EHR Decision Support: Transforming Clinical Outcomes
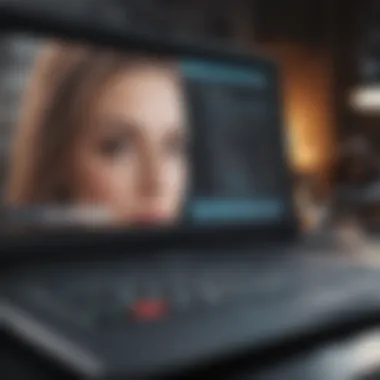
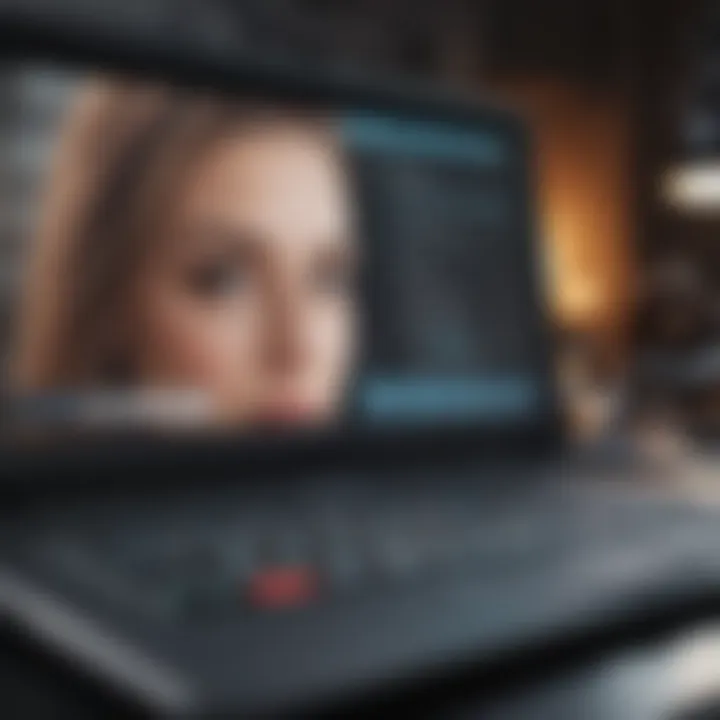
Intro
In the ever-evolving landscape of healthcare, technology continues to play a pivotal role in enhancing the delivery of care. Electronic Health Records (EHR) decision support systems are at the forefront of this transformation. They serve not just as digital filing cabinets but as essential tools that empower healthcare professionals to make informed decisions, ultimately leading to better patient outcomes. By integrating clinical knowledge and data, these systems provide real-time support, streamlining processes that can significantly impact the quality of patient care.
The integration of decision support within EHRs is not merely a trend but a necessity. As healthcare becomes more complex, with an ever-increasing pool of data and treatment options, the ability to navigate this information efficiently is more crucial than ever. The implications of leveraging EHR decision support are vast, stretching across various levels of healthcare, influencing both practitioners and patients alike.
To appreciate the scope of this discussion, consider how EHR decision support systems can lead to reduced medical errors, enhanced adherence to clinical guidelines, and improved population health management. Each of these points warrants a deeper exploration, revealing the multifaceted benefits they provide.
As we proceed, we will dissect the components that make these systems effective, address the challenges encountered in their implementation, and contemplate their future trajectory in the medical field. This dive into EHR decision support will highlight not only current applications but also the anticipated evolution of these vital resources in improving clinical outcomes.
Foreword to EHR Decision Support
In the realm of modern healthcare, the shift toward Electronic Health Record (EHR) systems presents an impressive evolution, particularly in the decision support mechanism that accompanies these records. The significance of EHR decision support can't be overstated; it stands as a pivotal component that influences how healthcare professionals engage with patient data and make critical decisions. In an age where data influences every facet of healthcare, the ability to interpret and act on that data swiftly is paramount. This article dives deeply into this integrated approach, considering not only the mechanics but also the broader implications of these systems on clinical outcomes.
Historical Context
The journey of EHR decision support systems can be traced back to the early digital records era, when the need for standardized, accessible information became apparent. In the 1960s, healthcare began its tentative steps into the digital realm, primarily driven by efforts to store patient data on computers. Early iterations were rudimentary at best and often isolated from practical clinical use.
However, as technology evolved, so did the capabilities of these systems. The 1990s saw significant advancements with the introduction of clinical decision support systems (CDSS). These systems were designed to provide clinicians with tailored patient data at the point of care, greatly enhancing diagnostic accuracy and treatment planning. Over the last two decades, the integration of sophisticated algorithms, machine learning, and natural language processing further revolutionized EHR decision support, shifting from simple alerts to comprehensive, context-aware suggestions for healthcare providers.
Definition and Purpose
EHR decision support systems are designed to aid healthcare providers by offering timely and relevant information that can influence clinical decision-making. At their core, these systems serve several essential purposes:
- Data Integration: They aggregate data from multiple sources, allowing for a holistic view of the patient's health history.
- Clinical Guideline Support: They incorporate evidence-based guidelines to assist in making treatment decisions that align with the latest research and best practices.
- Real-time Alerts: These systems notify clinicians of potential issues, such as drug interactions or abnormal lab results, thus enabling proactive management of patient care.
In essence, these systems are not just adjuncts to healthcare but rather essential tools that enhance the quality and safety of care provided to patients. They streamline workflows and help reduce the cognitive load on providers, allowing them to focus more on patient interaction and less on administrative tasks. The overarching aim is to improve patient outcomes while simultaneously enhancing the efficacy of healthcare delivery.
"The integration of EHR decision support is not merely a technological upgrade; it is a transformative shift in how healthcare is delivered, aimed at fostering a more informed and proactive approach to patient care."
Through the exploration of EHR decision support and its associated frameworks, the article seeks to shed light on its multifaceted role in contemporary healthcare environments. Examining each element comprehensively will elucidate the potential these systems hold for improving clinical outcomes and reshaping the landscape of medical practice.
Components of EHR Decision Support Systems
The heart of EHR decision support systems lies in their critical components, which work in concert to enhance clinical outcomes. Understanding these components is vital, as they significantly influence the efficiency of healthcare delivery and the quality of patient care. Let’s delve into the key elements that form the backbone of these systems.
Data Management
Data management is an essential aspect of EHR decision support systems, serving as the foundation upon which all functionalities sit. Without effective management of health information, the very purpose of decision support can quickly fall by the wayside. This encompasses not just the collection of patient data but also its organization, storage, and maintenance.
One significant benefit of robust data management is that it enables healthcare providers to have access to comprehensive and up-to-date patient records. A well-structured database helps in identifying trends and making informed clinical decisions. It is worth mentioning that the integrity and accuracy of the data are paramount. If the data is flawed, decision-making processes may lead to dire consequences.
Moreover, advanced data management techniques, like data mining and predictive analytics, offer healthcare teams valuable insights. These rolling insights empower clinicians by illuminating patterns in patient histories, predicting potential health risks, and suggesting appropriate interventions accordingly. In a fast-paced clinical environment, having relevant and reliable data at their fingertips can mean the difference between a successful diagnosis and a missed opportunity.
Clinical Guidelines Integration
The integration of clinical guidelines into EHR decision support systems establishes a framework that aligns healthcare practices with evidence-based guidelines. This component emphasizes the importance of systematic adherence to established clinical protocols.
When healthcare providers have seamless access to current clinical guidelines directly within their EHR systems, patient outcomes markedly improve. It reduces the likelihood of errors arising from outdated practices or misinformation. Furthermore, this integration fosters a culture of continuous learning among medical professionals, enabling them to stay updated on the latest evidence-based practices.
"Incorporating clinical guidelines within EHR systems not only streamlines workflows but also ensures that every decision made is rooted in sound evidence."
Additionally, integrating such guidelines into the workflow allows for real-time support during patient encounters. For instance, if a clinician is treating a patient with hypertension, the decision support system can automatically pull in the latest treatment protocols, thus providing reliable guidance right when it’s needed. This type of integration essentially turns the EHR into a dynamic tool that evolves with current medical standards.
Alerts and Reminders
Alerts and reminders represent the uncanny ability of EHR decision support systems to proactively assist clinicians in providing patient care. These components help in surfacing critical information at the right time, enhancing patient safety while ensuring thoroughness in clinical procedures.
Consider a scenario where a patient is due for a vaccination or a routine checkup; an automatic reminder can pop up, nudging the healthcare provider to take action. This is especially crucial in managing chronic diseases, where regular monitoring is vital for treatment success.
The significance of alerts cannot be overstated. They minimize the risk of oversights that could lead to delays in care or potential harm to patients. But while alerts could be a lifesaver, their effectiveness hinges on their design. If alerts are too frequent or irrelevant, clinicians may start ignoring them, which can do more harm than good.
A balance is necessary, thus making it essential for healthcare organizations to monitor and adjust the alerting systems to avoid “alert fatigue.”
Types of Decision Support Tools
In the realm of Electronic Health Record (EHR) decision support systems, various tools serve as the backbone for aiding healthcare providers. These tools are crucial for ensuring that clinicians can make informed choices, leading to improved patient outcomes. Harnessing the power of technology, the types of decision support tools span predictive analytics, diagnostic support systems, and treatment recommendations, each playing a unique role in modern healthcare. Their significance cannot be understated, as they not only enhance clinical workflows but also address the complexities of patient care with precision and efficiency.
Predictive Analytics
Predictive analytics represents a cornerstone of decision support tools, utilizing historical data and statistical algorithms to forecast future events. It goes beyond mere number-crunching by turning raw data into actionable intelligence, thus assisting healthcare professionals in early intervention efforts.
Imagine a scenario where a hospital utilizes predictive analytics to identify patients at high risk for readmission based on previous admissions, demographic factors, and chronic conditions. By flagging these individuals, care teams can proactively create tailored discharge plans aimed to reduce readmissions, ultimately leading to healthier outcomes and cost savings. Moreover, predictive analytics can be a game changer in areas such as population health management. By recognizing patterns and trends in health data, healthcare providers can mobilize resources effectively to ensure that vulnerable groups receive the care they need.
"Predictive tools can support clinicians in identifying best practices, ultimately steering the ship toward better care delivery."
Diagnostic Support Systems
In the landscape of clinical decision-making, diagnostic support systems provide an invaluable resource for healthcare providers. They leverage data from clinical guidelines and patient records to assist in the accurate diagnosis of conditions. These systems effectively act as an extra set of eyes, often catching potential misdiagnoses that a clinician may overlook.
For example, a diagnostic support tool might analyze symptoms entered into the EHR against a database of medical cases, suggesting possible diagnoses that the provider might consider. This is especially vital in complex cases where symptoms can overlap across multiple conditions. Additionally, these systems can integrate seamlessly with existing EHRs, delivering suggestions without interrupting the clinician's workflow, thus enhancing user experience.
Treatment Recommendations
When it comes to treatment options, decision support tools take the guesswork out of patient management. Treatment recommendation systems synthesize clinical guidelines, drug interactions, and patient data to suggest the best course of action tailored to individual patients. These recommendations ensure that patient care is evidence-based and consistent with current medical standards.
For instance, upon entering a new patient into the system, a provider can receive prompts regarding appropriate treatment protocols considering the patient’s specific health conditions and history. This utility not only helps improve treatment accuracy but also minimizes the risk of adverse effects due to drug interactions. As a result, patients experience better care outcomes, as the treatment process becomes more streamlined and focused on their unique needs.
Benefits of EHR Decision Support Systems
EHR decision support systems stand at the intersection of technology and healthcare, playing a pivotal role in enhancing clinical outcomes. Their importance cannot be overstated, as they integrate vast amounts of patient data and clinical guidelines to assist healthcare providers in making informed decisions. When implemented effectively, these systems can lead to substantial improvements across multiple dimensions of patient care.
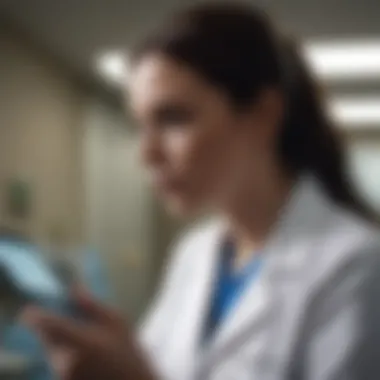
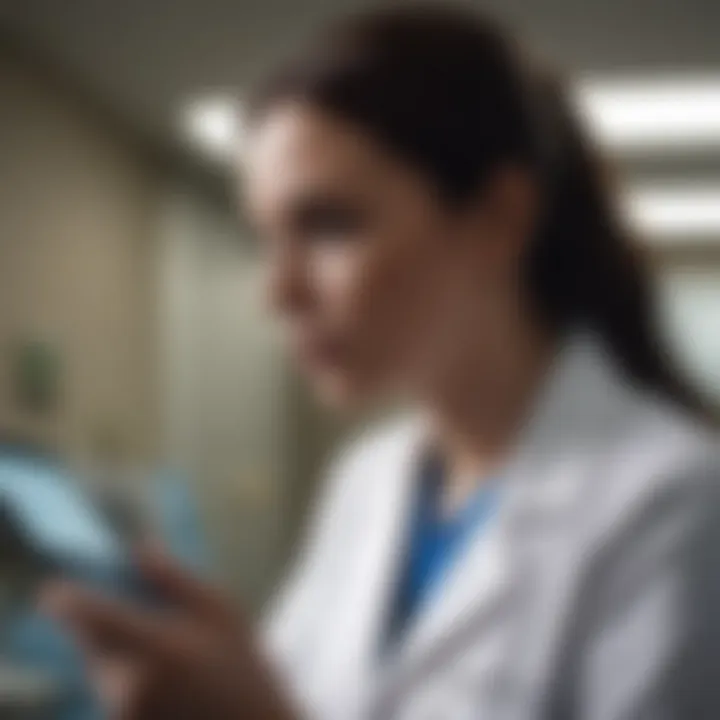
Improved Patient Outcomes
Improved patient outcomes are perhaps the most visible benefit of EHR decision support systems. By providing real-time access to crucial patient information, including lab results, previous conditions, and medications, healthcare providers can tailor their approaches to meet each patient’s unique needs. For instance, a primary care physician using an EHR system can quickly identify potential drug interactions, ensuring that a prescribed medication won’t adversely affect a patient’s existing treatment regimen. This vigilant attention to detail not only increases safety but often enhances the overall effectiveness of treatment plans.
"Better data leads to better decisions, resulting in happier patients and healthier communities."
Moreover, EHR decision support systems facilitate preventive care by alerting providers to necessary screenings and vaccinations based on age, lifestyle, or medical history. This proactive approach can significantly reduce the risk of chronic diseases and their associated complications, which are often costly for both patients and the healthcare system as a whole.
Enhanced Accuracy in Diagnoses
The accuracy of diagnoses is another critical area where EHR decision support shines. One of the key components of these systems is their ability to analyze complex datasets quickly. For example, a tool that offers diagnostic support can sift through symptom data, lab results, and clinical guidelines simultaneously. Take, for example, a patient presenting symptoms suggestive of various respiratory illnesses; an EHR system can help narrow down the possibilities based on historical data and recent evidence.
The integration of clinical best practices within EHR systems aids healthcare providers in following established protocols and guidelines for diagnosis. This structured approach minimizes the risk of human errors that can arise from misinterpretations or oversight. By empowering clinicians with data-driven insights, the systems support more accurate diagnoses and, consequently, reduce instances of misdiagnosis or delayed treatment.
Efficiency in Clinical Workflows
Efficiency is the name of the game in healthcare. EHR decision support systems streamline clinical workflows by automating many routine processes. For example, the streamlined order entry for tests and prescriptions reduces the time spent on paperwork, allowing healthcare professionals to focus more on patient care than administrative tasks. This not only enhances productivity but also leads to better job satisfaction among providers.
In addition, these systems often utilize alerts and reminders effectively. When a patient requires follow-up appointments or additional tests, alerts pop up in the provider's workflow, ensuring critical tasks do not slip through the cracks. This integrated organization fosters a more efficient healthcare environment, in which both patients and providers thrive.
- Elements contributing to efficiency include:
- Automated documentation processes
- Real-time data accessibility
- Reduced redundancies in tests and evaluations
As healthcare continues to evolve, the importance of EHR decision support systems becomes increasingly apparent. They are not merely helpful tools but essential components that enhance patient outcomes, improve diagnostic accuracy, and streamline clinical practices.
Challenges and Limitations
The realm of Electronic Health Records (EHR) decision support systems offers numerous advantages, yet it is not without its hurdles. Understanding the challenges and limitations is crucial for navigating the complexities of this technology in healthcare. These issues not only impact the implementation processes but also influence overall clinical outcomes. Addressing these points is essential for ensuring that EHR decision support systems reach their full potential in improving patient care.
Integration Issues
One of the significant challenges facing EHR decision support systems is the issue of integration with existing healthcare technologies. Many organizations have legacy systems, which may not seamlessly work with new EHR platforms. Poor integration can lead to data silos where important patient information is inaccessible or underutilized. This scenario not only hinders the effectiveness of decision support tools but also impacts clinicians’ ability to deliver timely care.
- Technical Compatibility: Different systems often run on varying protocols and architectures, making it tough to achieve interoperability.
- Workflow Disruption: Integrating new tools into established workflows requires careful planning; otherwise, it can disrupt the natural flow of clinical processes.
Failing to adequately address these integration issues can lead to frustration among healthcare providers, potentially affecting patient outcomes.
User Acceptance and Training
User acceptance stands at the crossroads of technology implementation and actual usage. If the healthcare staff do not embrace the decision support systems, the investments made could fall flat. A robust training program becomes paramount. Training should not just be a one-time orientation session but a continuous process.
- Resistance to Change: Clinicians often have reservations about adopting new tools, worrying that they may complicate their already busy schedules.
- Training Depth: It is not enough to simply introduce the tool; users must be educated on how to use it effectively. Incorporating real-world scenarios into training sessions can enhance practical knowledge.
“Without effective training and user acceptance, technology can easily turn into yet another burden, rather than a boon.”
Failing to prioritize this aspect may lead to underutilization of the systems, counteracting the intended benefits of enhanced clinical decision-making.
Data Privacy Concerns
In an age where data breaches loom large, concerns over data privacy in EHR decision support systems cannot be underestimated. Protecting patient information is both a legal requirement and an ethical obligation. The sensitivity of health data heightens the importance of ensuring stringent privacy measures.
- Legal Compliance: Organizations need to stay compliant with regulations like HIPAA in the U.S., which governs how protected health information is handled.
- Data Security: Implementing robust cybersecurity measures is essential to prevent unauthorized access and data breaches, which can have devastating effects on both patients and healthcare providers.
Neglecting these data privacy concerns could lead to severe repercussions, including legal ramifications and a loss of trust among patients.
In essence, addressing challenges related to integration, user acceptance, and data privacy is fundamental for maximizing the impact of EHR decision support systems in enhancing clinical outcomes. Only by actively tackling these limitations can healthcare providers truly harness the power of technology in their practice.
The Role of Artificial Intelligence
Artificial Intelligence (AI) is becoming an integral component in transforming Electronic Health Records (EHR) decision support systems. Its capabilities go beyond mere data processing; AI plays a pivotal role in enhancing clinical outcomes through various innovative techniques. As healthcare landscapes evolve, the introduction of AI tools is reshaping the ways in which clinicians access, interpret, and utilize patient data.
The significance of AI in decision support systems lies in its ability to analyze vast amounts of health information in real-time. This leads to improved diagnostic accuracy, timely treatment recommendations, and personalized patient care. With the ongoing challenges in the healthcare sector, integrating AI can lead to more effective patient management and optimized resource allocation.
Moreover, the thoughtful implementation of AI carries implications for reducing costs while boosting the quality of care, an essential goal for modern healthcare settings.
Machine Learning in Decision Support
At the heart of AI is machine learning, a technology that empowers systems to learn from data and make intelligent decisions without explicit programming. In the context of EHR decision support, machine learning algorithms can process patient data and identify patterns that may not be obvious at first glance.
- Predictive Analytics: This allows healthcare providers to forecast outcomes based on historical data. For instance, algorithms can analyze patient records to identify individuals at risk of certain conditions, such as diabetes or heart disease, enabling proactive interventions.
- Tailored Treatment Plans: Machine learning can help create personalized treatment plans by analyzing data from similar patient cases and outcomes, ultimately leading to more effective healthcare solutions.
- Continuous Learning: These systems continuously improve as they ingest more data, fine-tuning their accuracy and relevance over time.
However, integrating machine learning isn't without its hurdles. Ensuring data quality and addressing privacy concerns are paramount.
Natural Language Processing Applications
Natural Language Processing (NLP), another branch of AI, focuses on the interaction between computers and human language. In EHR systems, NLP processes unstructured data—like physician notes and patient feedback—that often holds critical insights.
- Information Extraction: Through NLP, decision support tools can extract vital information from free-text entries, facilitating quicker access to patient histories and pertinent clinical notes.
- Clinical Sentiment Analysis: By assessing the sentiment in patient surveys or feedback, providers gain qualitative insights into patient satisfaction and can adjust care strategies accordingly.
- Automated Documentation: NLP can also streamline the documentation process, aiding in coding and billing by transforming verbal notes into coded entries efficiently.
Taking advantage of NLP assists healthcare professionals in approaching patient care with a clearer understanding of individual needs and sentiments.
This technology marks a significant leap towards creating more efficient, responsive, and empathetic healthcare environments, providing clinicians with better tools to support their practice.
Future Directions of EHR Decision Support
The landscape of Electronic Health Records (EHR) decision support is not static; it is continuously evolving. This section sheds light on the future directions of EHR decision support systems, emphasizing emerging technologies and significant regulatory considerations. As healthcare providers increasingly rely on these complex systems, understanding their trajectory is crucial for stakeholders at every level.
Emerging Technologies
EHR decision support is on the cusp of a technological revolution, driven by innovations that promise to enhance the user experience and improve patient care.
- Artificial Intelligence (AI): AI is pivotal in transforming EHR decision support by enabling predictive analytics and tailored treatment recommendations. For instance, algorithms can analyze patient data, identify at-risk individuals, and suggest preventive measures. Such predictive capability can significantly reduce complications in chronic illnesses.
- Blockchain Technology: While commonly associated with cryptocurrencies, blockchain holds immense potential in safeguarding patient data within EHR systems. By ensuring data integrity and secure sharing between providers, blockchain can enhance trust and transparency, ultimately benefiting patient outcomes.
- Wearable Health Devices: These devices can seamlessly flow data into EHR systems in real time. This integration enables healthcare providers to monitor their patients continuously, allowing for timely interventions. For example, a patient with heart issues wearing a monitor can alert their doctor if irregular patterns are detected.
- Cloud Computing: Moving decision support systems to the cloud can facilitate better access to data, promote collaboration among healthcare teams, and reduce infrastructure costs. In scenarios where immediate information is essential, cloud-based systems can be a game-changer.
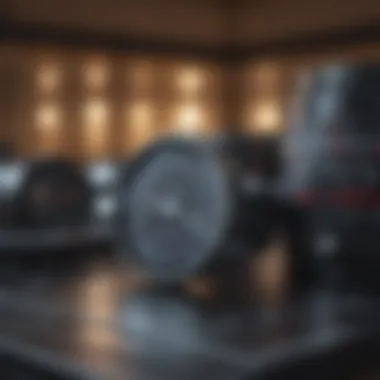
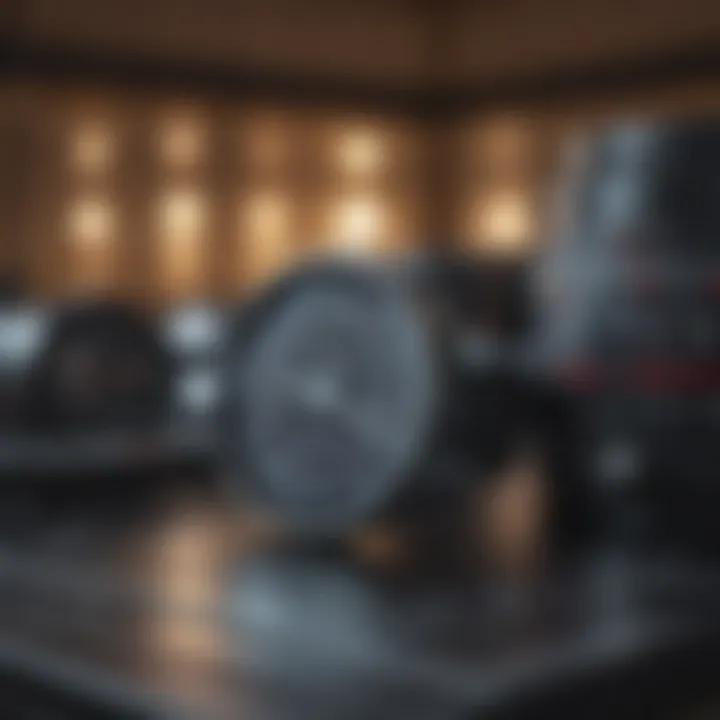
"The future of decision support lies in our ability to integrate diverse technologies to create a seamless and comprehensive healthcare experience."
— Anonymous
Policy and Regulatory Considerations
As EHR decision support systems advance, regulatory frameworks must adapt to keep pace with the technology. This domain is fraught with challenges and opportunities that demand careful attention.
- Data Privacy and Security Regulations: With advances in technology, the necessity to protect patient data becomes even more pressing. Policies like HIPAA in the U.S. must evolve to encompass new technologies like AI and blockchain, ensuring robust privacy controls while facilitating innovation.
- Standardization Efforts: Lack of standardization can lead to discrepancies in data interpretation and decision-making processes. Regulatory bodies need to implement clearer guidelines on data formats, interoperability, and system functionalities. Such standards can pave the way for smoother integrations across different platforms.
- Compliance and Accountability: There needs to be a clear delineation of responsibility when AI systems are involved in clinical decision-making. Who is accountable if an algorithm leads to adverse patient outcomes? Policymakers must establish frameworks that clearly define accountability to fully embrace the potential of AI in healthcare.
- Training and Certification Requirements: As new technologies are introduced, healthcare providers must receive proper training. Regulatory bodies may need to consider formal certification processes to ensure proficiency in using advanced decision support tools.
Case Studies on EHR Decision Support Implementation
Exploring case studies related to the implementation of Electronic Health Records (EHR) decision support systems reveals considerable insights into their efficacy, operationalization, and the real-world challenges organizations face. These examples serve as valuable lessons for healthcare institutions seeking to enhance clinical outcomes through technology. Understanding both the success stories and the hurdles can guide future strategies, making this analysis meaningful for professionals looking to adopt or enhance similar systems.
Successful Implementations
Across various healthcare settings, there have been bright spots demonstrating the strategic benefits of EHR decision support systems. One notable example is the Mayo Clinic, which integrated a sophisticated decision support tool within its EHR. The system aids clinicians in diagnosing and managing complex cases by providing comprehensive clinical guidelines directly within the workflow. This integration has reportedly led to a marked decrease in diagnostic errors and an increase in treatment accuracy.
Another success story can be seen in hospitals like the Cleveland Clinic, which adopted a specialized decision support system focusing on cardiovascular care. By leveraging predictive analytics for risk assessment, the system enabled cardiologists to evaluate patient risk factors in real-time, engaging them to make data-driven choices. Following the implementation, the facility noted improvement in both patient satisfaction and clinical outcomes.
These implementations underscore key advantages:
- Increased Efficiency: Reducing the time clinicians spend searching for guidelines or data improves patient flow and satisfaction.
- Enhanced Accuracy: Access to updated guidelines helps providers make informed decisions, lessening the risk of complications.
- Patient-Centric Approach: Tools that provide real-time insights foster a more tailored patient care experience, promoting better health outcomes.
Lessons Learned from Failures
Contrastingly, not every case has showcased harmonious integration. A compelling instance is found in a mid-sized hospital that entered a partnership to adopt a comprehensive EHR decision support system tailored to oncology. Early excitement turned to frustration when the predicted benefits failed to materialize. Key issues surfaced, such as:
- Lack of User Training: Staff reported feeling overwhelmed by the complexity of the tool. Proper training sessions were limited, resulting in underutilization of the system's features.
- Integration Challenges: The system struggled to incorporate existing patient data accurately, leading to delays and miscommunications in patient records.
- Resistance to Change: Some healthcare professionals preferred traditional methods, feeling the new system complicated their workflow rather than enhancing it.
Lessons drawn from these missteps are essential for guiding future implementations. Key takeaways include:
- Invest in Training: Ongoing education and support for users can bridge the knowledge gap and foster acceptance.
- Prioritize Integration: Ensuring smooth integration with existing workflows and data can significantly enhance user experience and adoption rates.
- Engage Stakeholders: Involving healthcare professionals in the selection and development process can lead to a greater acceptance and tailored functionality.
Interdisciplinary Collaboration
In the rapidly evolving landscape of healthcare, interdisciplinary collaboration stands as a cornerstone. This artful mingling of various expertise helps in addressing complex clinical challenges that a single discipline might struggle to tackle. Just as a chef relies on an array of spices to create a unique flavor, healthcare teams harness diverse knowledge and skills to improve patient care. Each professional brings distinct perspectives to the table, which enhances innovation and clinical outcomes.
Engaging Stakeholders in Development
Effective engagement of stakeholders is essential in the development of EHR decision support systems. When creating these systems, it's crucial to go beyond just the developers and technical staff. One should involve clinicians, nurses, administrators, patients, and even IT specialists in the design process. This inclusion helps uncover the real-world challenges faced by end-users, ensuring the tools developed align with their needs.
While one might think that integrating feedback is straightforward, it can be a tricky dance. Different stakeholders may have conflicting priorities or viewpoints. Navigating this minefield requires patience, communication, and a clear vision. Throughout the development, transparency is paramount. Keeping stakeholders informed about design choices and timelines fosters trust and buy-in, which ultimately contributes to more effective systems.
Furthermore, user acceptance hinges on their input during the development. If users believe their voices have been heard, they are far more likely to embrace the new technology instead of resisting it. It's about making these systems intuitive; when users feel they are part of the creation process, it paves the way for smoother transitions.
Healthcare Team Integration
Integrating EHR decision support effectively within healthcare teams is undeniably a balancing act. A well-coordinated team can leverage these systems to provide optimal patient care, yet barriers often exist. These barriers might be technological, cultural, or even procedural. Namely, if one professional doesn’t understand how to use a new tool, the benefits can quickly evaporate.
Training becomes an essential player in this narrative. A robust educational program ensures that all team members, from doctors to nursing aides, understand how to utilize the EHR effectively. This ongoing education is not a one-and-done deal; refresher sessions or updates to account for new features should be standard practice. Additionally, regular discussions about the technology in team meetings help to reinforce its relevance and application.
A collaborative environment thrives when team members feel comfortable sharing insights or challenges they encounter with the EHR. Integrating feedback and adapting processes accordingly make these tools more impactful over time. After all, if the technology isn’t enhancing teamwork and patient outcomes, it’s merely adding another layer of complexity.
"Collaboration is not just a buzzword; it is an integral part of achieving superior healthcare outcomes."
Ethical Considerations in Decision Support
As we navigate the landscape of Electronic Health Records (EHR) decision support systems, ethical considerations emerge as a cornerstone of effective implementation and use. These considerations not only influence the design and functionality of these systems but also play a critical role in forming trust between patients and healthcare professionals. The conversation around ethics in this context is multi-faceted and increasingly relevant in today's technology-driven healthcare environments.
Bias in Algorithms
Machine learning and algorithms significantly shape the modern decision support tools found within EHR systems. However, biases embedded in algorithms can lead to major ethical dilemmas. It's commonly understood in technology circles that an algorithm is only as good as the data it learns from. If the training data reflects historical inequalities or skewed demographics, the resulting model could inadvertently perpetuate these biases. For instance, if patient data predominantly represents one demographic group, recommendations might not adequately address the needs of different populations.
The implications of biased algorithms are profound; they can result in inequalities in treatment options and exacerbate health disparities. If one considers algorithms used in predictive analytics, which assess the risk of patients developing conditions based on historical health data, any bias in calculation can lead to mismanagement of care for marginalized groups.
Given this backdrop, stakeholders must be proactive. Regular audits to identify and mitigate bias in algorithmic outputs are essential steps in ensuring that fairness is woven into the fabric of EHR decision support systems. Additionally, engaging diverse teams in the development of these algorithms can help mitigate biases. After all, a kaleidoscope of perspectives can lead to more equitable healthcare recommendations.
Informed Consent Protocols
Informed consent remains a fundamental ethical principle in healthcare, particularly when used in conjunction with EHR decision support systems. Such systems often utilize patient data to inform clinical decision-making and must ensure that patients understand how their data is used and the potential implications.
Creating clear and comprehensive informed consent protocols is paramount. Patients ought to be educated about how their information will be utilized by the EHR system. This includes exploring features such as how data might assist healthcare providers in making treatment decisions or predicting health outcomes. However, this must be communicated in a tangible manner, avoiding medical jargon that might leave patients scratching their heads.
Moreover, ongoing consent is crucial. Patients should be offered the opportunity to withdraw their consent at any stage should they become uncomfortable with how their data is being utilized. This respect for patient autonomy is not just legal duty—it's an ethical imperative that fosters greater trust in the healthcare system.
"Ethics isn’t just a set of rules; it’s a framework for understanding how technology intersects with human rights and accessibility."
In the long run, the backbone of ethical considerations in EHR decision support systems will rely heavily on transparency, ongoing education, and engagement. By addressing biases that seep into algorithms and ensuring robust informed consent processes, stakeholders can harness the potential of technology while upholding ethical standards crucial for patient trust and better clinical outcomes.
Comparative Analysis of EHR Decision Support Systems
In today’s healthcare environment, the effectiveness of Electronic Health Record (EHR) decision support systems cannot be overstated. This comparative analysis seeks to uncover the nuances among various EHR decision support platforms and illuminate the benefits, considerations, and specific elements that make each unique. The importance of this analysis lies in fostering informed choices for healthcare providers who can utilize these systems to enhance patient care, streamline workflows, and achieve better clinical outcomes.
Evaluating Different Platforms
When it comes to evaluating different EHR decision support systems, healthcare professionals must keep in mind several key components. It’s not merely about features; it’s also about functionality in real-world settings. The original aim of these systems is to translate complex medical data into actionable insights. Here’s what to think about:
- Interoperability: Can the system seamlessly integrate with existing hospital applications? This ensures smooth data exchange and reduces information silos.
- User Interface Design: A user-friendly interface can significantly enhance user adoption. A good layout, with easy-to-navigate menus, allows clinicians to focus more on patient care instead of wrestling with the technology.
- Customization: The ability to tailor decision support tools according to specific patient populations or specialties can dramatically impact their effectiveness.
Different platforms can range from those providing basic alert systems to more advanced ones employing machine learning algorithms to adapt to individual patient needs.
"When evaluating platforms, think not just about what they offer, but how they fit your specific clinical environment."
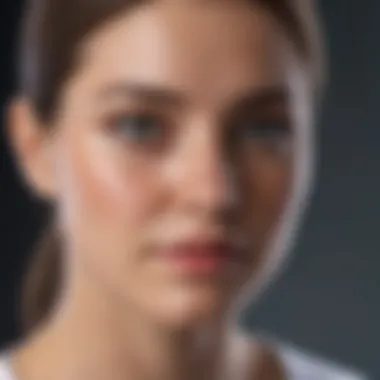
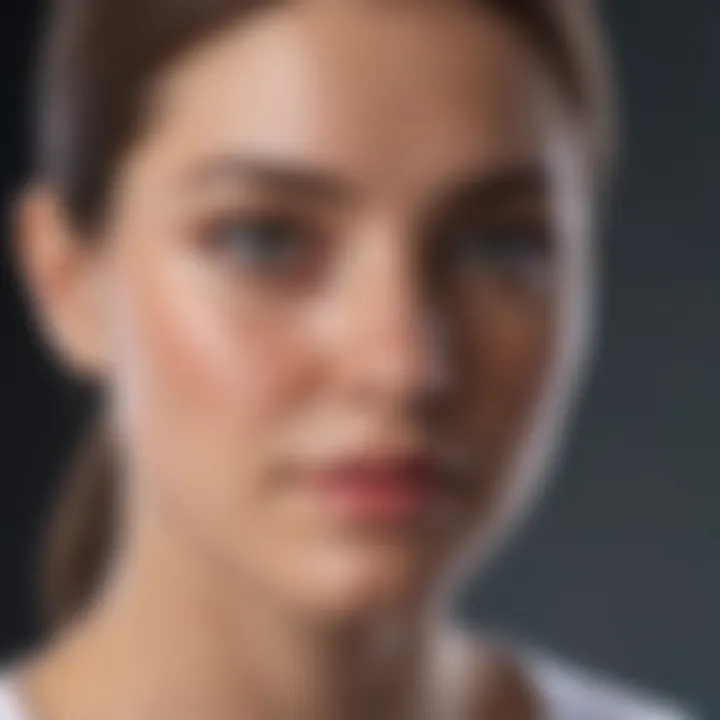
User Experience and Satisfaction
User experience can make or break the success of an EHR decision support system. In settings where time is of the essence, clinicians often find themselves grappling with systems that slow their pace rather than enhance it. Thus, understanding user satisfaction becomes pivotal not only for retention of the technology but also for improving patient outcomes.
Consider the following factors that drive user satisfaction:
- Speed and Responsiveness: A system that responds quickly to user queries can enhance workflow and decrease clinician frustration.
- Training and Support: Comprehensive training helps users feel equipped to leverage the system fully, leading to improved patient management. Ongoing support is beneficial, too.
- Feedback Mechanisms: Systems that incorporate user feedback for continuous improvement show responsiveness to clinician needs, fostering a collaborative partnership.
Ultimately, the effectiveness of any EHR decision support system hinges on its ability to be embraced by its users. Assessing user satisfaction provides crucial insights into performance as well as areas for enhancement, ensuring that technology remains a vital ally in patient care.
Training and Education for Healthcare Providers
The healthcare landscape is ever-evolving, with a myriad of new technologies reshaping how care is delivered. At the heart of this revolution are Electronic Health Record (EHR) decision support systems—essential tools that help providers make informed clinical decisions. However, even the most advanced technology is only as good as the people using it. This is where training and education come into play. It’s crucial for healthcare providers to be well-versed in these systems to maximize efficacy and improve patient outcomes.
Education ensures that healthcare professionals can navigate EHR decision support tools efficiently. Without proper training, these tools risk being underutilized, which could negate their potential benefits. When healthcare providers understand how to use EHR decision support, it translates into more accurate diagnoses, timely interventions, and ultimately, better patient care.
Developing Training Programs
Creating an effective training program for healthcare providers involves multiple layers tailored to diverse learning styles. First, institutions should assess the needs of their staff—understanding what providers already know and where gaps exist is vital. Here are several key elements to consider:
- Curriculum Design: The content should be relevant and updated frequently, addressing not just basic functionalities but also advanced features of the EHR system.
- Hands-On Training: Simulation-based training sessions can provide real-life scenarios where healthcare providers practice decision-making using the EHR.
- Resource Accessibility: It’s important to ensure that training materials—videos, manuals, and quick-reference guides—are easily accessible to all staff.
- Feedback Mechanisms: Regularly soliciting feedback from participants can refine the training process and make it more effective over time.
Implementing these components in a training program may not only increase proficiency but also build a confident workforce. A well-trained team fosters an environment where EHR decision support is viewed as an ally in the quest for improved clinical outcomes.
Assessing Training Effectiveness
Once training programs are in place, it’s just as critical to evaluate their effectiveness. This involves more than just a quick review; it requires an ongoing commitment to quality assurance. To assess the impact of training on EHR decision support usage:
- Pre-and Post-Training Assessments: Testing knowledge levels before and after training can provide clear metrics on improvement.
- Observation: Monitoring healthcare providers in real clinical settings can help document their ability to apply new skills in practice.
- Outcome Metrics: Tracking patient outcomes, efficiency in data entry, and error rates pre- and post-training will offer insights on the training's impact on real-world performance.
- Follow-Up Surveys: Conducting surveys several months after training can shed light on how knowledge retention affects ongoing usage.
Training is not a one-time event but a continuous process that reflects the ever-changing nature of healthcare technology. Ensuring healthcare providers are adept in their use of EHR decision support is integral for delivering high-quality care. With ongoing assessment, institutions can adapt their strategies to meet healthcare challenges head-on, ensuring providers are equipped with the necessary tools and knowledge to succeed.
"Effective training bridges the gap between technology and patient care, transforming potential into performance."
Impact of EHR Decision Support on Financial Aspects
The financial implications of EHR decision support systems are profound, affecting various stakeholders in the healthcare landscape. The integration of these systems correlates directly with financial performance, influencing costs, revenue generation, and overall sustainability of healthcare practices. Understanding the financial aspects is essential, not just for healthcare providers, but also for policymakers, investors, and insurance companies involved in this ecosystem.
Effective EHR decision support can lead to significant cost savings, which is a chief concern for healthcare facilities under constant pressure to control spending. The automation of routine tasks minimizes the time healthcare professionals spend on administrative duties, allowing them to focus more on patient care. As a result, this can lead to reduced labor costs over time. Furthermore, fewer billing errors, enhanced coding accuracy, and improved compliance with regulations translates into financial benefits for practices that adopt these systems.
In addition to cost savings, EHR decision support can enhance revenue streams. By providing timely information, clinicians can make better treatment decisions, which can lead to improved patient outcomes and satisfaction. Patients who have positive experiences are more likely to return and recommend services, promoting a stronger patient base and potentially increasing profitability.
Cost-Benefit Analysis
When evaluating the financial aspects of EHR decision support systems, a cost-benefit analysis is a useful tool. It aims to weigh the costs incurred during the implementation of these systems against the financial benefits realized over time.
- Initial Investment: Implementing EHR technology requires a notable initial outlay. This includes costs related to software purchase, system installation, and necessary hardware. There are also indirect costs, including training staff to use the new system efficiently.
- Operational Costs: Ongoing expenses are also connected to system maintenance and updates, user training refreshers, and technical support. These recurring costs can add up and must be factored in.
- Measurable Benefits: Ideally, over time, the efficiency gained through decision support leads to savings in labor, reducing overtime, and limiting the frequency of costly errors. Healthcare organizations should monitor metrics like the rate of claims denied due to coding mistakes, which can be tracked to quantify return on investment.
"It's essential to see beyond the initial costs of EHR decision support, understanding that long-term gains can greatly overshadow upfront expenses."
Return on Investment Considerations
Determining the Return on Investment (ROI) for EHR decision support systems involves assessing the overall financial benefits compared to the costs incurred during implementation.
- Time-Savings: Increased operational efficiency allows healthcare workers to see more patients and spend less time managing records, directly impacting revenue generation. This time-savings can be substantial, translating into increased number of patient encounters.
- Quality Improvements: Enhanced decision-making capabilities can lead to better treatment protocols and fewer complications, which reduces hospitalization costs and lengths of stay, thus benefiting the bottom line. This quality of care can also minimize the financial risk associated with malpractice lawsuits.
- Reimbursement Rates: Many insurers increasingly tie reimbursement rates to the quality of care delivered. EHR decision support systems, by facilitating adherence to best practices and clinical guidelines, can contribute positively to these rates.
In summary, the financial implications of EHR decision support systems encompass a variety of factors, from initial costs to long-term savings and enhanced revenue opportunities. By carefully analyzing the cost-benefit dynamics and ROI, healthcare organizations can make informed decisions that bolster both clinical outcomes and their financial viability.
Global Perspective on EHR Decision Support
Understanding EHR decision support from a global perspective is central to appreciating the nuances of healthcare delivery in different countries and regions. Each healthcare system has its unique features, influenced by cultural, economic, and regulatory factors. By examining these variances, we can unveil the potential enhancements such systems may offer to clinical outcomes worldwide.
Differences Across Healthcare Systems
When we look at EHR decision support systems through a global lens, it’s crucial to recognize that no two healthcare systems are alike. Some nations have highly integrated, government-funded health systems, while others lean towards privatized care. For instance, countries such as Sweden and the UK may have EHR systems that are deeply embedded within a national healthcare infrastructure, focusing heavily on uniformity and accessibility. In contrast, the United States presents a fragmented approach where multiple vendors vie for market share, leading to differing degrees of interoperability and functionality.
In the UK, for example, the National Health Service (NHS) has introduced centralized decision support systems that emphasize evidence-based pathways. These systems help clincians follow best practice guidelines sourced from research. Conversely, in the U.S., hospitals often end up customizing EHR solutions, resulting in a plethora of systems that don’t communicate well with one another. Such differences underline the need for tailored training and support that aligns with the local context of each healthcare environment.
"Global variations in EHR systems underscore the importance of adapting decision support technologies to fit the unique needs and practices of local healthcare providers"
Cultural Impacts on Implementation
Cultural factors also play a pivotal role in the successful implementation of EHR decision support systems. In cultures that cherish collective decision-making, healthcare providers might find it easier to foster teamwork in using these technologies. In contrast, more hierarchical societies may see challenges as order and authority might stymie collaborative practices that decision support systems often rely on.
For instance, in Japan, where a blend of technological advancement and deep-rooted traditions prevails, there is significant trust in technology among health professionals. This can facilitate smoother adoption of EHRs and their associated decision support functionalities. Meanwhile, in countries where there is skepticism towards technology, healthcare professionals may resist using these systems, fearing that they could replace clinical judgment with algorithms.
Moreover, the assumed roles of directives and protocols may vary. Some regions prioritize clinical autonomy, while others might endorse strictly following decision pathways laid out by healthcare authorities. Understanding these cultural dynamics is integral for successful implementation as it directly impacts how providers engage with technology and the subsequent clinical outcomes.
In summary, a global perspective on EHR decision support highlights differences in systems and emphasizes the need to account for cultural variations in their implementation. As healthcare continues to evolve on a global scale, so too must our approaches to the technologies that support it.
Culmination
The final section wraps up the insights and value derived from examining EHR decision support systems in the context of modern healthcare. It is crucial to recognize that the integration of technology in clinical settings not just enhances operational efficiency, but significantly influences patient outcomes. Notably, these systems bridge the gap between data and actionable insights, equipping healthcare professionals with the necessary tools to make informed decisions.
Summarizing Key Insights
To summarize the key findings presented throughout the article:
- EHR decision support systems are essential components in contemporary healthcare, providing real-time assistance to clinicians.
- These systems have shown potential in improving accuracy during diagnoses and optimizing treatment plans, which can lead to better patient satisfaction and outcomes.
- Challenges such as integration issues with existing systems, resistance from users, and concerns regarding data privacy still pose barriers that must be addressed.
- Stakeholder engagement and continuous training are vital for successful implementation.
- Emerging technologies, particularly AI and machine learning, will likely shape the future landscape of these systems, providing even more sophisticated tools to aid clinical decision making.
Here, it is evident that EHR decision support systems represent a significant investment for the healthcare industry. When successfully integrated, they streamline workflows and offer insights that allow clinicians to provide personalized care tailored to individual patient needs.
Future Research Directions
Looking ahead, several avenues warrant deeper exploration:
- Impact Assessment: There is a need for comprehensive studies that assess the long-term impacts of EHR decision support on patient care outcomes at various institutions.
- User Experience Research: Understanding how clinicians and other healthcare personnel interact with these systems can guide enhancements in design and functionality.
- Ethical Standards Development: Research into the establishment of ethical standards, particularly regarding bias in algorithms, is crucial as systems become increasingly automated.
- International Comparisons: Investigating how different countries implement and utilize EHR systems can yield valuable insights, helping to identify best practices and adaptations across diverse healthcare environments.
Overall, as we navigate an increasingly complex healthcare landscape, research into EHR decision support systems will provide the backbone necessary to ensure the clinical environment evolves in tandem with technological advancements. This commitment to enhancing decision support systems not only promises to improve direct patient care but also optimizes the broader operational efficiency of healthcare facilities, ultimately leading to a more effective healthcare delivery model.