, offer unique advantages and challenges.
- Illumina Sequencing: This method is the most widely used due to its cost-effectiveness and high throughput capability. It generates millions of short reads, ideal for gene expression analysis.
- Long-read Sequencing: Platforms like Pacific Biosciences and Oxford Nanopore provide longer reads, facilitating the discovery of splice variants and structural variants more effectively. This approach is advantageous for analyzing complex events in RNA transcripts.
Analysis of RNA Sequencing Data
The analysis of RNA sequencing data is crucial in today's molecular biology. This section aims to highlight the key stages and considerations involved in effectively understanding the data produced from RNA-seq experiments. Addressing this topic is essential, as accurate data analysis can directly influence research outcomes, leading to important biological insights. The steps involved in data analysis can be grouped into three main areas: preprocessing raw data, quantification of gene expression, and statistical approaches in RNA-seq.
Preprocessing Raw Data
Preprocessing is a fundamental step in RNA-seq data analysis. Raw sequencing reads often contain various artifacts, such as adapter sequences, low-quality bases, and PCR duplicates that can distort the final results. Before any meaningful analysis can occur, it's critical to ensure that the raw data is clean and reliable.
Key activities during preprocessing include:
- Quality Control: Assessing the quality of the sequencing reads using tools like FastQC helps researchers identify potential problems early in the analysis.
- Trimming: Removing low-quality bases and adapter sequences using software such as Trimmomatic ensures that only high-quality sequences are retained for further analysis.
- Filtering: Discarding reads that do not meet specific quality thresholds enhances the integrity of the data further.
By meticulously preprocessing the raw data, researchers lay a solid foundation for subsequent analysis, which is vital for accurate gene expression measurement.
Quantification of Gene Expression
Quantification of gene expression is a pivotal next step following preprocessing. This phase focuses on determining the abundance of RNA transcripts from the sequencing data. Accurate quantification is essential not only for understanding gene function but also for exploring how gene expression varies under different conditions.
There are various methods employed in quantifying gene expression, including:
- Count-Based Methods: These techniques estimate transcript levels by counting the number of reads that map to each gene. Tools like HTSeq and featureCounts are often utilized here.
- Normalization: To make the expression levels comparable across samples, normalization methods, such as the TPM (Transcripts Per Million) or RPKM (Reads Per Kilobase of transcript per Million mapped reads), adjust for differences in sequencing depth and gene length.
Overall, the quantification process is integral to interpreting the data meaningfully, allowing for the identification of differentially expressed genes under various experimental conditions.
Statistical Approaches in RNA-seq
The final aspect of RNA sequencing data analysis involves applying statistical methods to validate findings and interpret the results accurately. This phase is critical as it enables researchers to draw significant conclusions from their data.
Several statistical approaches are often used in RNA-seq analysis:
- Differential Expression Analysis: Tools like DESeq2 and EdgeR are designed to identify genes that are significantly differentially expressed across conditions. They use models that account for biological variability, ensuring reliable results.
- Multiple Testing Correction: Since many genes are tested simultaneously, methods like the Benjamini-Hochberg procedure help control false discovery rates. This process is crucial for maintaining the reliability of any identified differentially expressed genes.
- Cluster Analysis: Researchers often employ clustering algorithms to group genes with similar expression patterns, revealing insights into co-regulation or shared functions.
Statistical methodologies bring rigor to RNA-seq analysis, allowing researchers to confidently interpret biological significance and draw broader conclusions from their findings.
In summary, analysis of RNA sequencing data consists of several critical stepsβeach plays a vital role in ensuring the integrity and interpretability of results. By focusing on preprocessing raw data, quantifying gene expression, and applying proper statistical frameworks, researchers can extract meaningful insights from their RNA-seq experiments, thereby advancing the field of molecular biology.
Challenges in RNA Sequencing Studies
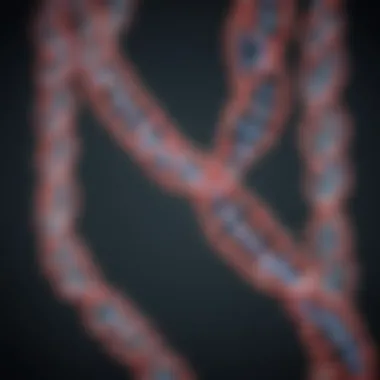
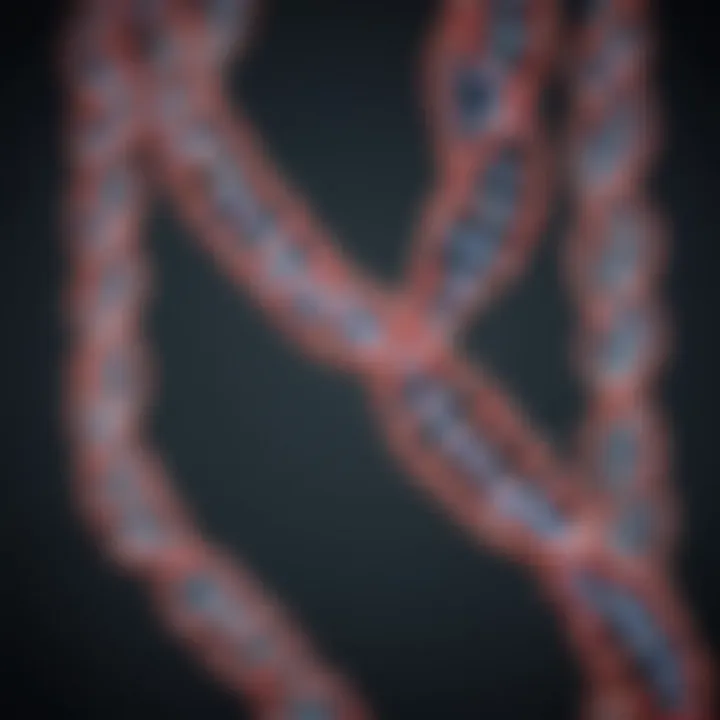
RNA sequencing, while revolutionary, does not come without its set of challenges. These challenges can significantly impact the accuracy and reliability of results derived from RNA-seq experiments. Understanding these limitations is crucial not only for improving data quality but also for guiding future research in the field.
Technical Limitations
One major challenge in RNA sequencing lies in the technical limitations of the technologies used. Several factors contribute to this issue:
- Sequencing Depth: Insufficient sequencing depth can lead to underrepresentation of lowly expressed genes, skewing the overall gene expression profile. Not capturing these genes may ignore critical biological information.
- Read Length: Different technologies offer varying read lengths, which can affect the assembly of transcripts, particularly for complex species with large genomes. Short reads might fail to adequately characterize isoforms and structural variants.
- Contamination: Sample contamination during preparation or sequencing can introduce noise into the dataset. This contamination complicates downstream analyses, making it difficult to ascertain true biological signals.
These technical limitations necessitate a careful approach to design and execution of RNA-seq experiments. Ensuring optimal sequencing depth and minimizing contamination are essential steps in this regard.
Biological Variability
Biological variability presents another layer of complexity in RNA sequencing studies. This variability can arise from multiple sources:
- Inter-sample Variability: Differences in gene expression levels among samples due to biological factors such as age, diet, or environmental exposure can complicate comparisons and interpretations.
- Intra-sample Variability: Changes within a single sample over time or across different cells contribute to inconsistencies, making it essential to carefully replicate experiments and control for these variations.
- Tissue Specificity: Gene expression is often tissue-specific. Measurements conducted on heterogeneous tissues can dilute signals from specific cell types, leading to misleading conclusions about overall gene expression.
Addressing biological variability requires a thorough experimental design, incorporating adequate controls and potential biological replicates to capture the true nature of gene expression within specific contexts.
Data Interpretation Issues
Once RNA sequencing data is acquired, interpretation poses significant challenges. The complexities of the data can lead to misinterpretations and inaccuracies:
- Statistical Challenges: The sheer volume of data generated makes statistical analysis complex. Proper choice of statistical models is crucial to draw valid conclusions but can be difficult for researchers who are not proficient in bioinformatics.
- Software Limitations: Many bioinformatics tools are available for data analysis, yet some may not effectively handle the unique characteristics of RNA-seq data. Bugs or limitations in software can distort results.
- Biological Context: Interpreting results without considering the biological context can lead to erroneous conclusions. It is vital to link findings to existing biological knowledge to ensure results are meaningful.
In summary, while RNA sequencing offers great insights, it is imperative to navigate the challenges presented during studies to ensure the reliability and validity of findings. Proper planning, execution, and data interpretation are critical to advancing our understanding of gene expression and its implications.
Future Directions in RNA Sequencing
The trajectory of RNA sequencing, commonly called RNA-seq, continues to expand, promising exciting developments for molecular biology. Understanding the future directions in RNA sequencing is crucial for several reasons. Firstly, it highlights how rapidly advancing technology can enhance research capabilities. Secondly, it reveals how integration with other biological data can lead to a more comprehensive understanding of life processes. Lastly, it underscores RNA-seq's potential impact on personalized medicine, tailoring treatments to individual patients based on their unique genetic makeup.
Technological Advancements
Technological advancements in RNA sequencing are poised to address current limitations and usher in new possibilities. High-throughput sequencing methods have progressed significantly, allowing researchers to generate vast amounts of data quickly and accurately. These innovations not only enhance speed but also increase the fidelity of the sequence data collected. For instance, long-read sequencing technologies, such as those from PacBio and Oxford Nanopore, enable a more extensive characterization of complex transcripts, including full-length isoforms and polyadenylation sites.
Additionally, improvements in bioinformatics tools are essential. These tools help to manage and analyze the large datasets generated by high-throughput sequencing. New algorithms and machine learning techniques provide deeper insights into gene expression dynamics and regulatory networks. As technologies continue to evolve, researchers can expect more streamlined processes and richer data interpretation.
Integration with Other Omics Data
The future of RNA sequencing also lies in its integration with other omics disciplines, such as genomics, proteomics, and metabolomics. The holistic view created by linking various omics data presents a clearer picture of biological systems. For example, combining RNA-seq data with proteomics can reveal how gene expression translates into protein levels and interactions. This multidimensional approach may uncover cellular responses to environmental changes and disease states.
Moreover, recent developments in multi-omics studies allow researchers to correlate transcriptomic data with genomic alterations, thereby identifying potential therapeutic targets. Such integrative analytics can significantly enhance our understanding of complex diseases like cancer.
Impact on Personalized Medicine
Personalized medicine is another exciting area where RNA sequencing is likely to make a substantial impact. By analyzing an individual's RNA profile, clinicians can identify unique genetic expressions that may influence disease susceptibility and drug response. This precision can lead to customized treatment plans, improving patient outcomes significantly.
Furthermore, insights gained from RNA-seq studies can pave the way for novel biomarker development. These biomarkers aid in early diagnosis and tracking disease progression, enabling timely interventions. As the integration of RNA sequencing into clinical practice grows, the potential for improved healthcare through targeted therapies will become a reality, profoundly changing patient management practices.
The future of RNA sequencing promises to intersect rapidly with technological advancements and personalized healthcare, offering unprecedented insights into molecular biology.
Closure
The conclusion serves as the capstone of our exploration into RNA sequencing. It synthesizes the insights gathered from previous sections, dismissing ambiguity in the context of RNA-seq's effect on molecular biology today. By clearly outlining the key findings and implications of RNA sequencing, we provide a comprehensive overview that not only reaffirms the relevance of the subject but also underscores its practical applications.
Summary of Key Points
A few key points stand out in this discussion:
- Significance in Gene Expression Research: RNA sequencing plays a crucial role in revealing gene expression patterns, allowing for the analysis of both normal and diseased states in organisms.
- Potential in Cancer Studies: Its application in cancer research has facilitated the identification of specific genetic variations linked to tumorigenesis, paving the way for targeted therapies.
- Probing Developmental Processes: By examining gene expression at various developmental stages, researchers can better understand complex biological processes that underpin growth and cellular differentiation.
- Environmental Impact Assessments: RNA-seq enables scientists to investigate how organisms respond to environmental changes, illustrating biological adaptability and resilience.
Overall, these points highlight the multifaceted applications of RNA sequencing and establish its foundational role in advancing our understanding of biology.
Implications for Future Research
As we look ahead, the future of RNA sequencing is filled with significant potential. Here are some implications worth noting:
- Technological Integration: RNA sequencing will likely integrate more seamlessly with other high-throughput methods, such as proteomics and metabolomics, providing a holistic view of cellular function.
- Advancements in Personalizd Medicine: The ability to tailor medical treatments based on gene expression data will continue to grow in importance, ultimately leading to improved patient outcomes in complex diseases.
- Standardization of Protocols: Ongoing efforts to standardize RNA sequencing methodologies can enhance comparability across studies, aiding reproducibility in findings and fostering collaboration among researchers.
"The impact of RNA sequencing extends beyond individual studies; it influences entire fields of research towards more precise and individualized approaches in medicine."
In summary, the conclusion reiterates not only the profound implications of RNA sequencing but also its vitality in guiding future research directions. Continued investment in this field will unlock new capabilities and insights into the biological world.