How AI is Shaping the Future of Healthcare
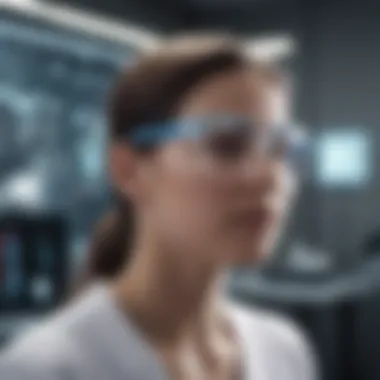
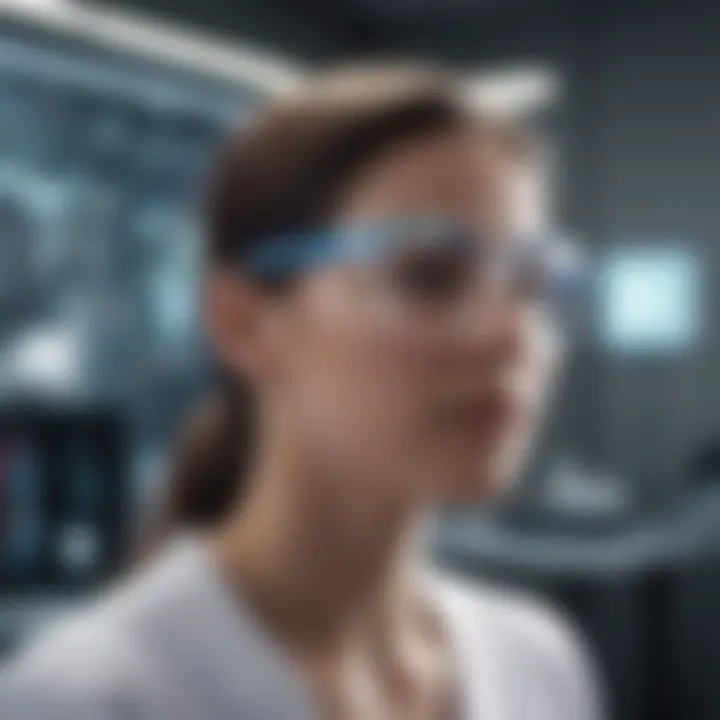
Intro
The world of healthcare is in a constant state of evolution, and recently, artificial intelligence has burst onto the scene as a force to be reckoned with. The synergy between AI and healthcare leaves no stone unturned, as it digs deep into diagnostics, treatment, administrative tasks, and even ethical debates surrounding technology in medicine. This intersection not only reshapes how practitioners deliver care but also redefines patient experiences and outcomes.
When we discuss the implications of AI in healthcare, we’re not merely talking about robots in operating rooms or algorithms crunching numbers to find diseases. We are entering an era where tasks once thought to be the exclusive domain of highly trained medical professionals are now becoming enhanced—making accuracy and personalization the new standard. This article aims to delve thoroughly into how AI is driving this transformation and what challenges lie ahead to make the most out of this partnership.
Research Overview
Summary of Key Findings
A close look at current practices reveals several key findings regarding AI in healthcare:
- Enhanced Diagnostics: AI algorithms, particularly those that utilize deep learning, have shown remarkable promise in diagnosing conditions like diabetic retinopathy, skin cancers, and pneumonia more accurately than human eyes.
- Personalized Treatment Plans: Machine learning is not just working behind the scenes; it is actively tailoring treatment plans based on an individual's genetic makeup and unique health history, which, in turn, leads to more successful outcomes.
- Operational Efficiency: From streamlining patient records to predicting patient admissions, AI tools are making bureaucratic processes more manageable and freeing practitioners to focus more on patient care.
- Ethical Considerations: A delicate dance occurs between innovation and ethics. AI’s pervasive influence raises concerns around data privacy, biases, and the unpredictable consequences of machine decision-making in life-and-death scenarios.
Research Objectives and Hypotheses
The primary objective of this exploration is to craft a detailed narrative regarding the implications of AI within healthcare settings. To achieve this, several hypotheses are considered:
- Increased adoption of AI technologies will correlate with improved patient outcomes, reducing error rates in diagnostics.
- Personalization of treatment through AI will lead to increased patient satisfaction compared to traditional methods.
- The integration of AI in administrative processes will lead to reduced operational costs and allow healthcare professionals to focus more on patient care.
- Ethical considerations will stifle the full realization of AI’s potential due to legislative and societal pushback.
By framing these hypotheses, this article aims to illuminate not only the remarkable abilities of AI but also the complex world of ethics that surrounds its application in healthcare.
Prelude to Healthcare and Artificial Intelligence
The convergence of healthcare and artificial intelligence (AI) is a topic that carries immense significance in today’s world. As we turn the corner into an era where technology is inextricably linked to our very existence, the implications of AI in healthcare cannot be overstated. This relationship not only shapes the way medical professionals diagnose and treat patients but also influences the very policies that govern health systems globally. The seamless integration of AI can lead to improved patient outcomes, increased efficiency, and a transformation in the healthcare delivery model.
Notably, the role of AI extends beyond simple automation; its usage enhances decision-making, all while managing vast amounts of data in real time. Medical practitioners find themselves increasingly reliant on sophisticated algorithms that assist in everything from routine diagnostics to complex surgeries. The emphasis here is to recognize the multifaceted role of AI, which touches nearly every aspect of patient care and operational management.
"Artificial intelligence not only augments the capabilities of healthcare systems but also opens doors to revolutionary approaches in patient care."
Overview of AI in Modern Healthcare
In recent years, AI has made its mark across various facets of modern healthcare. From chatbots providing initial patient assessments to machine learning algorithms diagnosing diseases based on imaging data, the advancements are numerous. The integration of AI systems into electronic health records is another leap forward, facilitating prompt access to patient history while anticipating the potential risks associated with individualized treatments.
The eagerness in the industry to embrace AI stems from its ability to minimize errors commonly associated with human oversight. For instance, AI-powered systems have exhibited an exceptional capacity for analyzing medical images, often outperforming traditional diagnostic methods. This is of particular interest to radiologists who routinely interpret scans, as AI can flag anomalies with speed and accuracy that surpasses human capabilities.
Additionally, predictive analytics fueled by AI helps in population health management, where it becomes pivotal in understanding and preventing disease outbreaks. By studying data trends, healthcare providers can better allocate resources for patient care, and tailor interventions to match community needs. Thus, the potential benefits of AI are not only significant but transformative.
Historical Context of AI in Medicine
The journey of AI in medicine wasn’t built overnight. Its roots reach back to the 1950s, when the concept of machine learning was first introduced. Early efforts, however, were rudimentary at best, with limited computational power and even less data than is available today. The first AI systems were primarily focused on symbolic reasoning and problem-solving, not directly applicable to complex medical scenarios.
By the late 20th century, the landscape began to shift. Development of expert systems like MYCIN in the 1970s laid foundational work for computerized diagnostic tools. MYCIN was designed to diagnose bacterial infections; its systematic approach demonstrated the potential of AI in making medical decisions based on patient symptoms and data.
As technology continued to advance, so did our understanding of how to collect and analyze large datasets. The arrival of more sophisticated algorithms and enhanced computational abilities fueled the rise of AI applications in medicine, making strides in clinical trials and diagnostics.
Fast forward to today, and we have entered an age where AI isn't just an auxiliary tool but an indispensable part of healthcare strategies. The lessons learned from historical developments continue to inform current practices, providing a solid framework to navigate the evolving intersection of healthcare and AI.
In summary, the exploration of healthcare and AI is one that transcends mere novelty; it’s about creating a responsive, reliable, and forward-thinking medical environment. As we delve deeper into the various applications and implications, we can appreciate why this dialogue is vital for both today and the future.
Applications of AI in Healthcare
Artificial Intelligence (AI) is making waves in various domains, and healthcare is no exception. Its integration into medical practices has the potential to revolutionize patient care, streamline operations, and enhance diagnostic processes. This segment will delve into various applications of AI in healthcare, illustrating how they are shaping the future of medicine.
Diagnostic Imaging
Diagnostic imaging stands as one of the paramount applications of AI in healthcare. With the ability to analyze large amounts of visual data quickly and accurately, AI systems can assist radiologists in diagnosing conditions that might otherwise go unnoticed.
Machine Learning Algorithms in Radiology
Machine learning algorithms in radiology have gained traction due to their capacity for processing images to identify abnormalities. What makes these algorithms tick is their reliance on pattern recognition, which allows them to pinpoint issues such as tumors or fractures with unmatched precision. These machines can be trained using vast datasets of medical images, enabling them to learn from diverse visual anomalies. The key characteristic here is adaptability—these algorithms improve over time as they get exposed to more data. However, while they offer significant benefits, the human touch in interpreting results remains irreplaceable.
AI-assisted Pathology
Similarly, AI-assisted pathology is transforming how pathologists review tissue samples. These systems can analyze cellular patterns, vastly speeding up the identification of diseases like cancer. A standout aspect of AI-assisted pathology is its ability to manage and process a high volume of samples in a fraction of the time it would take a human. This choice is gaining popularity for its efficiency, but it also raises questions about the loss of human oversight in diagnosing complex conditions.
Predictive Analytics
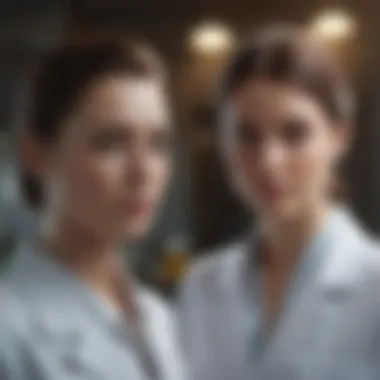
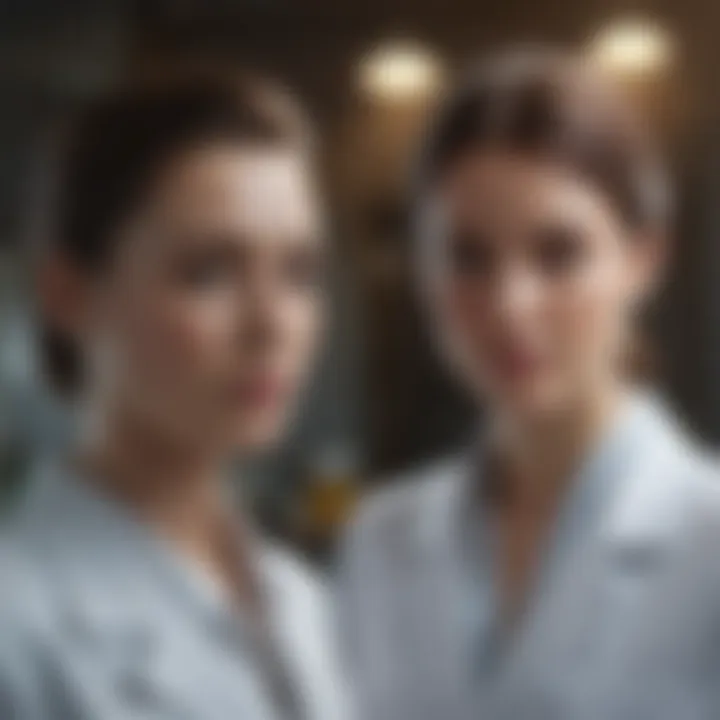
Predictive analytics harnesses AI to forecast outcomes based on historical data, an invaluable asset in proactive healthcare. These models are empowering doctors to take preemptive measures against diseases.
Risk Assessment Models
Risk assessment models utilize patient data—considering factors such as age, history, and genetics—to predict potential health risks. The crucial advantage of these models lies in their preventive nature, enabling healthcare providers to intervene before conditions escalate. They offer a promising route toward personalized care, though the unpredictability of human behavior poses challenges in achieving 100% accuracy.
Population Health Management
On a broader scale, AI plays a pivotal role in population health management. It enables healthcare systems to analyze health trends across various demographics, supporting targeted interventions. A notable feature of this approach is its community focus, helping in resource allocation and policy-making. While the benefits are clear, the reliance on big data can sometimes lead to privacy concerns, requiring stringent data protection measures.
Personalized Medicine
One of the most exciting frontiers of AI lies in personalized medicine, where treatment plans are tailored to individual patient needs.
Genomic Data Analysis
Genomic data analysis leverages AI to interpret genetic information, guiding treatment decisions. The key trait of this analysis is its precision, allowing healthcare providers to devise strategies based on a patient's unique genetic makeup. It’s not only a beneficial strategy for more effective treatments but also holds the potential of reducing adverse reactions to medications. Still, the complexity of human genetics makes this field challenging, and ethical considerations must be addressed.
Treatment Planning
Treatment planning, enhanced by AI, can streamline the process of developing individualized care strategies. By analyzing various treatment outcomes from a multitude of cases, AI algorithms can suggest methods that have historically yielded positive results for similar patients. However, despite its impressive potential, it can sometimes oversimplify complex medical scenarios, necessitating human oversight.
Robotic Surgery
AI's role in surgical procedures is another groundbreaking area, helping to improve outcomes and recovery times.
Automation in Surgical Procedures
Automation in surgical procedures deploys robots to assist during operations, enhancing control and minimizing human error. This aspect of AI has seen increased adoption due to its ability to execute precise movements even in high-pressure environments. Yet, the dependence on technology can raise concerns about reliability, especially in emergencies.
Enhanced Precision and Recovery
The precision that AI brings to surgery not only improves patient outcomes but often leads to quicker recoveries. With better accuracy, the risk of complications is significantly lowered, benefitting both patients and healthcare systems at large. However, over-reliance on robotic systems could also downplay the importance of medical professionals in the surgical field.
Telemedicine and AI
Finally, the advent of telemedicine, augmented by AI, is changing how healthcare is delivered, making it more accessible.
Virtual Health Assistants
Virtual health assistants are increasingly used to guide patients through healthcare processes, providing information and answering questions. The major benefit is accessibility—patients can access information from the comfort of their homes. However, one must be cautious; these systems can sometimes misinterpret complex medical conditions, prompting a need for expert consultation.
Remote Patient Monitoring
Remote patient monitoring is another AI application allowing healthcare providers to track patient data remotely, collecting vital signs and medication adherence in real-time. This system provides a unique advantage in managing chronic illnesses without requiring constant visits to the clinic. Nevertheless, reliability can be affected by device inaccuracies, highlighting the need for careful monitoring of the data.
To sum up, AI's applications in healthcare signify an era of innovation and improvement. From diagnostics to treatment, its role is multifunctional and deeply transformative. However, each application comes with its set of advantages and challenges that need careful consideration.
AI in Healthcare Research
The realm of AI in healthcare research is a rapidly evolving landscape that plays a critical role in the medical field's advancement. This segment focuses on how AI enhances clinical trials and drug development, with particular emphasis on the techniques employed and their real-world applications. In an era where data proliferates, the intersection of AI and healthcare research presents opportunities to streamline processes, improve outcomes, and push the boundaries of knowledge in medicine. The benefits reflect not only efficiency but also an elevation in the integrity of research findings. When AI is applied in these areas, it brings precision and speed that are hard to replicate with traditional methods.
Clinical Trials and Data Collection
Clinical trials have always been the cornerstone of medical research, but traditional trial methods can often be cumbersome and time-consuming. Here, AI sets the pace for transformation. By automating participant selection, researchers can make the trial process more efficient and less biased.
Automating Participant Selection
Automating participant selection revolutionizes how individuals are recruited for studies. This process utilizes algorithms that scan vast databases of patient records, identifying suitable candidates much faster than a human ever could. As a key part of the clinical trial landscape, it enhances the quality of trials by ensuring a representative population.
A hallmark of this method is its ability to reduce recruitment time significantly, leading to quicker trial outputs. As trials work on tight schedules to bring new treatments to market, this feature is invaluable. Not only does it save time, but it can also decrease costs associated with prolonged recruitment phases.
However, a notable disadvantage might arise from the reliance on algorithms, which could inadvertently overlook qualified participants due to predefined criteria. This presents a challenge in ensuring inclusivity, which could skew results.
Real-time Data Analysis
Real-time data analysis is another pivotal advance made possible through AI in clinical research. This technique involves not just collecting data but actively analyzing it throughout the trial, allowing researchers to shift gears quickly based on emerging results. In a situation where every moment counts, real-time insights enable a more adaptive approach to study protocols.
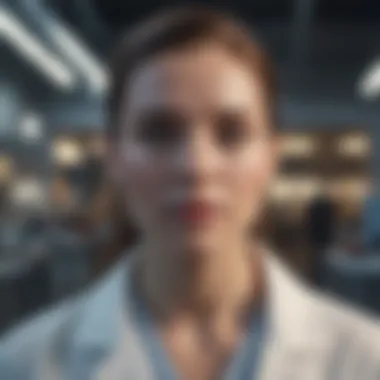
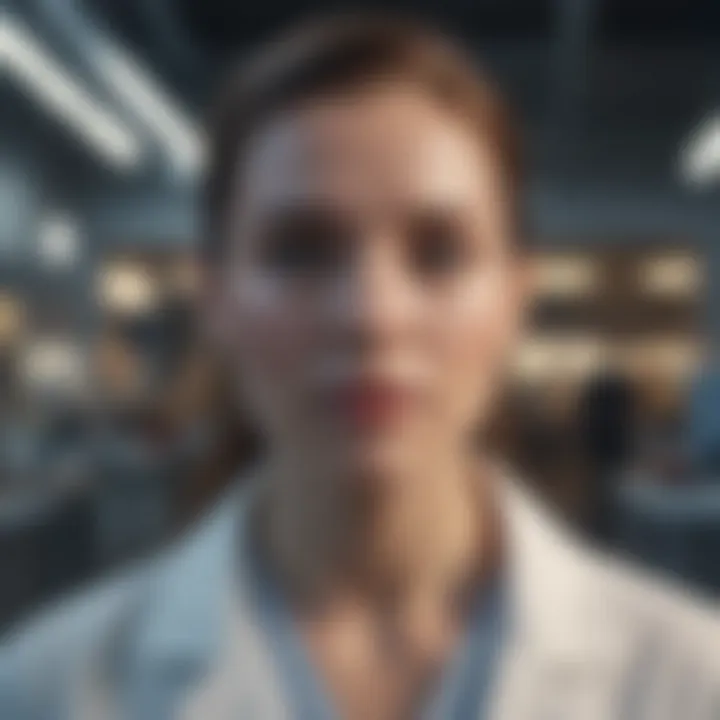
A key characteristic of this method is its capacity to enhance decision-making processes. Researchers can identify trends and anomalies almost instantaneously, shaping how a clinical trial unfolds. Meanwhile, the immediate feedback loop aids in detecting adverse effects sooner than with traditional methods, ultimately protecting participant safety.
However, the complexity of interpreting vast amounts of data in real-time may challenge researchers less familiar with data science principles, potentially leading to overreliance on automated systems.
Drug Discovery and Development
As the healthcare landscape continues to change, drug discovery and development stand out as fields ripe for technological innovation. AI is disrupting the norms here, offering methods that challenge conventional wisdom.
Modeling Biological Processes
Modeling biological processes allows researchers to simulate and predict how new drugs interact within the body. By employing complex algorithms and machine learning, scientists can analyze interactions at a molecular level without the need for extensive lab experimentation, thus accelerating discovery.
This method is appreciated for its predictive capabilities—it allows for more efficient exploration of potential therapies, saving time and reducing costs associated with trial-and-error approaches in labs. Despite its clarity, models sometimes rely on existing data, which may limit the breadth of predictions and occasionally overlook unquantifiable variables.
Identifying Potential Drug Targets
Identifying potential drug targets through AI helps researchers zoom in on specific areas of treatment, rather than casting wide nets as in the past. By using big data analytics, AI algorithms can pinpoint specific proteins or genes that could be effective targets for new medications.
This characteristic makes the process more focused, thus increasing the potential for successful outcomes while decreasing the number of failed drug trials. The targeted nature of this approach lends itself greatly to personalized medicine, paving the way for tailored therapies. However, this precision may come at a cost—it could lead to overlooking broader patterns that could also warrant investigation in a more traditional search.
With ongoing advancements in data-handling capabilities, the marriage of AI and healthcare research remains a hotbed for innovation. As these technologies continue to mature, they promise to not only enhance research methodologies but fundamentally reshape how healthcare systems operate at their core.
Ethical Considerations in AI Healthcare
The incorporation of artificial intelligence into healthcare raises significant ethical considerations that cannot be overlooked. These challenges touch on the very core of what healthcare stands for—trust, privacy, and equity. With advanced technologies making strides in diagnostics and treatment personalization, it’s paramount that we pause and reflect on ethical principles to safeguard patient interests. Furthermore, discussions around these pertinent issues pave the way for accountability and transparency within the AI systems that are increasingly becoming part of healthcare delivery.
Data Privacy and Security
In today's digital age, data is often referred to as the new oil, but, unlike oil, it's highly sensitive and personal. The use of AI in healthcare frequently requires access to vast amounts of patient data. This necessitates stringent measures to maintain the integrity and confidentiality of such information. If healthcare providers deploy algorithms that aggregate personal health data without proper safeguards, they risk exposing patients to breaches in privacy. Consider the ramifications of a security breach where sensitive health records are exposed—a nightmare scenario for both patients and providers alike.
Healthcare entities must implement robust encryption techniques and rigorous access controls to ensure that data remains safe from unauthorized access. They should establish clear protocols regarding data sharing and consent processes. The fact is, securing this sensitive information is not just about technology; it’s also about fostering a culture of privacy that emphasizes respect for the patient’s right to control their own data.
Bias and Discrimination
Algorithms and Healthcare Inequality
One of the darker aspects of AI in healthcare is the potential for algorithms to perpetuate bias and worsen healthcare disparities. Algorithms can lack the nuance necessary to understand the diverse backgrounds of patients, leading to unequal healthcare delivery. For instance, if a machine learning model is trained predominantly on data from one demographic, it can fail to accurately predict outcomes or recommend appropriate treatments for individuals from other racial or ethnic groups. This is not a mere oversight; it risks reinforcing existing healthcare inequalities.
The key characteristic that makes this issue especially concerning is that these biases can manifest in various forms in algorithms—whether it’s through misinterpretation of data or the omission of vital cultural considerations in treatment plans. Thus, it's becoming increasingly important for researchers and healthcare professionals to interrogate the training data used in AI models and to continually assess their performance across diverse populations.
Ensuring Fairness in AI Models
The challenge of ensuring fairness in AI models is equally vital. Transparent practices are crucial, allowing stakeholders to scrutinize the algorithms employed in healthcare. Systems must be designed to identify and mitigate bias at every stage—be it during data collection, model training, or outcome evaluation. The objective should be to develop AI systems that not only serve the majority but also give equitable attention to marginalized communities.
Ensuring fairness not only enhances the ethical integrity of technologies used in healthcare but also builds trust among patients. When they perceive that AI is applied in a way that respects their diversity and individuality, it fosters a cooperative relationship between users and the systems. To be fair, an AI model must be free from discriminatory practices that could ultimately harm vulnerable populations.
Informed Consent
Obtaining informed consent in an AI-driven healthcare landscape is worth careful consideration. Patients must be clearly informed about how their data will be used—especially if it's going to feed into an AI system that drives decision-making processes in their care.
Informed consent shouldn’t be a one-size-fits-all approach. An ethical framework for AI should incorporate a dialogue that allows patients to voice their concerns or preferences. Whether it's about diagnostic procedures or the potential use of their data for research purposes, they must feel equipped to make decisions regarding their healthcare journey. Transparency is vital here; patients should never feel like they’re stepping into a black box when it comes to understanding their treatment options. A well-informed patient is empowered, leading to better health outcomes and satisfaction.
"The ethical deployment of AI in healthcare is not just about technical solutions—it's about nurturing a culture of respect, understanding, and above all, trust."
Navigating the ethical dimensions of AI in healthcare requires serious commitment from all stakeholders. The balance between innovation and ethical responsibility is delicate, and ultimately, patient welfare should remain at the forefront. By acknowledging these ethical considerations, we can aim to create a healthcare environment that is not only efficient but also just and equitable.
Challenges and Limitations of AI in Healthcare
As promising as the integration of artificial intelligence into the healthcare arena may seem, it's essential to recognize that it doesn’t come without hurdles. Addressing these challenges and limitations is vital for ensuring that the technology meets the needs of patients and healthcare professionals alike.
The key areas where AI faces significant barriers include its integration with existing systems, the regulatory hurdles it must overcome, and the inherent dependence on data quality. Each of these elements plays a crucial role in determining how successfully AI will be applied in practical healthcare environments.
Integration with Existing Systems
To put it simply, AI must play well with the tools and infrastructure currently in use. Most healthcare environments rely on a patchwork of electronic health records, diagnostic tools, and administration systems. The challenge lies in marrying these existing systems with new AI technologies without creating chaos.
- Legacy Software: Many hospitals and healthcare facilities still operate with outdated software that might not support new AI applications, leading to potential integration hiccups.
- Interoperability Issues: Different systems often lack the ability to communicate seamlessly with one another, complicating the data exchange that AI depends on.
- Training Staff: Healthcare professionals might need training to smoothly work with AI systems, which can be time-consuming and resource-intensive.
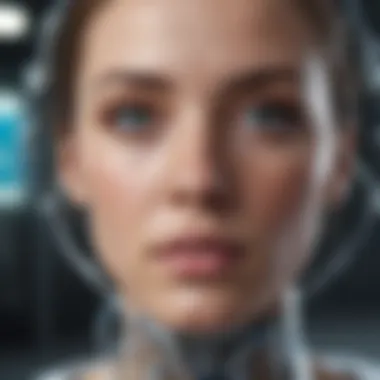
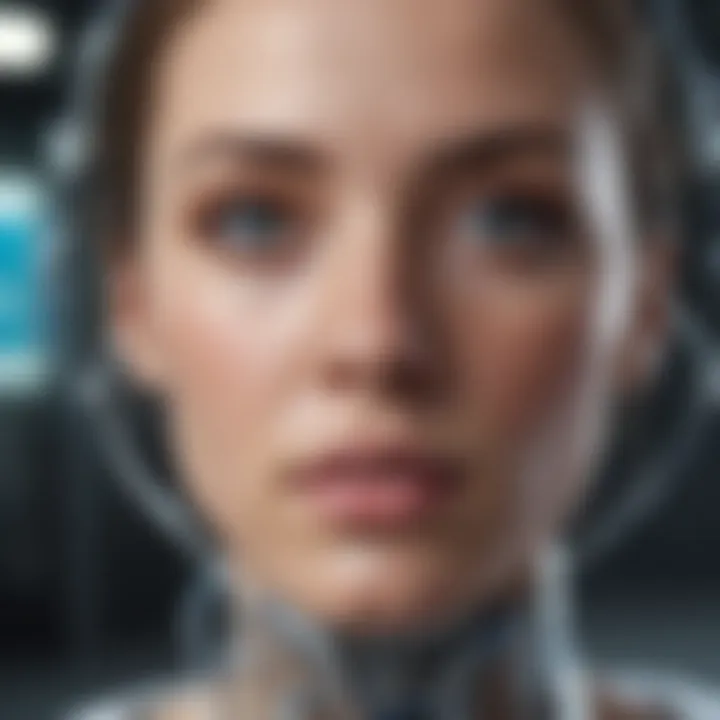
Regulatory Hurdles
Regulations inevitably shape the pathway for AI in healthcare. Regulatory bodies are tasked with ensuring patient safety, data privacy, and efficacy in medical treatments. Yet, navigating this bureaucratic landscape can be daunting for AI developers.
- Approval Processes: Gaining approval for AI applications often involves rigorous testing and scrutiny. This process can slow down innovation and access to advanced AI tools.
- Updating Regulations: As technology evolves very quickly, existing regulations may not keep up, leaving potential gaps in oversight.
- Liability Issues: Determining who is at fault when an AI system fails can be a thorny issue, complicating the landscape for both developers and healthcare institutions.
Dependence on Data Quality
Heard that old saying about garbage in, garbage out? Well, it holds true in the world of AI. High-quality, comprehensive data is the lifeblood of effective AI systems in healthcare. If the data is flawed or biased, the results will be, too.
- Data Sourcing: AI requires vast amounts of data for training, which means sourcing it from diverse, trustworthy repositories is crucial. Without this, the AI can learn inaccurate patterns.
- Bias in Data Sets: Often, datasets used for training AI models can reflect existing biases within the healthcare system, leading to disparities in care outcomes.
- Continuous Monitoring: Healthcare is dynamic, and to avoid outdated insights, there must be continuous monitoring and updating of data input processes.
AI in healthcare holds immense promise, yet maximizing its potential requires tackling these key challenges head-on. Each limitation must be acknowledged and addressed to secure patient safety and improve healthcare delivery.
Through understanding these complexities, stakeholders can better navigate the path towards a more innovative, efficient, and equitable healthcare system. By facing the obstacles of integration, regulation, and data quality, the future of AI in healthcare can transform from being a speculative vision to a robust reality.
The Future of AI in Healthcare
The realm of healthcare is on the brink of a profound metamorphosis, spearheaded by the advancements in artificial intelligence. As AI continues to weave itself into the fabric of medical practices, its potential to augment patient outcomes, streamline processes, and push the boundaries of research is becoming unmistakably clear. The future of AI in healthcare is not just about technology; it's about fostering a symbiotic relationship between human expertise and machine intelligence. In this section, we will navigate through the emerging trends, innovative practices, and the pivotal collaborations essential for shaping a future where healthcare is more accessible, efficient, and ethical.
Trends and Innovations
Continued Advancement in Machine Learning
The heartbeat of AI in healthcare lies within machine learning—an area that’s constantly gaining ground. This ongoing evolution is largely fueled by the sheer volume of data generated in the health sector, enabling algorithms to detect patterns that the human eye might miss. The capability of these machine learning systems to adapt and improve through experience allows them to provide predictive insights that enhance clinical decision-making.
A key characteristic of this advancement is the ability to process unstructured data. This means that machine learning algorithms can analyze various formats, like electronic health records or even social media posts about health experiences, which is a significant leap from traditional, structured data analysis. Such a feature not only increases the breadth of information available for insights but also boosts the efficacy of patient care protocols.
This trend is especially favorable because it entrusts healthcare providers with tools that can enhance diagnostic accuracy and treatment planning. However, one must also consider the data dependency—the algorithms are only as good as the quality and quantity of information fed into them, highlighting the importance of robust data governance practices.
Increased Use of Natural Language Processing
Delving into natural language processing (NLP), we encounter another transformative frontier in AI's healthcare journey. NLP has become an essential tool in interpreting vast amounts of textual data, facilitating clearer communication between healthcare professionals and patients while also streamlining administrative workflows. This technological stride enables systems to understand patient notes, correspondence, and research papers efficiently, thereby improving information retrieval.
A noteworthy characteristic of NLP is its ability to enhance patient interaction. Through applications like chatbots and virtual assistants, NLP can analyze patient inquiries in real-time, providing quick responses and referrals without human intervention. This not only alleviates the workload on healthcare personnel but also enhances patient satisfaction through timeliness and accuracy in information provided.
Although the advantages are clear, it's essential to be cautious of privacy concerns. The data harnessed by NLP systems must be handled with care to prevent breaches that could jeopardize patient confidentiality. Given the sensitive nature of health data, achieving balance here is crucial—as the technology continues to leap forward, we must ensure confidentiality doesn’t take a backseat.
Collaboration Between AI and Healthcare Professionals
To harness the advancements mentioned, collaboration is of paramount importance. This shared journey between AI developers and healthcare professionals is essential for the successful implementation of AI technologies. Such partnerships can cultivate an environment where feedback from frontline practitioners informs AI design, producing tools that are not only innovative but also practical and tailored for the healthcare landscape.
With clinicians, data scientists, and engineers working hand in hand, we pave the way for solutions that genuinely address the nuances of patient care, improve operational efficiency, and ultimately foster a more patient-centric approach to healthcare delivery.
Building an Ethical Framework
Beyond innovations and collaborations lies the pressing need for a robust ethical framework. As AI technologies proliferate, they must be underpinned by guidelines that prioritize patient welfare. Ethical considerations extend to data usage, bias mitigation, and transparency, ensuring that AI serves humanity rather than complicates it.
To prepare for the future effectively, we must engage in ongoing dialogues that encompass all stakeholders. Policymakers, tech developers, ethicists, and healthcare providers must convene to establish a shared set of values, fostering an ecosystem of trust and safety in the deployment of AI in healthcare.
The relationship between healthcare and AI holds immense promise, but it is clear that aiming for a future steeped in ethics and collective efforts is just as important as the technological advancements themselves.
The End
In summing up this exploration of the intricate relationship between artificial intelligence and healthcare, it's clear that AI has not merely nudged the door open; it’s kicked it down. The sheer volume of insights gained from the infusion of AI into healthcare processes stands as a testament to its transformative potential.
Recap of AI’s Impact on Healthcare
AI’s contributions to healthcare are manifold. From diagnostic advancements in imaging techniques to personalized treatment strategies based on genomics, AI is weaving itself into the very fabric of patient care. One of the most significant benefits lies in its ability to analyze vast datasets—unearthing patterns and trends that the human eye might miss. Take, for instance:
- Early detection of diseases through machine learning algorithms that assess radiology scans with precision.
- Tailoring treatments to individual patients by analyzing genetic information, ensuring that therapies are more effective and reduce unnecessary side effects.
- Streamlining administrative tasks, thus freeing up healthcare professionals to focus more on direct patient care.
Despite these advancements, it’s crucial to highlight that AI is not a panacea. The technology still struggles with data quality issues and potential biases, leading to disparities in healthcare access and outcomes. As AI systems continue to evolve, addressing these challenges should remain a priority for all stakeholders involved.
Final Thoughts on Interdisciplinary Collaboration
The future of healthcare lies in collaboration. Merging the expertise of healthcare professionals with the analytical prowess of AI specialists can lead to innovative solutions that enhance patient outcomes. It’s not just about technology pushing boundaries; it’s about people from varying disciplines coming together to craft a better healthcare experience.
By fostering an environment where interdisciplinary collaboration is encouraged, we can build an ethical framework that prioritizes patient welfare over profit. This underscores the importance of including diverse perspectives—be it from technology, ethics, medicine, or policy. In this way, we can influence the direction AI takes in healthcare, steering it toward a more equitable and effective future.
"AI should be a partner to healthcare professionals, not their replacement."
In closing, the intersection of AI and healthcare presents both opportunities and challenges. By remaining vigilant and proactive, we can harness this technology’s capability to improve healthcare while adhering to ethical standards.